Two Birds with One Stone: A Link Prediction Model for Knowledge Hypergraph Based on Fully-Connected Tensor Decomposition.
Advanced Data Mining and Applications: 19th International Conference, ADMA 2023, Shenyang, China, August 21–23, 2023, Proceedings, Part II(2023)
摘要
Knowledge hypergraph link prediction aims to predict missing relationships in knowledge hypergraphs and is one of the effective methods for graph completion. The existing optimal knowledge hypergraph link method based on tensor decomposition, i.e., GETD (Generalized Model based on Tucker Decomposition and Tensor Ring Decomposition), has achieved good performance by extending Tucker decomposition, but there are still two main problems: (1)GETD does not establish operations or connections between any two tensor factors, resulting in limited representation of tensor correlation (referred to as finiteness); (2)The tensor decomposed by GETD is highly sensitive to the arrangement of tensor patterns (referred to as sensitivity). In response to the above issues, we propose a knowledge hypergraph link prediction model, called GETD + , based on fully-connected tensor decomposition(FCTN). By combining Tucker decomposition and FCTN, a multi-linear operation/connection is established for any two factor tensors obtained from tensor decomposition. This not only enhances the representation ability of tensors, but also eliminates sensitivity to tensor pattern arrangement. Finally, the superiority of the GETD + model was verified through a large number of experiments on real knowledge hypergraph datasets and knowledge graph datasets.
更多查看译文
关键词
knowledge hypergraph,link prediction model,fully-connected
AI 理解论文
溯源树
样例
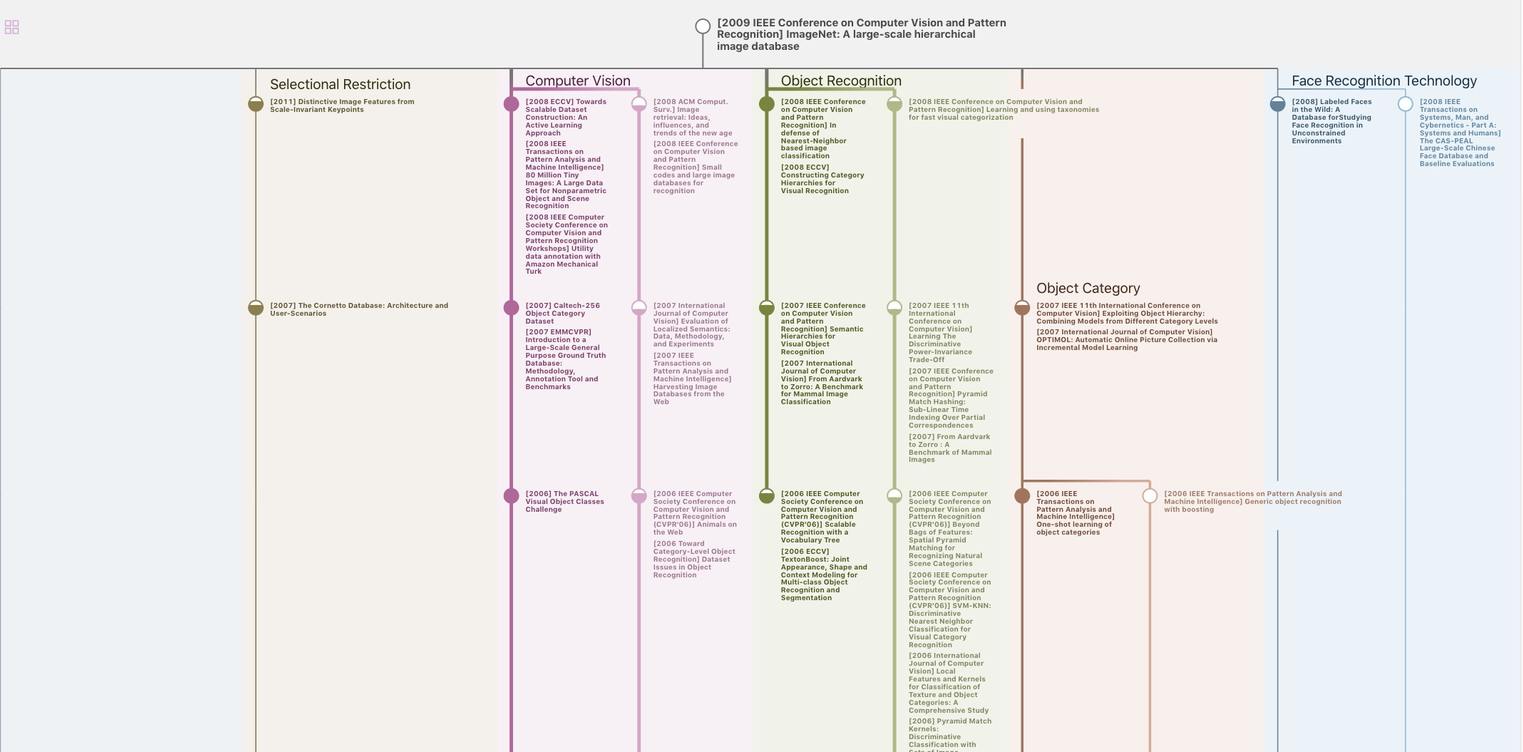
生成溯源树,研究论文发展脉络
Chat Paper
正在生成论文摘要