Volumetric 3D Point Cloud Attribute Compression: Learned polynomial bilateral filter for prediction
ICASSP 2024 - 2024 IEEE International Conference on Acoustics, Speech and Signal Processing (ICASSP)(2023)
摘要
We extend a previous study on 3D point cloud attribute compression scheme
that uses a volumetric approach: given a target volumetric attribute function
$f : \mathbb{R}^3 \mapsto \mathbb{R}$, we quantize and encode parameters
$\theta$ that characterize $f$ at the encoder, for reconstruction
$f_{\hat{\theta}}(\mathbf(x))$ at known 3D points $\mathbf(x)$ at the decoder.
Specifically, parameters $\theta$ are quantized coefficients of B-spline basis
vectors $\mathbf{\Phi}_l$ (for order $p \geq 2$) that span the function space
$\mathcal{F}_l^{(p)}$ at a particular resolution $l$, which are coded from
coarse to fine resolutions for scalability. In this work, we focus on the
prediction of finer-grained coefficients given coarser-grained ones by learning
parameters of a polynomial bilateral filter (PBF) from data. PBF is a
pseudo-linear filter that is signal-dependent with a graph spectral
interpretation common in the graph signal processing (GSP) field. We
demonstrate PBF's predictive performance over a linear predictor inspired by
MPEG standardization over a wide range of point cloud datasets.
更多查看译文
关键词
3D point cloud compression,deep learning
AI 理解论文
溯源树
样例
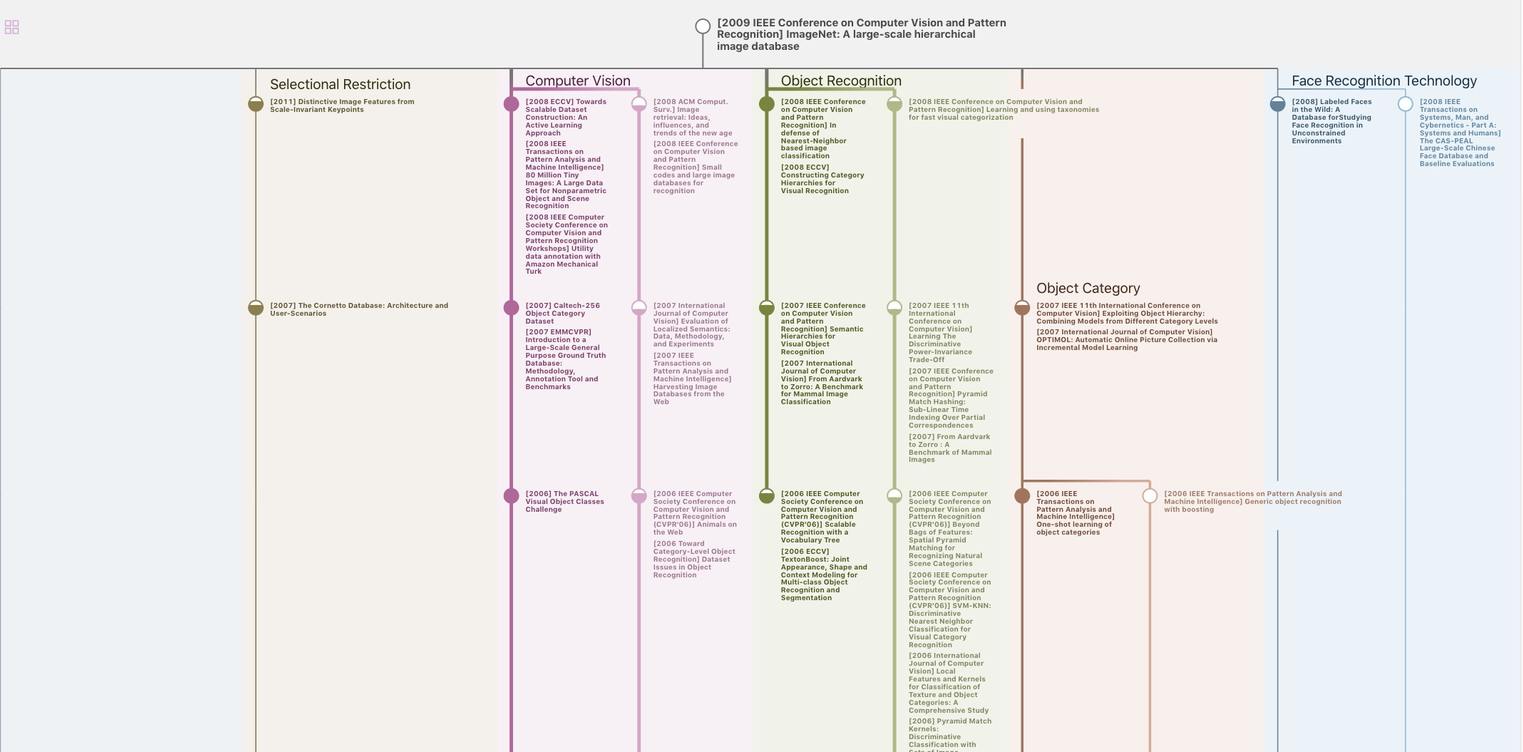
生成溯源树,研究论文发展脉络
Chat Paper
正在生成论文摘要