Enhancing the Interpretability of Deep Multi-agent Reinforcement Learning via Neural Logic Reasoning
ARTIFICIAL NEURAL NETWORKS AND MACHINE LEARNING, ICANN 2023, PART X(2023)
摘要
Explaining the decision-making policies of deep reinforcement learning is challenging owing to the black-box nature of neural networks. We address this challenge by combining deep learning models and symbolic structures into a neural-logic model that reasons in the form of neural logic programming. The proposed explainable multi-agent reinforcement learning algorithm performs reasoning in a symbolic-represented environment using multi-hop reasoning, a relational pathsearching method that uses prior symbolic knowledge. Furthermore, to alleviate the partial observability problem in multi-agent systems, we devised an explainable history module using an attention mechanism to incorporate past experiences while preserving interpretability. Experimental studies demonstrate that the proposed method can effectively learn close-to-optimal policies while generating expressive rules to explain the decisions. Particularly, it can learn more abstract concepts than conventional neural network approaches.
更多查看译文
关键词
reinforcement learning,multi-agent,neural logic programming
AI 理解论文
溯源树
样例
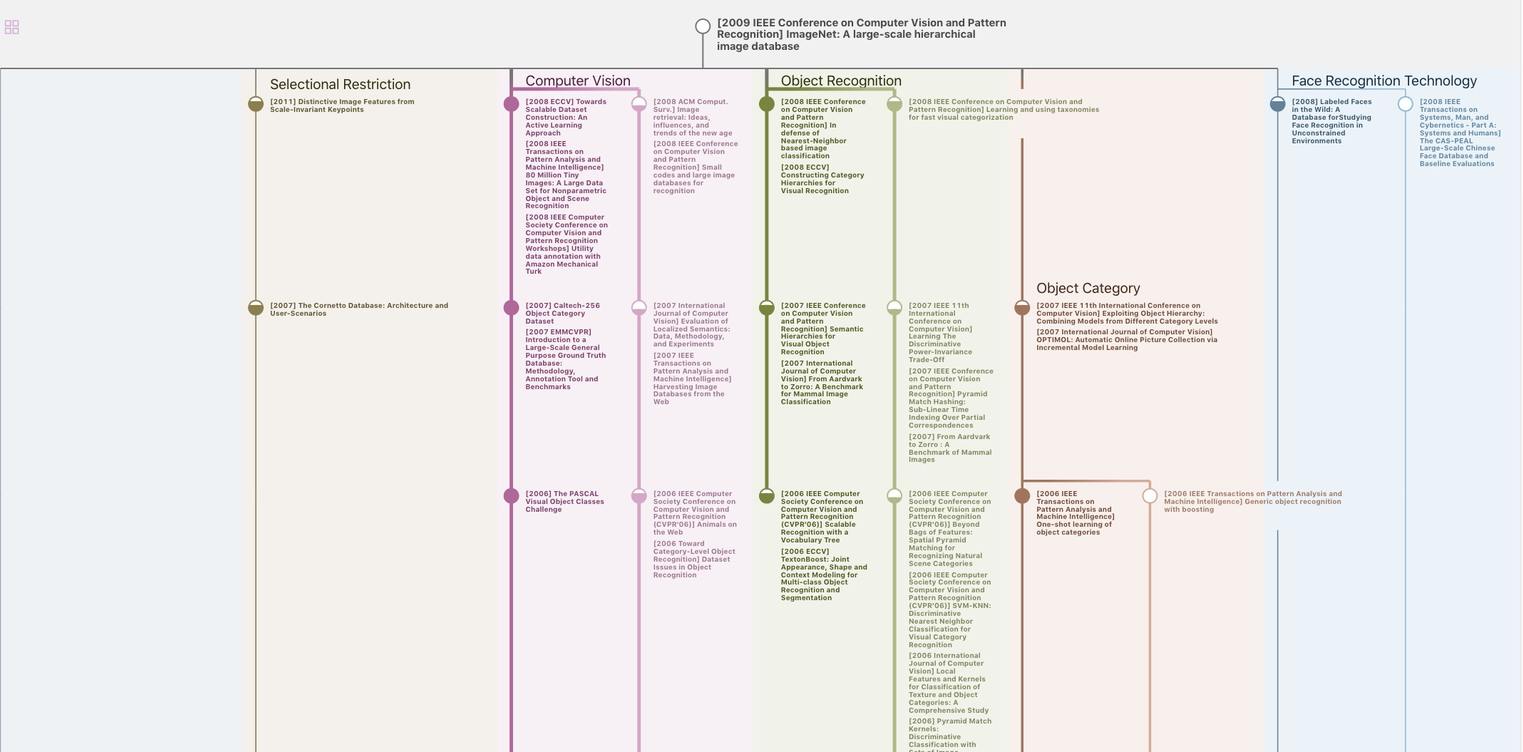
生成溯源树,研究论文发展脉络
Chat Paper
正在生成论文摘要