Vast TVB parameter space exploration: A Modular Framework for Accelerating the Multi-Scale Simulation of Human Brain Dynamics.
CoRR(2023)
摘要
Global neural dynamics emerge from multi-scale brain structures, with neurons
communicating through synapses to form transiently communicating networks.
Network activity arises from intercellular communication that depends on the
structure of connectome tracts and local connection, intracellular signalling
cascades, and the extracellular molecular milieu that regulate cellular
properties. Multi-scale models of brain function have begun to directly link
the emergence of global brain dynamics in conscious and unconscious brain
states to microscopic changes at the level of cells. In particular, AdEx
mean-field models representing statistical properties of local populations of
neurons have been connected following human tractography data to represent
multi-scale neural phenomena in simulations using The Virtual Brain (TVB).
While mean-field models can be run on personal computers for short simulations,
or in parallel on high-performance computing (HPC) architectures for longer
simulations and parameter scans, the computational burden remains high and vast
areas of the parameter space remain unexplored. In this work, we report that
our TVB-HPC framework, a modular set of methods used here to implement the
TVB-AdEx model for GPU and analyze emergent dynamics, notably accelerates
simulations and substantially reduces computational resource requirements. The
framework preserves the stability and robustness of the TVB-AdEx model, thus
facilitating finer resolution exploration of vast parameter spaces as well as
longer simulations previously near impossible to perform. Given that simulation
and analysis toolkits are made public as open-source packages, our framework
serves as a template onto which other models can be easily scripted and
personalized datasets can be used for studies of inter-individual variability
of parameters related to functional brain dynamics.
更多查看译文
AI 理解论文
溯源树
样例
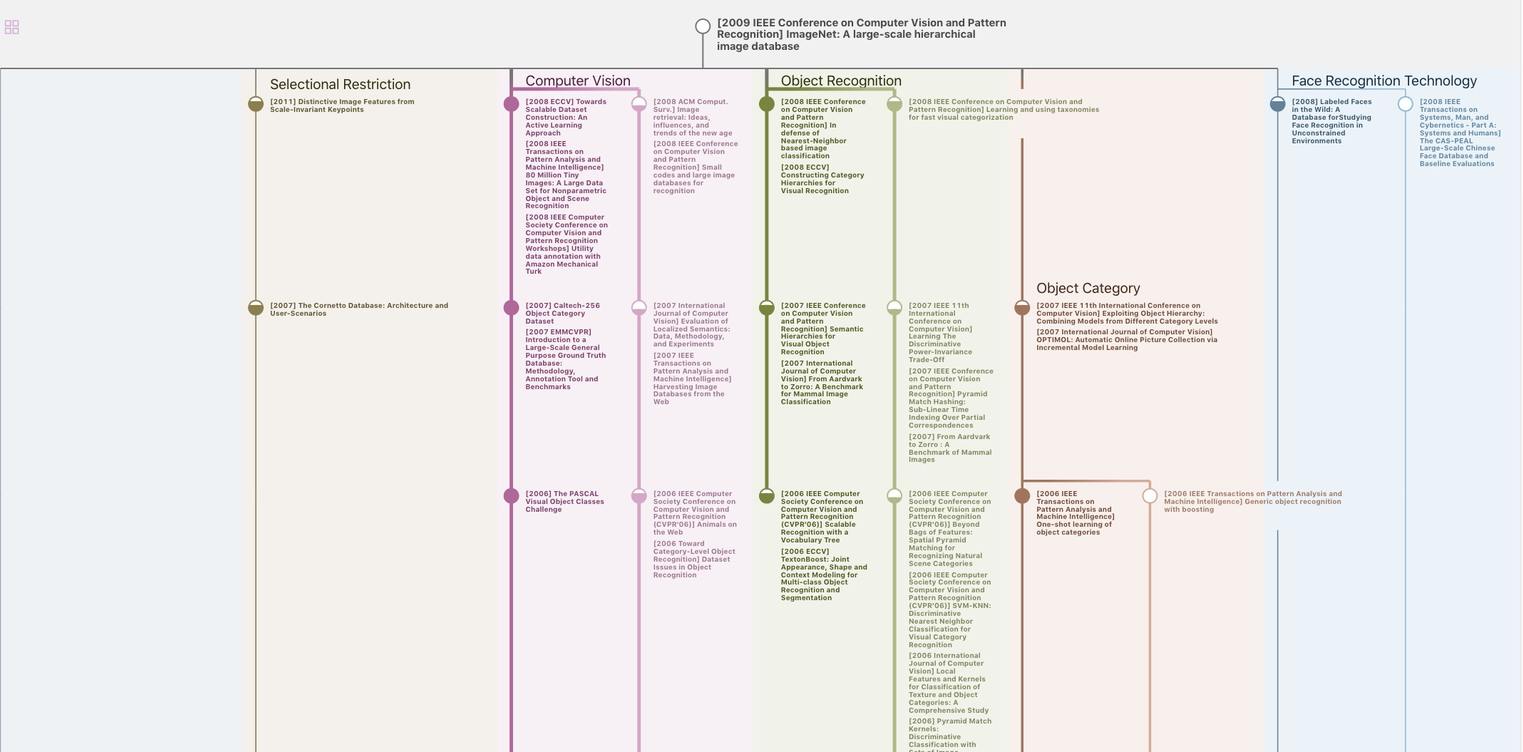
生成溯源树,研究论文发展脉络
Chat Paper
正在生成论文摘要