Clinical Trial Histology Image Based End-to-End Biomarker Expression Levels Prediction and Visualization Using Constrained GANs
APPLICATIONS OF MEDICAL ARTIFICIAL INTELLIGENCE, AMAI 2023(2024)
摘要
The gold standard for diagnosing cancer is through pathological examination. This typically involves the utilization of staining techniques such as hematoxylin-eosin (H&E) and immunohistochemistry (IHC) as relying solely on H&E can sometimes result in inaccurate cancer diagnoses. IHC examination offers additional evidence to support the diagnostic process. Given challenging accessibility issues of IHC examination, generating virtual IHC images from H&E-stained images presents a viable solution. This study proposes Active Medical Segmentation and Rendering (AMSR), an end-to-end framework for biomarker expression levels prediction and virtual staining, leveraging constrained Generative Adversarial Networks (GAN). The proposed framework mimics the staining processes, surpassing prior works and offering a feasible substitute for traditional histopathology methods. Preliminary results are presented using a clinical trial dataset pertaining to the CEACAM5 biomarker.
更多查看译文
关键词
Histological staining,Biomarker expression,GAN Models
AI 理解论文
溯源树
样例
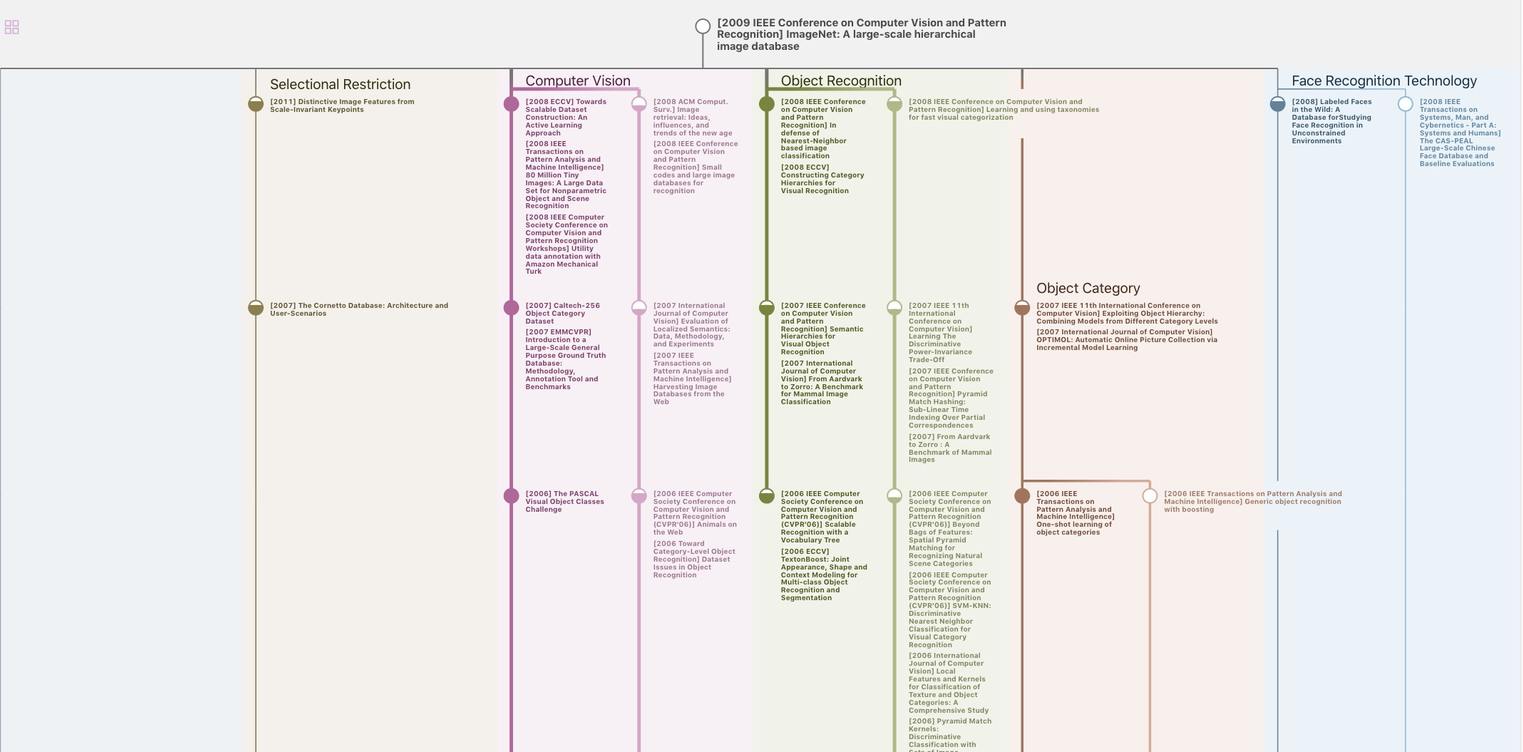
生成溯源树,研究论文发展脉络
Chat Paper
正在生成论文摘要