Anisotropic Operator Based on Adaptable Metric-Convolution Stage-Depth Filtering Applied to Depth Completion.
Pattern Recognition: 7th Asian Conference, ACPR 2023, Kitakyushu, Japan, November 5–8, 2023, Proceedings, Part III(2023)
摘要
Nowadays, depth maps are a crucial source of information for many applications based on artificial vision. Applications such as video games, 3D cinema, or unmanned autonomous vehicle control strongly depend on data extracted from depth maps. Depth maps can be estimated by algorithms or acquired by sensors (such as Kinect sensor, Time-of-Flight camera, or LiDAR sensor). Acquired depth data frequently contains holes or data with low confidence levels. An interpolation model is necessary to solve the problem of lack of data or complete these holes in depth maps. We constructed a manifold given the image domain and a metric whose parameters are learned from the data. The primary approach is to provide the parameterized metric enough flexibility to estimate the manifold’s shape correctly. Additionally, the proposal embedded depth estimation in a pipeline considering convolution stages and the anisotropic metric. We estimated the parameters’ proposal using the PSO algorithm. We assessed our proposal using the publicly available KITTI Depth Completion Suite dataset. Obtained results show that this proposal outperforms our previous implementation and other contemporary models. Additionally, we performed an ablation study of the model showing that the critical component of the model is the first convolution stage, meaning this stage that enforces the edges of the color image is a crucial component of the model.
更多查看译文
关键词
anisotropic,completion,metric-convolution,stage-depth
AI 理解论文
溯源树
样例
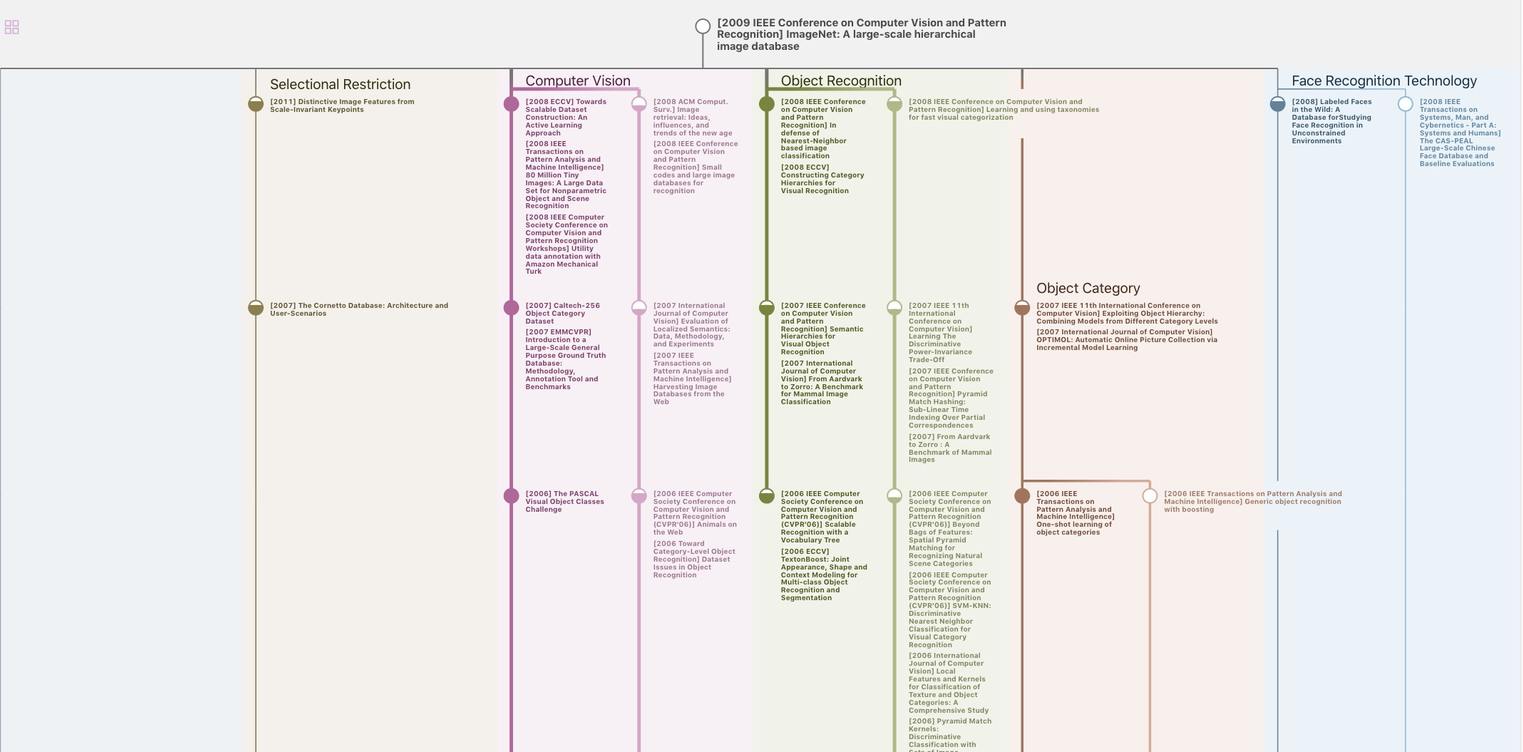
生成溯源树,研究论文发展脉络
Chat Paper
正在生成论文摘要