Geodemographic Profiling of Malicious IP addresses
2023 20TH ANNUAL INTERNATIONAL CONFERENCE ON PRIVACY, SECURITY AND TRUST, PST(2023)
摘要
Predicting and tracking cyber-attacks is the first step towards preventing them. Cyber-attacks have been found to have connections with socio-economical and political characteristics. In this paper, we investigate whether the social, demographic, economic, health, and wellness characteristics of a geographical region affect the selection of IP addresses originating from it for initiating attacks. We call the task the geodemographic profiling of malicious IP addresses. Geodemographic profiling of malicious IP addresses is essential for predicting and tracking cyber-attacks as it helps direct and prioritize efforts to defend against attacks from certain types of profiles. To address the problem, we collect data that include IP black-lists, IP geo-location, and socio-economical/demographics/health and wellness (SDH) characteristics of geographical regions in the US. We model the research problem as a classification problem. Thus, we train supervised machine learning models on the collected data with a wide range of SDH features. By testing the trained models, we predict if an area is highly likely to be the source of malicious IP addresses. We also investigate the effect of different labeling methods, outlier analysis, class balancing, and feature selection approaches. We obtain the best prediction performance with 99.26% accuracy and AUC (Area Under the ROC curve) when the SVM classifier is trained on over-sampled datasets with features selected using the Random Forest embedded feature selector. The most relevant features in the prediction process include the percentage of people with overweight, people walking or bicycling, place of birth, and presidential election results.
更多查看译文
关键词
malicious IP profiling,malware,geo-location,geodemographic,socio-economical,political,health and wellness,machine learning
AI 理解论文
溯源树
样例
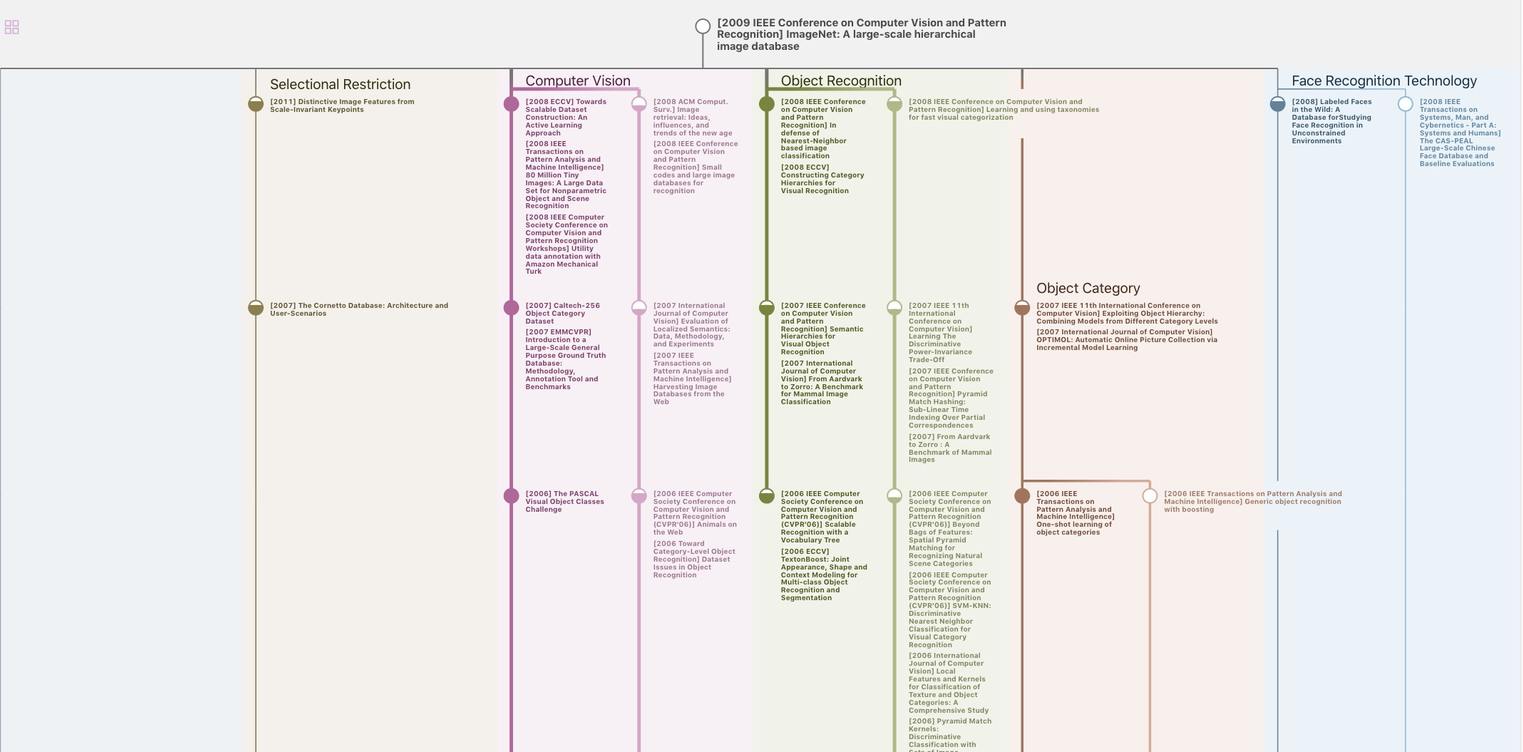
生成溯源树,研究论文发展脉络
Chat Paper
正在生成论文摘要