Efficient Memory Encoding Explains the Interactions Between Hippocampus Size, Individual Experience, and Clinical Outcomes: A Computational Model
biorxiv(2023)
摘要
The relationship between hippocampal volume and memory function has produced mixed results in neuroscience research. However, an experience-dependent efficient encoding mechanism underlies these varied observations. We present a model that utilizes an autoencoder to prioritize sparseness and transforms the recurrent loop between the cortex and hippocampus into a deep neural network. We trained our model with the Fashion MNIST database and a loss function to modify synapses via backpropagation of mean squared recall error. The model exhibited experience-dependent efficient encoding, representing frequently repeated objects with fewer neurons and smaller loss penalties and similar representations for objects repeated equally. Our findings clarify perplexing results from neurodevelopmental studies: linking increased hippocampus size and memory impairments in ASD to decreased sparseness, and explaining dementia symptoms of forgetting with varied neuronal integrity. Our findings propose a novel model that connects observed relationships between hippocampus size and memory, contributing to the development of a larger theory on experience-dependent encoding and storage and its failure.
### Competing Interest Statement
The authors have declared no competing interest.
更多查看译文
AI 理解论文
溯源树
样例
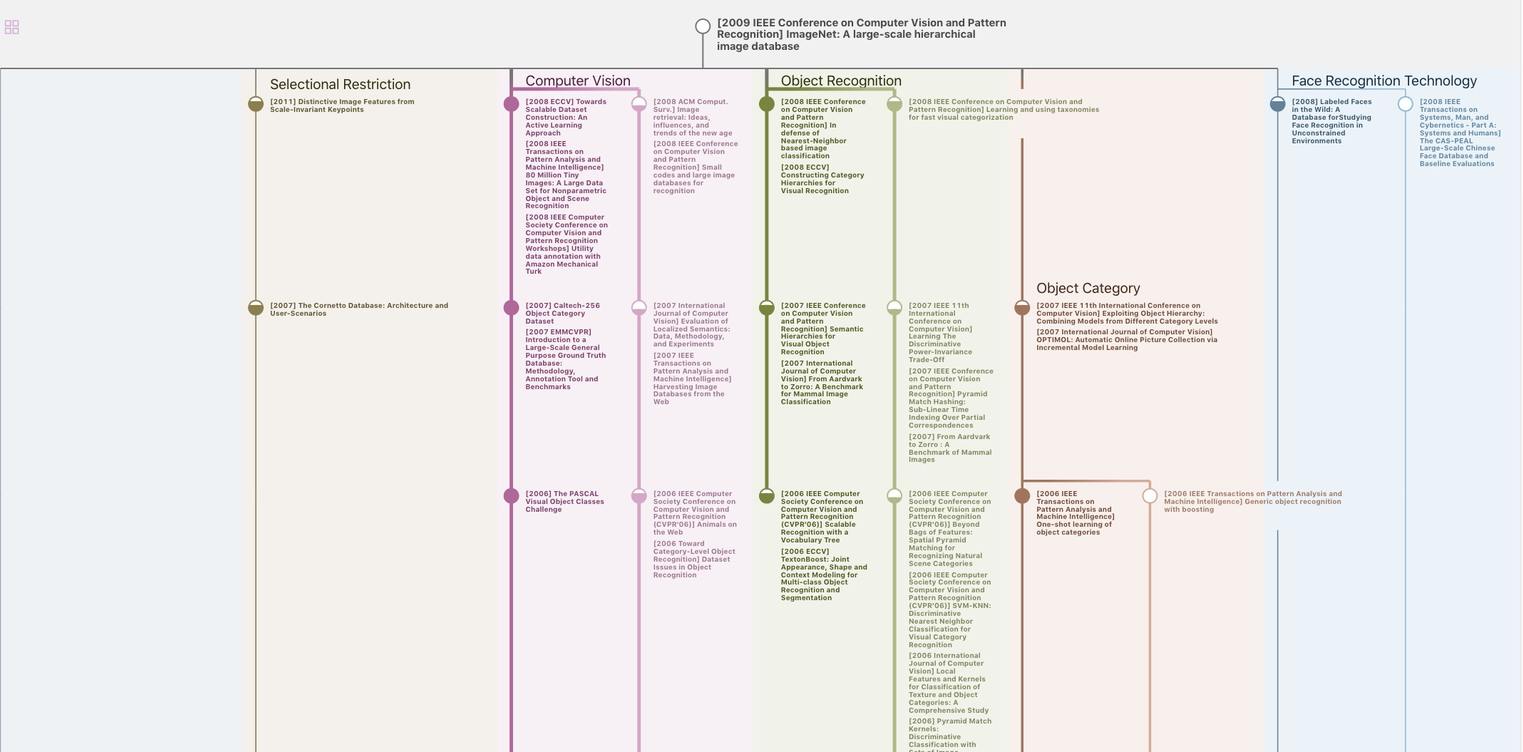
生成溯源树,研究论文发展脉络
Chat Paper
正在生成论文摘要