KDED: A Knowledge Distillation Based Edge Detector.
PRICAI 2023: Trends in Artificial Intelligence: 20th Pacific Rim International Conference on Artificial Intelligence, PRICAI 2023, Jakarta, Indonesia, November 15–19, 2023, Proceedings, Part III(2023)
摘要
Deep learning-based edge detectors are successful due to the large amount of supervisory information provided by manual labeling. However, there are inevitably errors in the manually labeled supervisory information (MLSI), which greatly mislead the learning of the models and become the bottleneck of deep learning-based edge detectors. To solve the drawbacks of MLSI, we propose a novel Knowledge Distillation based Edge Detector (KDED). By means of knowledge distillation, MLSI is transformed into edge probability map to supervise the learning of the models, which can effectively correct the errors in MLSI and represents disputed edges by probability. Adapting to the new training strategy and solving the sample imbalance problem, the Sample Balance Loss is proposed, which ensures the stability of the model and improve the accuracy. The experimental results indicate that KDED remarkably improves the accuracy without increasing the parameters and the computational cost. KDED achieves an ODS F-measure of 0.832 with 14.8 M parameters on BSDS500 dataset, which is significantly super to the results of previous methods. The source code is available at this link .
更多查看译文
关键词
detector,knowledge,distillation,knowledge
AI 理解论文
溯源树
样例
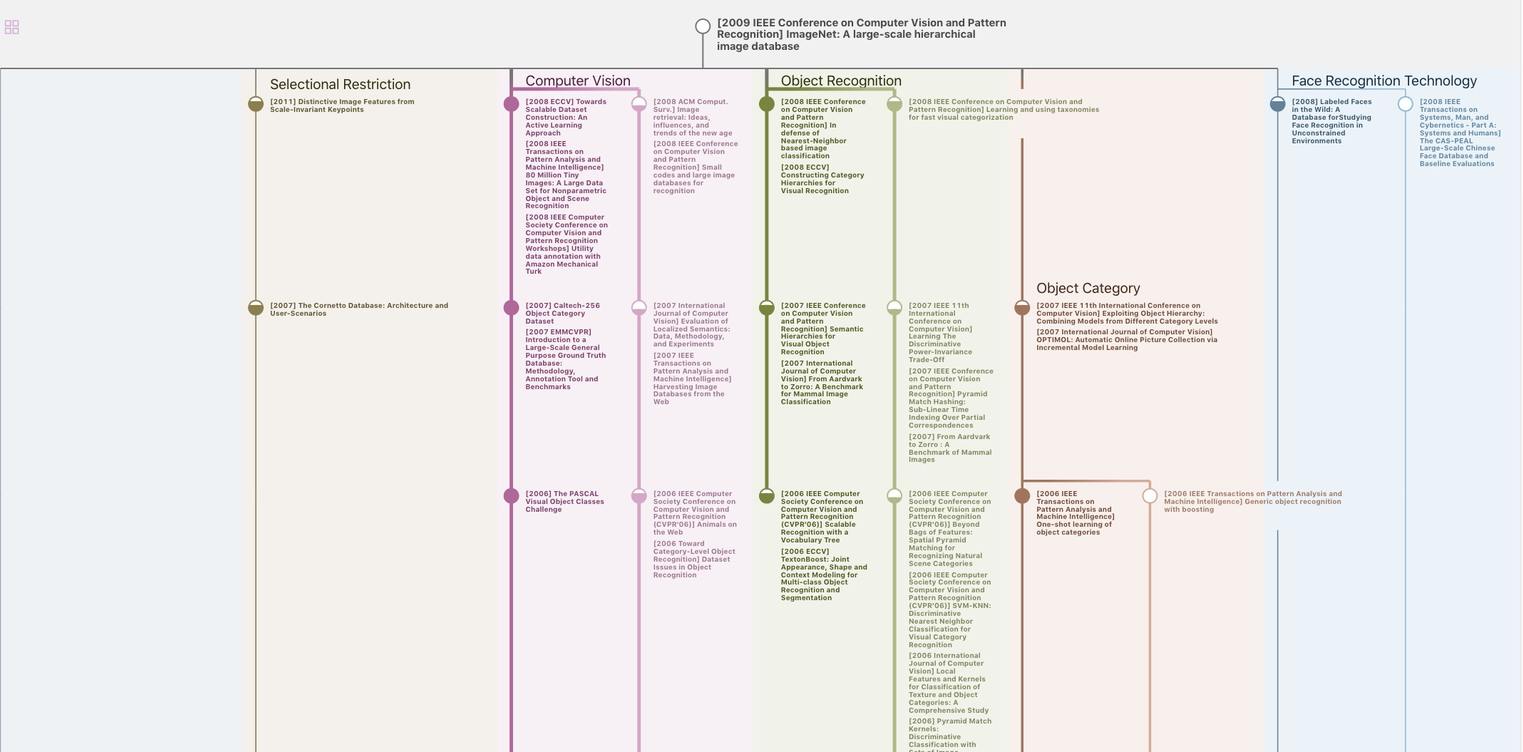
生成溯源树,研究论文发展脉络
Chat Paper
正在生成论文摘要