A Pareto-Optimal Privacy-Accuracy Settlement For Differentially Private Image Classification
2023 5th International Conference on Pattern Analysis and Intelligent Systems (PAIS)(2023)
摘要
Effectively training differentially private models in machine learning requires optimizing the hyper-parameters while ensuring privacy and maintaining accuracy. This research addresses this challenge by analyzing hyper-parameter tuning results and employs the Pareto frontier approach to identify optimal trade-offs and architectures for private learning. The findings enhance understanding of privacy considerations and inform the development of effective training methodologies and the decision-making process for practical applications.
更多查看译文
关键词
Privacy preserving deep learning,Differential privacy,privacy-utility trade-off,Pareto Frontier
AI 理解论文
溯源树
样例
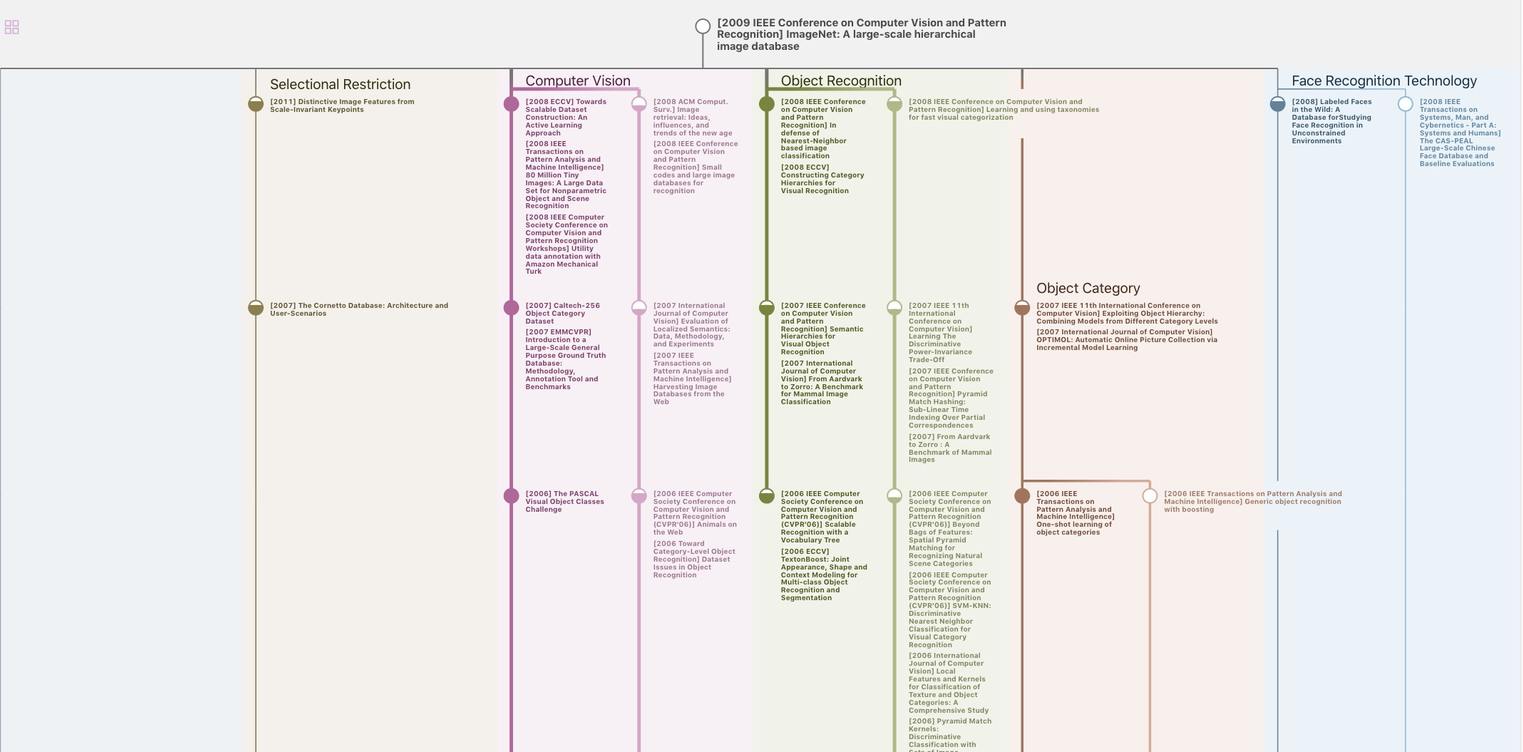
生成溯源树,研究论文发展脉络
Chat Paper
正在生成论文摘要