Coupled Dense Convolutional Neural Networks with Autoencoder for Unsupervised Hyperspectral Super-Resolution.
Image and Graphics : 12th International Conference, ICIG 2023, Nanjing, China, September 22–24, 2023, Proceedings, Part V(2023)
摘要
Hyperspectral Imagery (HSI) contains rich spectral information, but the resolution of hyperspectral imagery is often low sometimes. Recently, hyperspectral super-resolution technology has been developed to meet the needs of engineering applications. The new technology can mitigate many problems due to lower original spatial resolution. Nowadays, the development of deep learning provides many paths to design super-resolution methods and facilitates the development of related technologies. DenseNet, a sophisticated tool used to achieve prediction using deep networks, has found applications in various fields. Our contribution to this field involves the development of a coupled dense convolutional neural network (CoDenNet). It comprises three autoencoders that work together to acquire endmembers and abundances. Two of the three autoencoders have been designed explicitly for learning the parameters of the point spread function (PSF) alongside the spectral response function (SRF). The third autoencoder, on the other hand, fosters connections between different types of imagery: HSI and MSI. Compared with other super-resolution (SR) and fusion methods, We demonstrate the effectiveness and competitiveness of the proposed approach.
更多查看译文
关键词
dense convolutional neural networks,autoencoder,convolutional neural networks,super-resolution
AI 理解论文
溯源树
样例
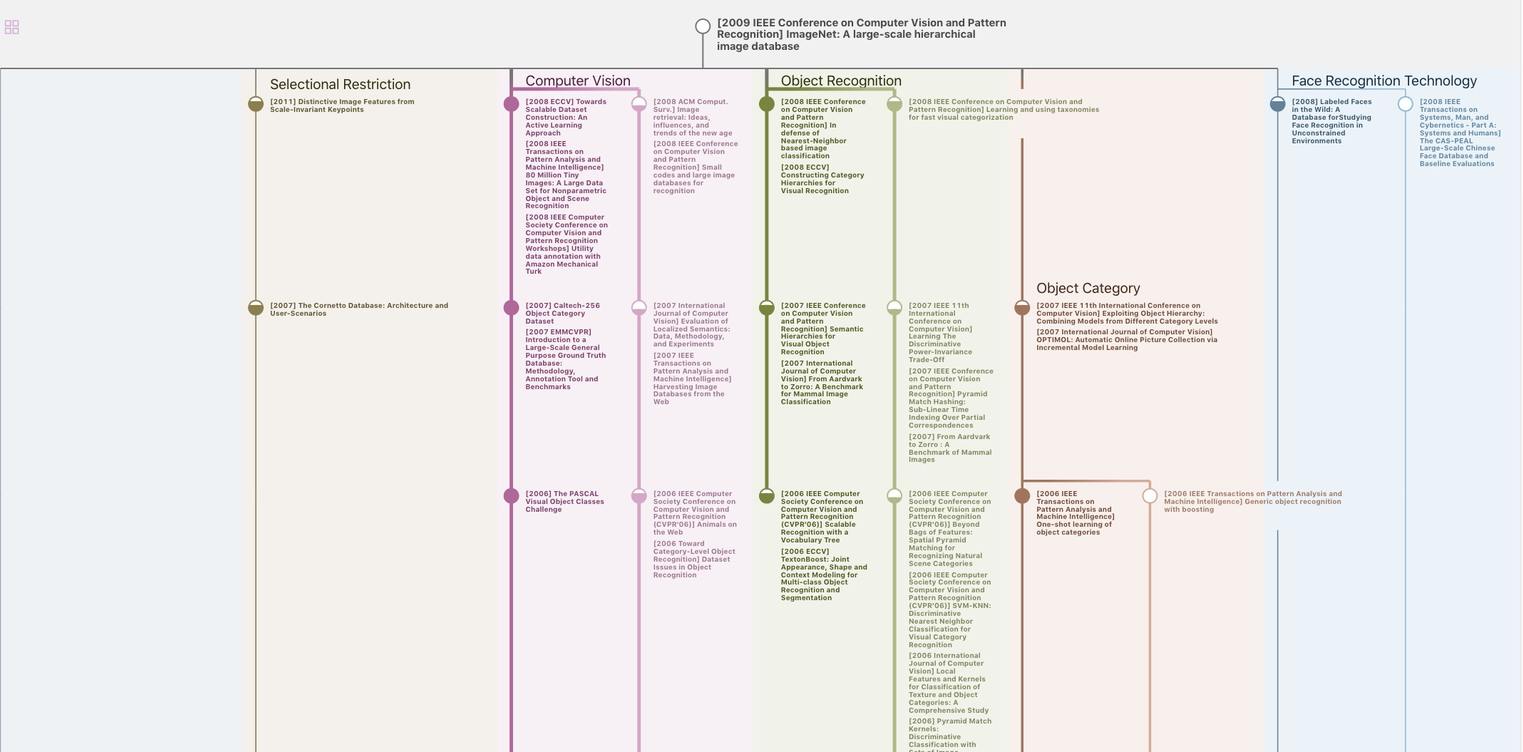
生成溯源树,研究论文发展脉络
Chat Paper
正在生成论文摘要