MAGNet: Muti-scale Attention and Evolutionary Graph Structure for Long Sequence Time-Series Forecasting
ARTIFICIAL NEURAL NETWORKS AND MACHINE LEARNING, ICANN 2023, PT VI(2023)
摘要
Long sequence time-series forecasting (LSTF) has been widely applied in various fields, such as electricity usage planning and financial long-term strategic guidance. However, LSTF faces challenges in capturing two different types of information: the temporal dependencies of individual features and the interdependencies among multiple features in multivariate time series forecasting. Graph neural networks (GNNs) are commonly used to reveal the correlations among feature variables using graph structures in multivariate forecasting. However, in LSTF, the interdependencies among variables are often dynamic and evolving. Therefore, in this paper, we propose a Multi-scale Attention and Evolutionary Graph Structure (MAGNet) framework to address these challenges. To capture the dynamic changes in interdependencies among variables, we design an evolutionary graph learning layer that constructs an adjacency matrix for each time step and uses gated recurrent units to model the changing correlations, thus learning dynamic feature graph structures. We also utilize graph convolutional modules to capture the dependencies in the learned feature graph structure. Furthermore, to capture the two types of information that the temporal dependencies of individual features and the interdependencies among multiple features, we propose a multi-scale temporal capturing module that incorporates channel attention and spatial attention. Finally, we compare and analyze our proposed method against several high-performance models on 6 real-world datasets. Experimental results demonstrate the efficiency of the proposed method. Code is available at this repository: https://github.com/Masterleia/MAGNet.
更多查看译文
关键词
Time Series Forecasting,Long Time Series Forecasting,GNN,Attention,Deep Learning
AI 理解论文
溯源树
样例
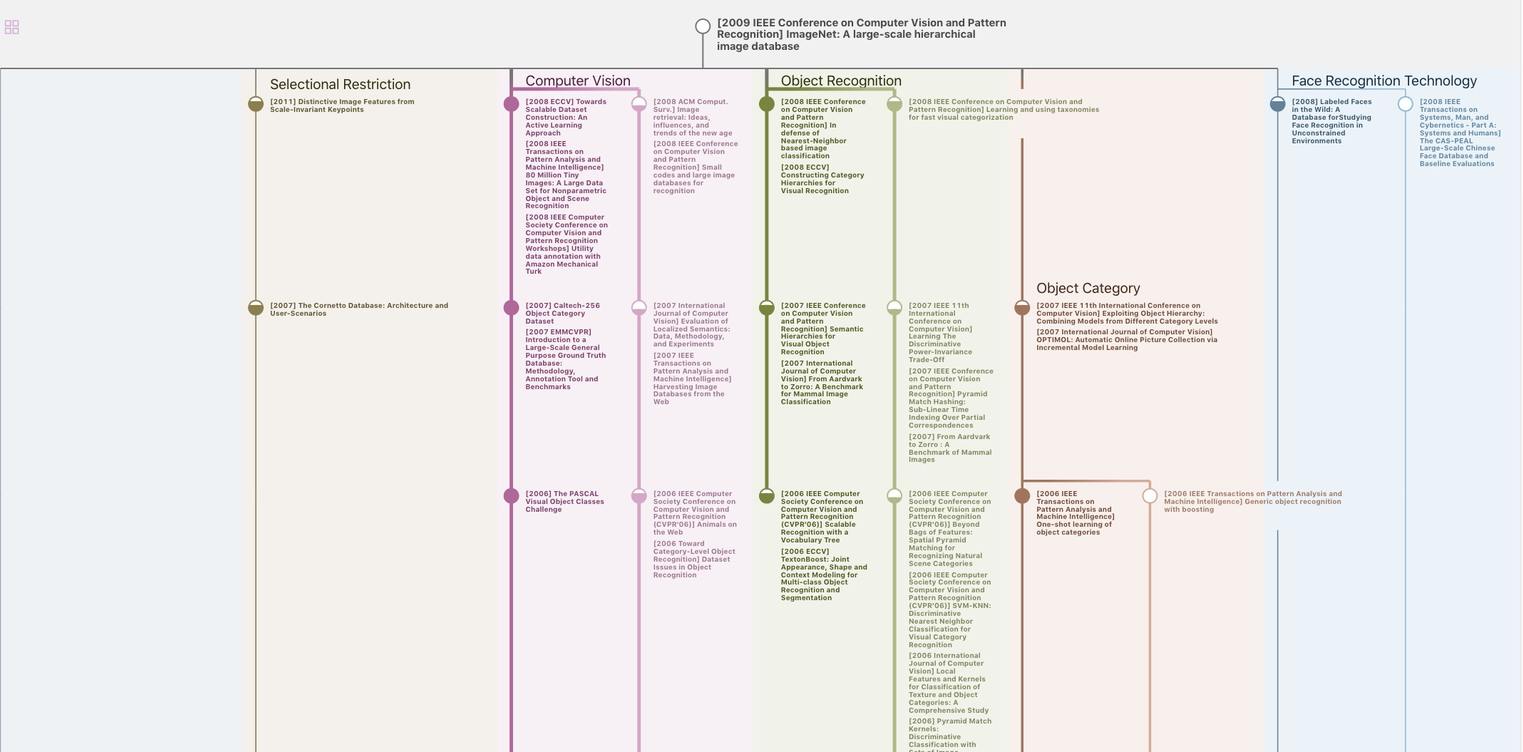
生成溯源树,研究论文发展脉络
Chat Paper
正在生成论文摘要