LogTraceAD: Anomaly Detection from Both Logs and Traces with Graph Representation Learning.
CNCIT '23: Proceedings of the 2023 2nd International Conference on Networks, Communications and Information Technology(2023)
摘要
Abstract: The anomaly detection technique is increasingly applied in various security fields and the effectiveness and efficiency of anomaly detection models have become vitally important issues. Deep learning models are widely used to detect anomalies due to their flexibility and learning ability. However, in order to improve the performance of anomaly detection models, information used for model training and detecting is most significant. Previous methods involve the usage of system logs and traces, but mostly only focus on one single type of data source. And combining the logs and traces appropriately to retrieve comprehensive information for anomaly detection is still challenging. We propose LogTraceAD, a novel anomaly detection method that utilizes the logs and traces to generate a graph, and leverages a variational autoencoder-based graph representation learning model to complete feature learning. Then the feature data containing information from both types of data can be used for anomaly detection. We conduct the experiment on a publicly available dataset that contains 23,334 anomalies in 7,705,050 logs and 132,485 traces and compare the performance of the proposed method with several previous approaches. The result shows our method can achieve a 24% and 27% improvement respectively compared to methods using only logs or traces, and will not cause high overhead.
更多查看译文
AI 理解论文
溯源树
样例
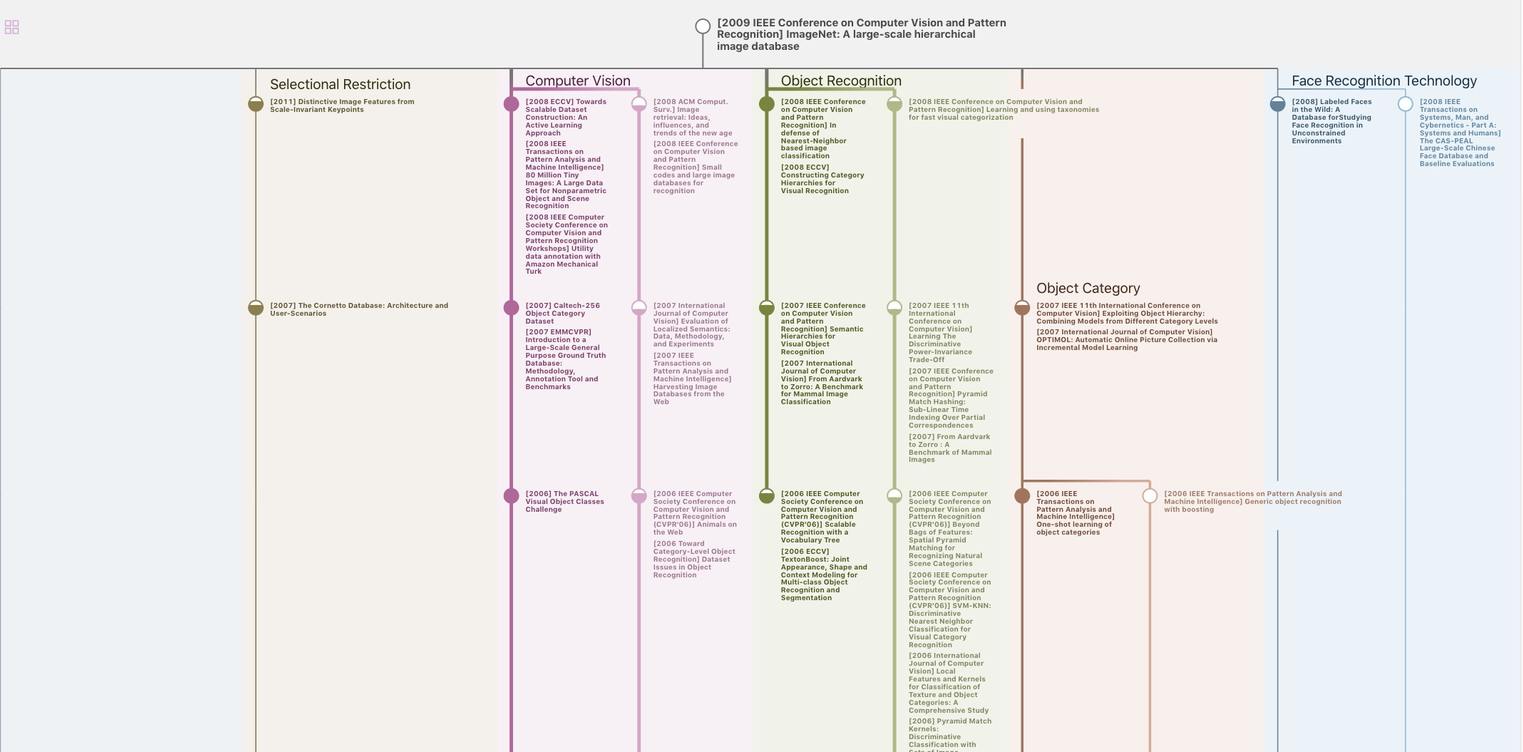
生成溯源树,研究论文发展脉络
Chat Paper
正在生成论文摘要