Exploring Optimal Configurations in Active Learning for Medical Imaging
ARTIFICIAL INTELLIGENCE XL, AI 2023(2023)
摘要
Medical imaging is a critical component of clinical decision-making, patient diagnosis, treatment planning, intervention, and therapy. However, due to the shortage of qualified radiologists, there is an increasing burden on healthcare practitioners, which underscores the need to develop reliable automated methods. Despite the development of novel computational techniques, interpreting medical images remains challenging due to noise and varying acquisition conditions. One promising solution to improve the reliability and accuracy of automated medical image analysis is Interactive Machine Learning (IML), which integrates human expertise into the model training process. Active learning (AL) is an important IML technique that can iteratively query for informative samples to be labeled by humans, leading to more data-efficient learning. To fully leverage the potential of active learning, however, it is crucial to understand the optimal setup for different components of an AL system. This paper presents an evaluation of the effectiveness of different combinations of data representation, model capacity, and query strategy for active learning systems designed for medical image classification tasks. The results of this evaluation show that employing raw image representations as input, in conjunction with a ResNet50 model and margin-based queries, yields more reliable and accurate automated methods for medical image analysis.
更多查看译文
关键词
Human-in-the-loop (HITL),Interactive Machine Learning (IML),Active Learning (AL)
AI 理解论文
溯源树
样例
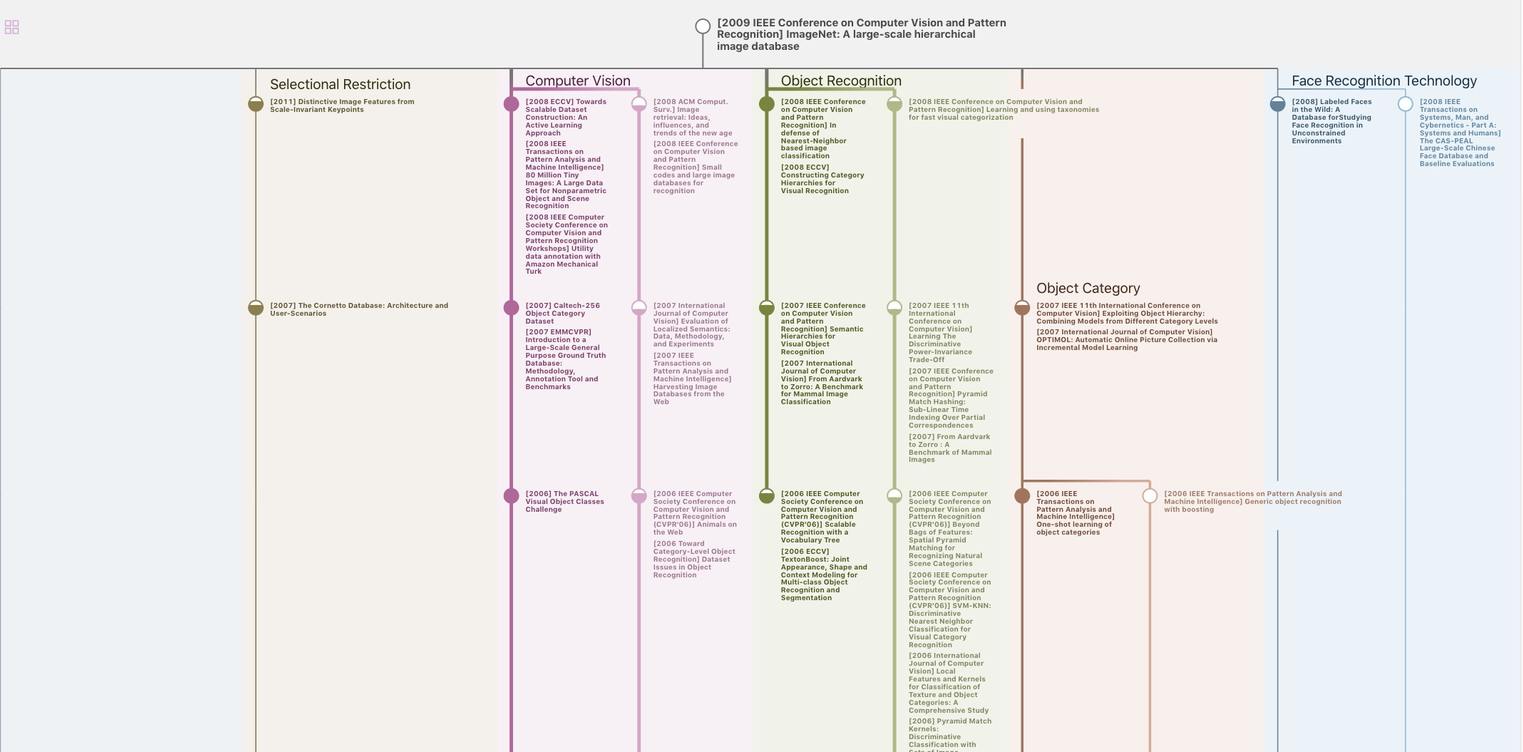
生成溯源树,研究论文发展脉络
Chat Paper
正在生成论文摘要