Self-ChakmaNet: A deep learning framework for indigenous language learning using handwritten characters
EGYPTIAN INFORMATICS JOURNAL(2023)
摘要
According to UNESCO's Atlas of the World's Languages in Danger, 40% of the languages today are counted as endangered in the future. Indigenous languages are endangered because of the less availability of interactive learning mediums for those languages. Thus this paper proposes an interactive deep learning method for Handwritten Character Recognition of the indigenous language "Chakma." The method comprises dataset creation using a mobile app named "EthnicData." It reports the first "Handwriting Character Dataset" of Chakma containing 47,000 images of 47 characters of Chakma language using the app. A novel SelfONN-based deep learning model, Self-ChakmaNet, is proposed in this research for Chakma Handwritten character recognition. The Self-ChakmaNet achieved 99.84% for overall accuracy, precision, recall, F1 score, and sensitivity. The proposed model with high accuracy can be implemented in mobile devices for handwritten character recognition as the model has less number of parameters and a faster processing speed.
更多查看译文
关键词
Chakma language,Handwritten character recognition,Deep learning,Self-ONN
AI 理解论文
溯源树
样例
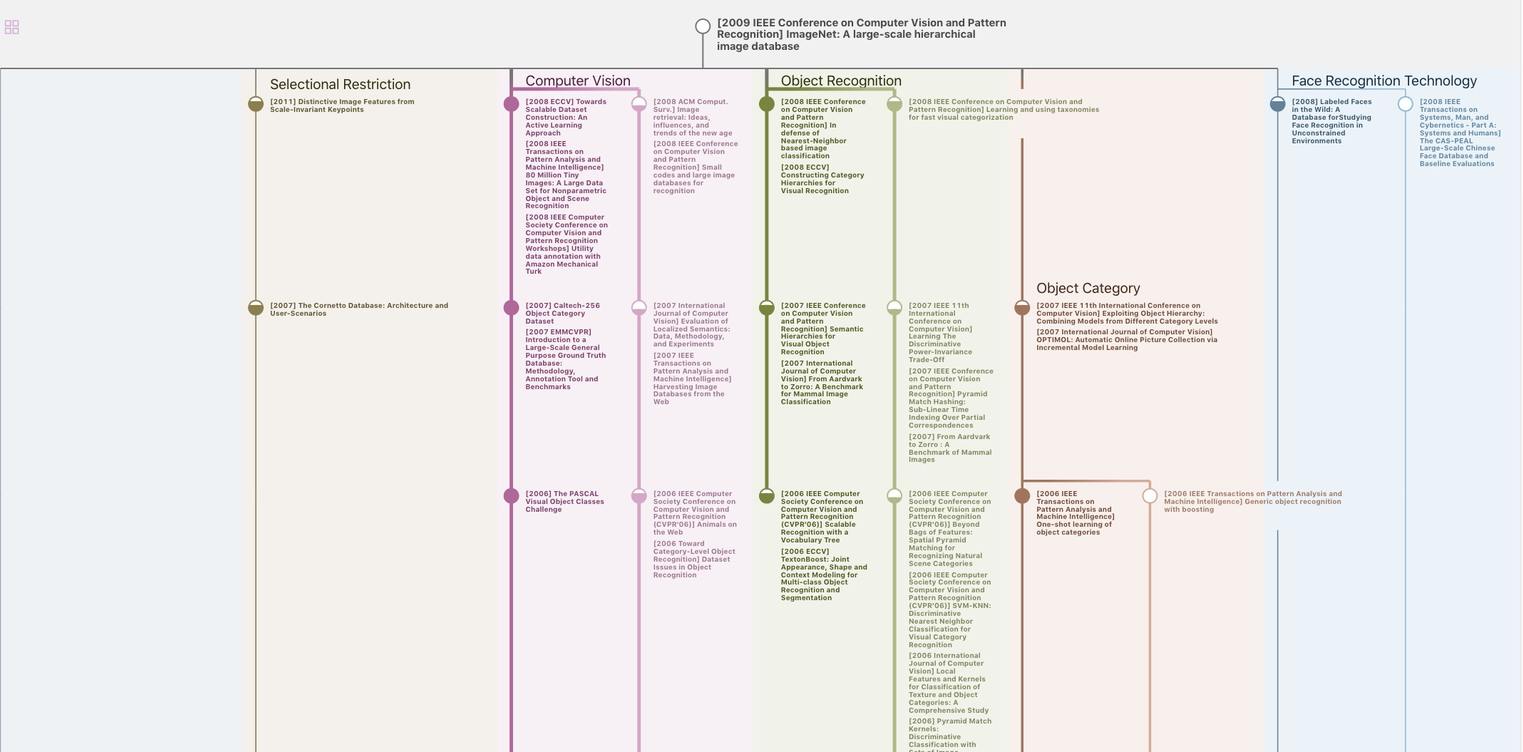
生成溯源树,研究论文发展脉络
Chat Paper
正在生成论文摘要