Attention-based Multi-scale ViT Fine-grained Visual Classification.
International Conference on Computer Science and Artificial Intelligence(2022)
摘要
Fine-grained visual classification (FGVC) is a challenging task in image classification due to the small differences between classes and the large differences within subclasses. In the early works, some methods mainly rely on constructing bounding box annotations and integrating attention mechanisms based on CNN methods for fine-grained visual classification. In recent years, the Vision Transformer (ViT) has begun to show better performance in image classification, object detection, and object tracking. To further investigate the performance of ViT in FGVC, this paper proposes to combine the CNN method with ViT and introduce a dual-path hierarchy into the pyramid structure - top-down feature path and bottom-up channel-spatial attention path; DropBlock is used to accurately localize discriminative regions; SENet and global covariance pooling (GCP) measures are used to further enhance the ability of the network model to extract feature maps information. The Attention-based Multi-scale ViT Fine-grained Visual Classification (AMViT-CNN) proposed in this work has achieved good classification results on public fine-grained datasets (CUB-200-2011, Stanford-Cars).
更多查看译文
AI 理解论文
溯源树
样例
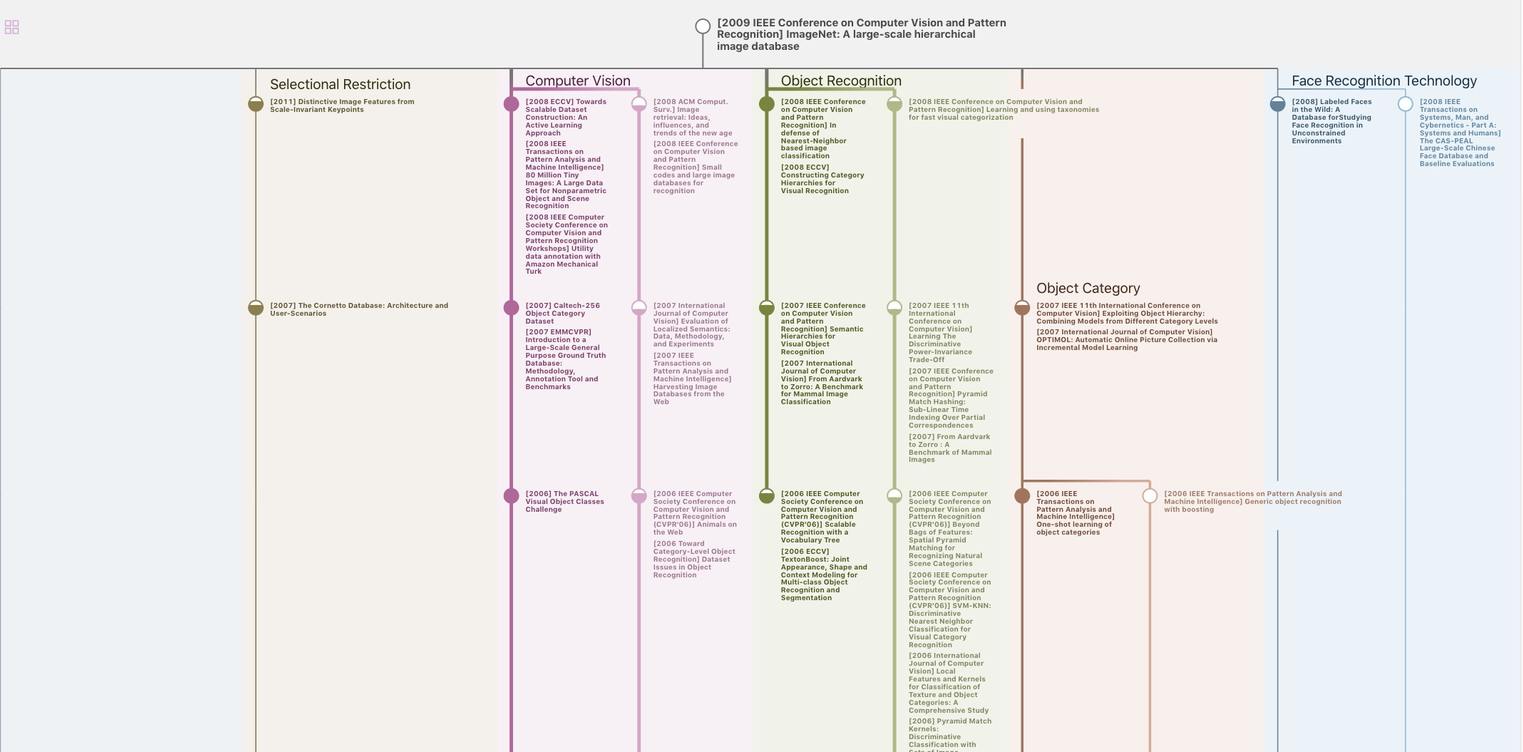
生成溯源树,研究论文发展脉络
Chat Paper
正在生成论文摘要