A Road Damage Segmentation Method for Complex Environment Based on Improved UNet.
Image and Graphics: 12th International Conference, ICIG 2023, Nanjing, China, September 22–24, 2023, Proceedings, Part I(2023)
摘要
Detecting and repairing road damage timely is crucial for ensuring traffic safety and reducing hazards. Cracks and potholes are the primary indications of early-stage road damage. However, existing deep learning-based methods for road damage detection often have limited feature extraction capabilities and only perform well in specific detection environments. To address these challenges, this paper proposes an improved UNet-based road damage segmentation method for complex environments. This method incorporates Atrous Spatial Pyramid Pooling (ASPP) and Coordinate Attention (CA) into the network, enhancing its ability to capture features of various sizes and to localize feature information. The proposed model effectively detects both cracks and potholes under different road environments, such as concrete, asphalt, and gravel. Experimental results demonstrate that the proposed network achieves 78.8% and 88.57% segmentation Intersection over Union (IoU) for cracks and potholes, respectively, outperforming classical semantic segmentation networks such as UNet, PSPNet, Attention UNet, UNet++, DANet, SegFormer, and TransUNet. This model can be applied to intelligent road inspection and maintenance to improve inspection efficiency.
更多查看译文
关键词
road damage segmentation method
AI 理解论文
溯源树
样例
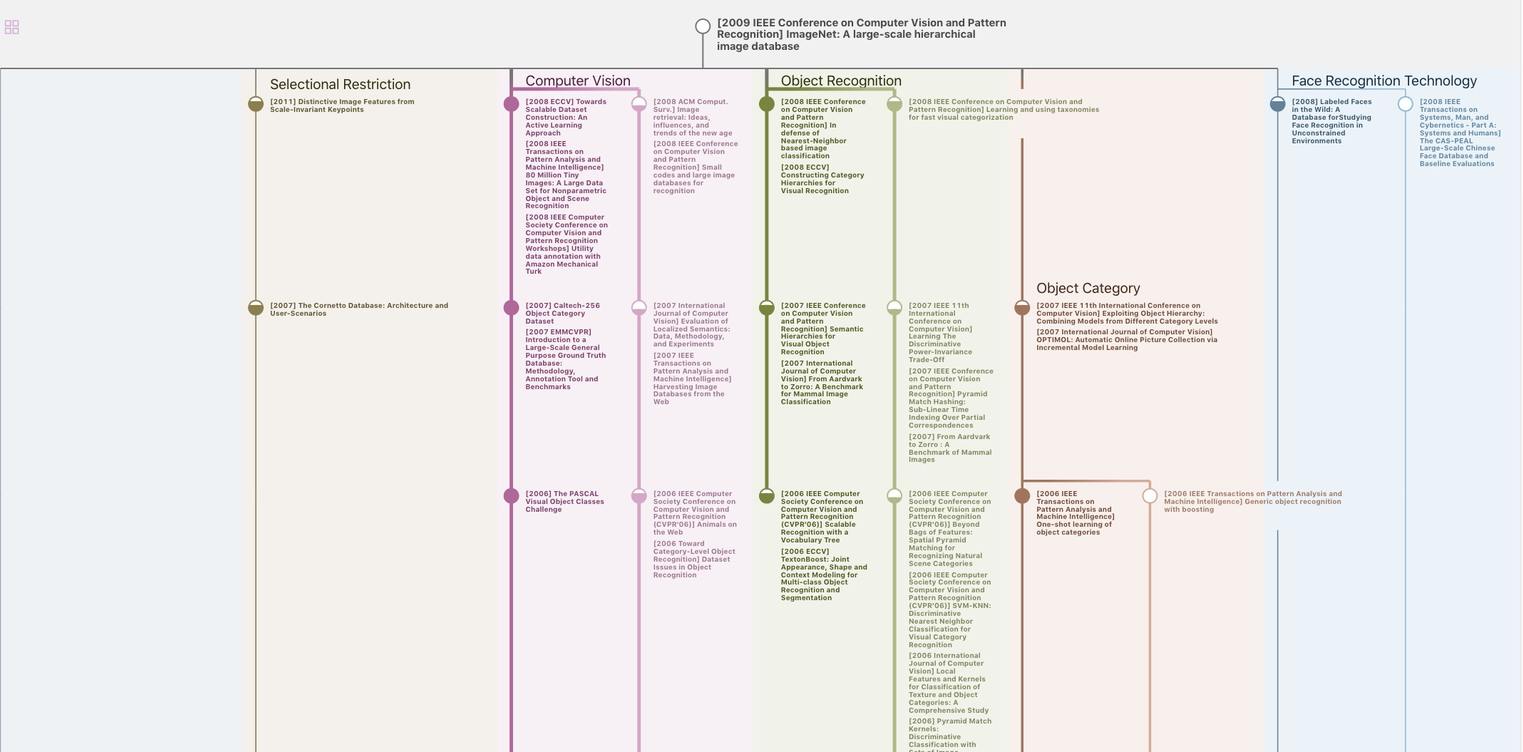
生成溯源树,研究论文发展脉络
Chat Paper
正在生成论文摘要