Diffusion Init: Stronger Initialisation of Decision-Based Black-Box Attacks for Visual Object Tracking.
Pattern Recognition: 7th Asian Conference, ACPR 2023, Kitakyushu, Japan, November 5–8, 2023, Proceedings, Part II(2023)
摘要
Adversarial attacks have emerged in the field of visual object tracking to mislead the tracker and result in its failure. Black-box attacks in particular have attracted increasing attention for their affinity with real-world applications. In the paradigm of decision-based black-box attacks, the magnitude of perturbation is gradually amplified, while the optimisation direction is predefined by an initial adversarial sample. Considering the pivotal role played by the initial adversarial sample in determining the success of an attack, we utilise the noise generated from the reverse process of a diffusion model as a better attacking direction. On the one hand, the diffusion model generates Gaussian noise, which formulate global information interaction, with a comprehensive impact on Transformer-based trackers. On the other hand, the diffusion model pays more attention to the target region during the inverse process, resulting in a more powerful perturbation of the target object. Our method, which is widely applicable, has been validated on a range of trackers using several benchmarking datasets. It is shown to deliver more extensive tracking performance degradation, compared to other state-of-the-art methods. We also investigate different approaches to the problem of generating the initial adversarial sample, confirming the effectiveness and rationality of our proposed diffusion initialisation method.
更多查看译文
关键词
object tracking,attacks,stronger initialisation,diffusion,decision-based,black-box
AI 理解论文
溯源树
样例
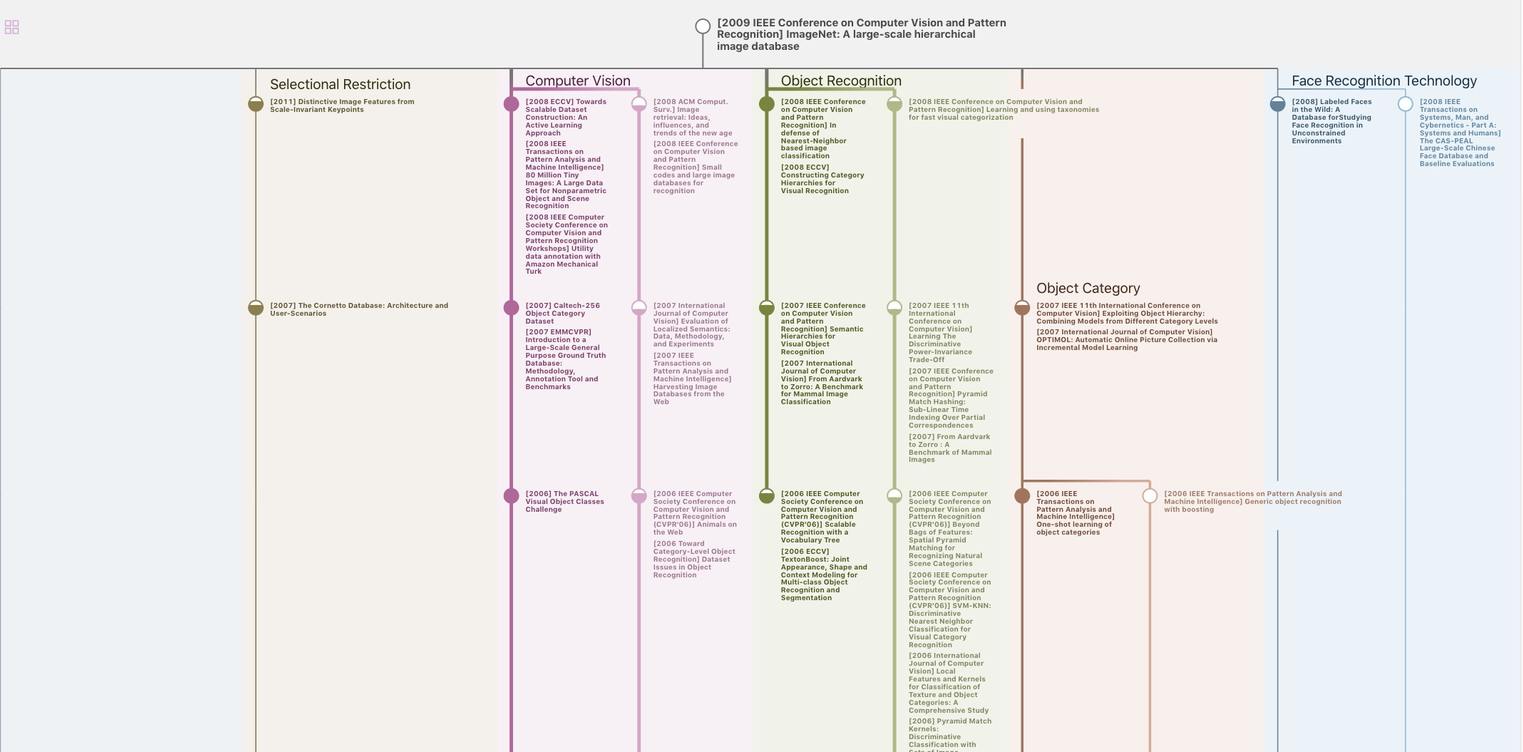
生成溯源树,研究论文发展脉络
Chat Paper
正在生成论文摘要