Experimental Digital Twin for Job Shops with Transportation Agents.
Learning and Intelligent Optimization: 17th International Conference, LION 17, Nice, France, June 4–8, 2023, Revised Selected Papers(2023)
摘要
Production scheduling in multi-stage manufacturing environments is subject to combinatorial optimization problems, such as the Job Shop Problem (JSP). The transportation of materials when assigned to mobile agents, such as Automated Guided Vehicles (AGVs), results in a Job Shop Problem with Transportation Agents (JSPTA). The transportation tasks require routing the AGVs within the physical space of the production environment. Efficient scheduling of production and material flow is thus crucial to enable flexible manufacturing systems. Neural combinatorial optimization has evolved to solve combinatorial optimization problems using deep Reinforcement Learning (RL). The key aim is to learn robust heuristics that tackle the trade-off of optimality versus time complexity and scale better to dynamic changes in the problem. The present simulation environments used to train RL agents for solving the JSPTA lack accessibility (e.g. use of proprietary software), configurability (e.g. changing shop floor layout), and extendability (e.g. implementing other RL methods). This research aims to address this gap by designing an Experimental Digital Twin (EDT) for the JSPTA. It represents an RL environment that considers the physical space for the execution of production jobs with transportation agents. We created our EDT using a simulation tool selected based on requirement analysis and tested it with a customized state-of-the-art neural combinatorial approach against two common Priority Dispatching Rules (PDRs). With a focus on the makespan, our findings reveal that the neural combinatorial approach outperformed the other PDRs, even when tested on unseen shop floor layouts. Furthermore, our results call for further investigation of multi-agent collaboration and layout optimization. Our EDT is a first step towards creating self-adaptive manufacturing systems and testing potential optimization scenarios before transferring them to real-world applications.
更多查看译文
关键词
digital twin,experimental digital twin,job shops
AI 理解论文
溯源树
样例
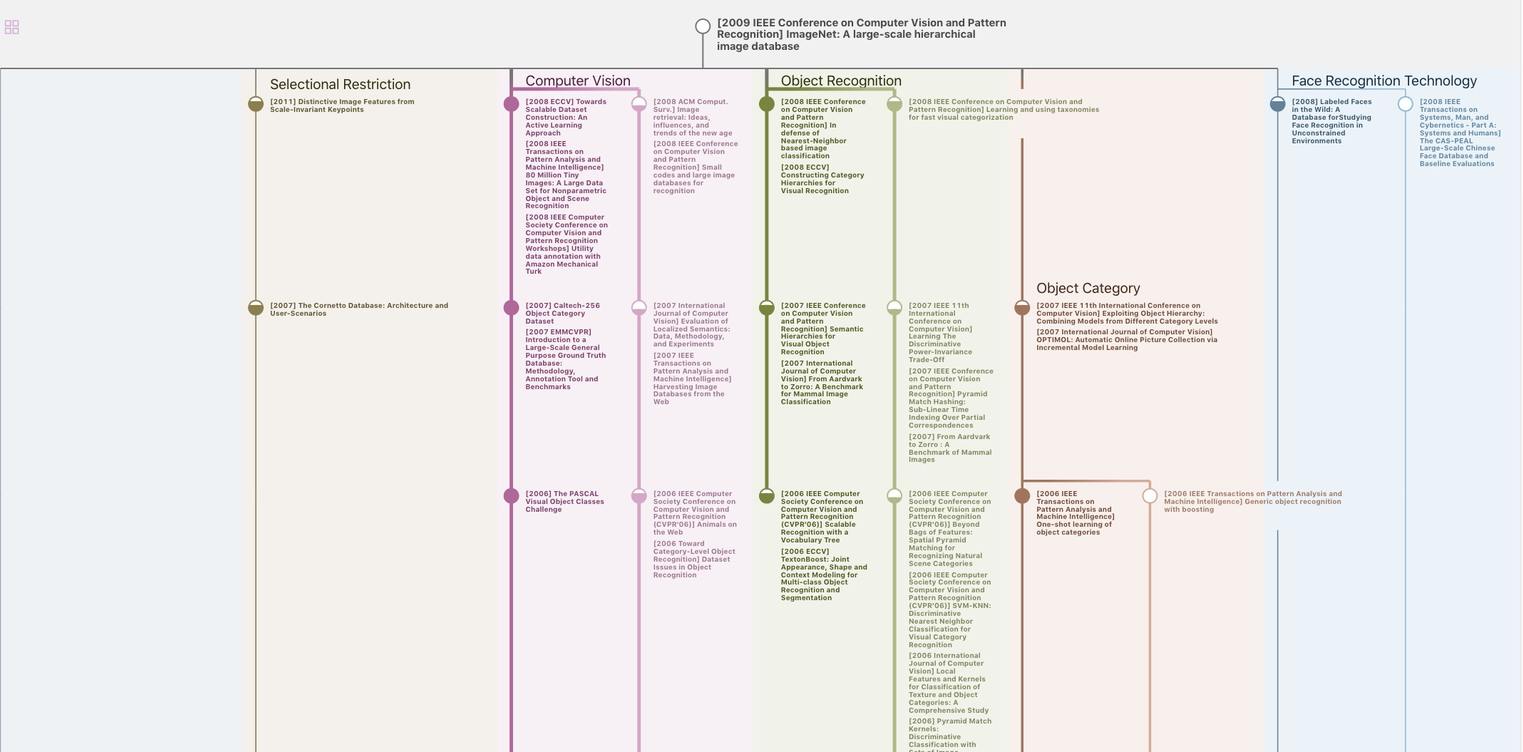
生成溯源树,研究论文发展脉络
Chat Paper
正在生成论文摘要