Tiny-YOLOv7: Tiny Object Detection Model for Drone Imagery.
Image and Graphics : 12th International Conference, ICIG 2023, Nanjing, China, September 22–24, 2023, Proceedings, Part III(2023)
摘要
With the rapid development of drones, tiny object detection in drone-captured scenarios has become a challenge task. However, the altitude of the drone changes while flying lead to the scale of the object changes dramatically. In addition, drones flying quickly cause motion blur on the densely tiny objects. In order to address the two issues mention above, we propose Tiny-YOLOv7. In order to detect multi-scale objects, we replace the original prediction heads with transformer prediction heads. For the motion blur issue, we propose DBS module to extract more visual elements and maintain computational cost of model. The DBS module consists of Dynamic Region-Aware Convolution (DRConv), Batch Normalization and Silu modules. On scenarios with dense objects, we additionally incorporate the Convolutional Block Attention Model (CBAM) to find the attention region of dense objects. Tiny-YOLOv7 is an effective and elegant method for handing tiny object detection. We validate our model through extensive experiments on VisDrone2021 and DOTA-v1.0 datasets. The results show that our method obtains remarkable improvements over the other models. In VisDrone2021 dataset, the mAP result of our method is 39.22 % , which is higher than SOTA method by 1.07 % . Furthermore, experiments on dataset DOTA-v1.0 demonstrate generalization of the propose model.
更多查看译文
关键词
tiny-yolov7 object detection model,drone imagery
AI 理解论文
溯源树
样例
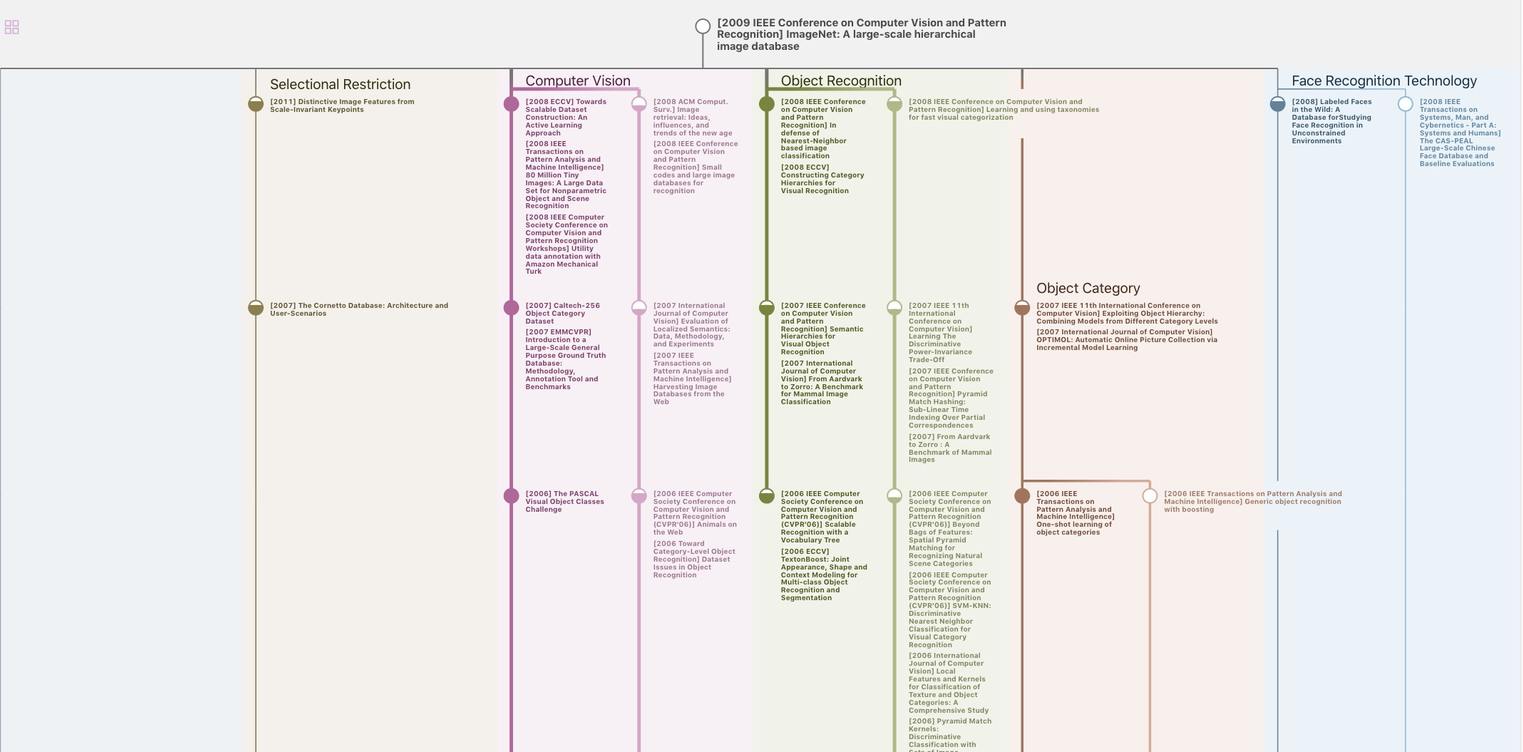
生成溯源树,研究论文发展脉络
Chat Paper
正在生成论文摘要