TRAPDOOR: Repurposing neural network backdoors to detect dataset bias in machine learning-based genomic analysis
2023 IFIP/IEEE 31ST INTERNATIONAL CONFERENCE ON VERY LARGE SCALE INTEGRATION, VLSI-SOC(2023)
摘要
Use of Machine Learning (ML) to understand underlying patterns in gene mutations (genomics) has far-reaching results in diagnosis and treatment for life-threatening diseases like cancer. Success and sustainability of ML algorithms depends on the quality and diversity of training data, and under-representation of groups (gender, race, etc.) can lead to exacerbation of systemic discrimination issues. In this work, we propose TRAPDOOR, a methodology for the identification of biased datasets by repurposing, otherwise malicious, neural backdoors. Our methodology can leak potential bias information about the cloud's dataset which is collected in a collaborative setting, without hampering the genuine performance. Using a real-world cancer genomics dataset, we analyze feasibility of leaking bias for gender and race attributes. Our experimental results show that TRAPDOOR can detect the presence of dataset bias with 100% accuracy, and furthermore can also extract the extent of bias by recovering the percentage with a small error.
更多查看译文
AI 理解论文
溯源树
样例
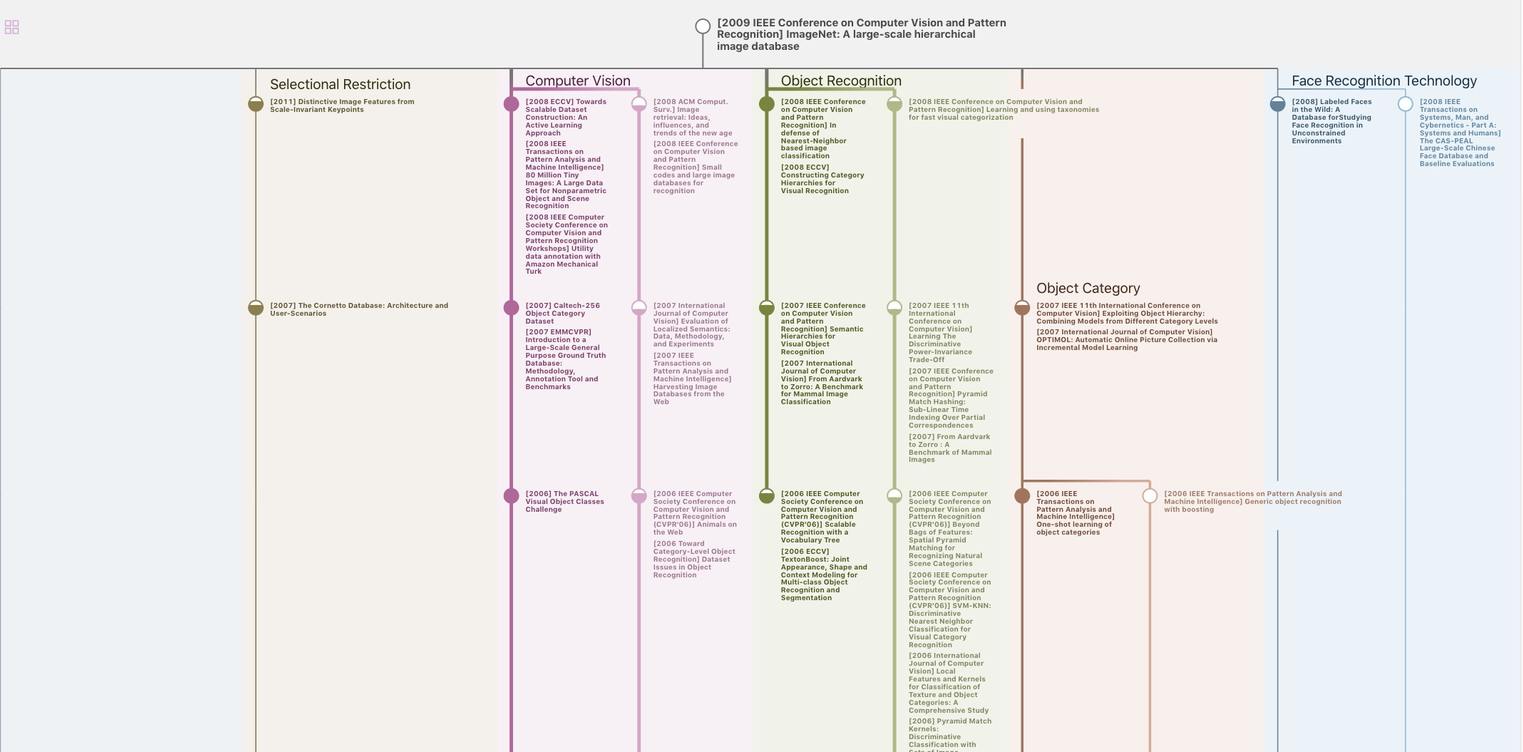
生成溯源树,研究论文发展脉络
Chat Paper
正在生成论文摘要