Can Existing 3D Monocular Object Detection Methods Work in Roadside Contexts? A Reproducibility Study.
AIxIA 2023 – Advances in Artificial Intelligence: XXIInd International Conference of the Italian Association for Artificial Intelligence, AIxIA 2023, Rome, Italy, November 6–9, 2023, Proceedings(2023)
摘要
Detecting 3D objects in images from urban monocular cameras is essential to enable intelligent monitoring applications for local municipalities decision-support systems. However, existing detection methods in this domain are mainly focused on autonomous driving and limited to frontal views from sensors mounted on the vehicle. In contrast, to monitor urban areas, local municipalities rely on streams collected from fixed cameras, especially in intersections and particularly dangerous areas. Such streams represent a rich source of data for applications focused on traffic patterns, road conditions, and potential hazards. In this paper, given the lack of availability of large-scale datasets of images from roadside cameras, and the time-consuming process of generating real labelled data, we first proposed a synthetic dataset using the CARLA simulator, which makes dataset creation efficient yet acceptable. The dataset consists of 7,481 development images and 7,518 test images. Then, we reproduced state-of-the-art models for monocular 3D object detection proven to work well in autonomous driving (e.g., M3DRPN, Monodle, SMOKE, and Kinematic) and tested them on the newly generated dataset. Our results show that our dataset can serve as a reference for future experiments and that state-of-the-art models from the autonomous driving domain do not always generalize well to monocular roadside camera images. Source code and data are available at https://bit.ly/monocular-3d-odt .
更多查看译文
关键词
roadside contexts,object detection,3d
AI 理解论文
溯源树
样例
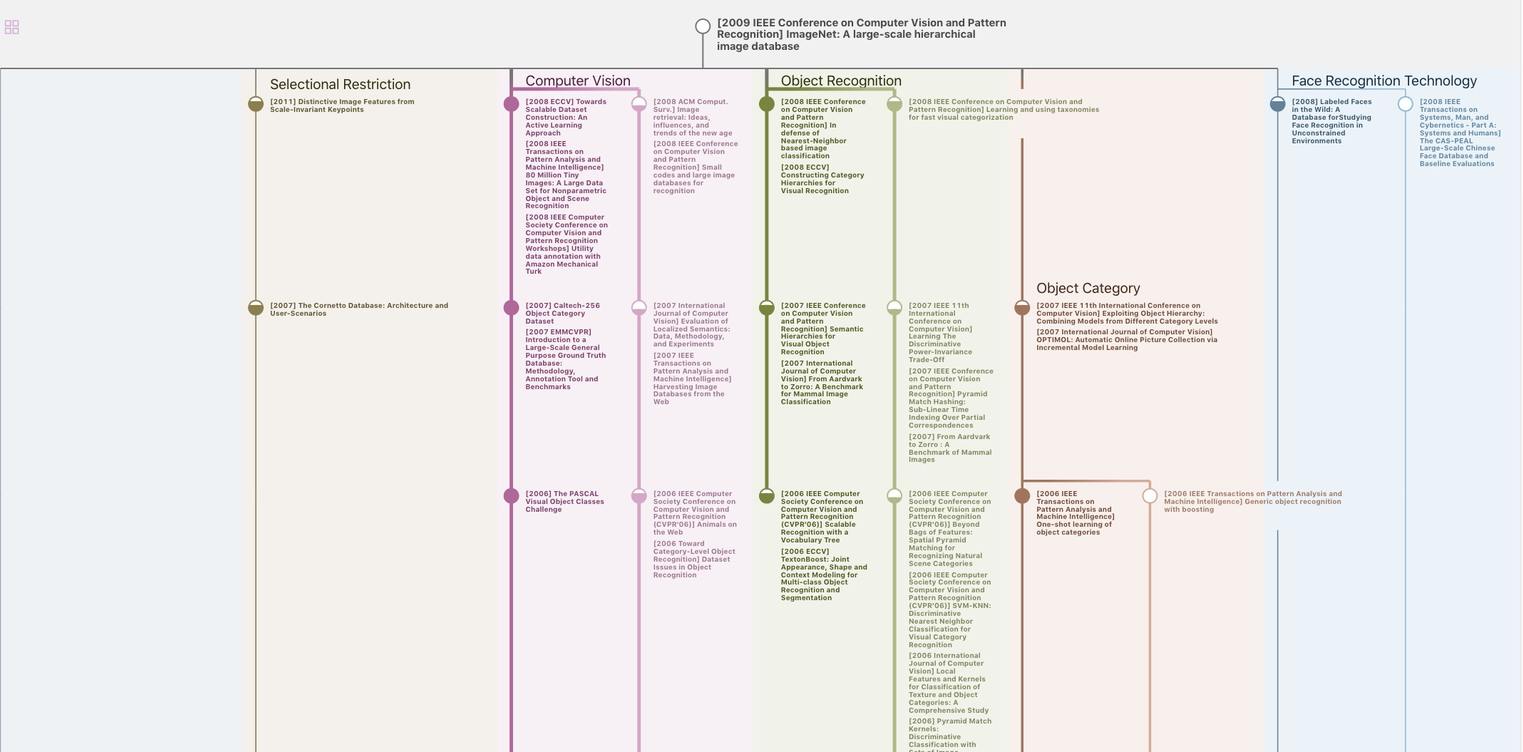
生成溯源树,研究论文发展脉络
Chat Paper
正在生成论文摘要