Towards Reliable and Efficient Vegetation Segmentation for Australian Wheat Data Analysis
DATABASES THEORY AND APPLICATIONS, ADC 2023(2024)
摘要
Automated crop data analysis plays an important role in modern Australian agriculture. As one of the key procedures of analysis, vegetation segmentation, which aims to predict pixel-level labels of vegetation images, has recently demonstrated advanced results on benchmark datasets. However, the promising results are built upon the assumption that the test data and the training data always follow an identical distribution. Due to the differences in vegetation species, country, or illumination conditions, such assumptions are commonly violated in the real-world scenario. As a pilot study, this work confirms the model pre-trained on worldwide vegetation data has a degradation issue when being applied to the Australian wheat data. Instead of conducting expensive pixel-level annotation of Australian wheat data, we propose a self-training strategy that incorporates confidence estimated pseudo-labeling of the wheat data in the training process to close the distribution gap. Meanwhile, to reduce the computational cost, we equip the lightweight transformer framework with a token clustering and reconstruction module. Extensive experimental results demonstrate that the proposed network can achieve 6.4% higher mIOU and 8.6% lower computational costs over the baseline methods.
更多查看译文
AI 理解论文
溯源树
样例
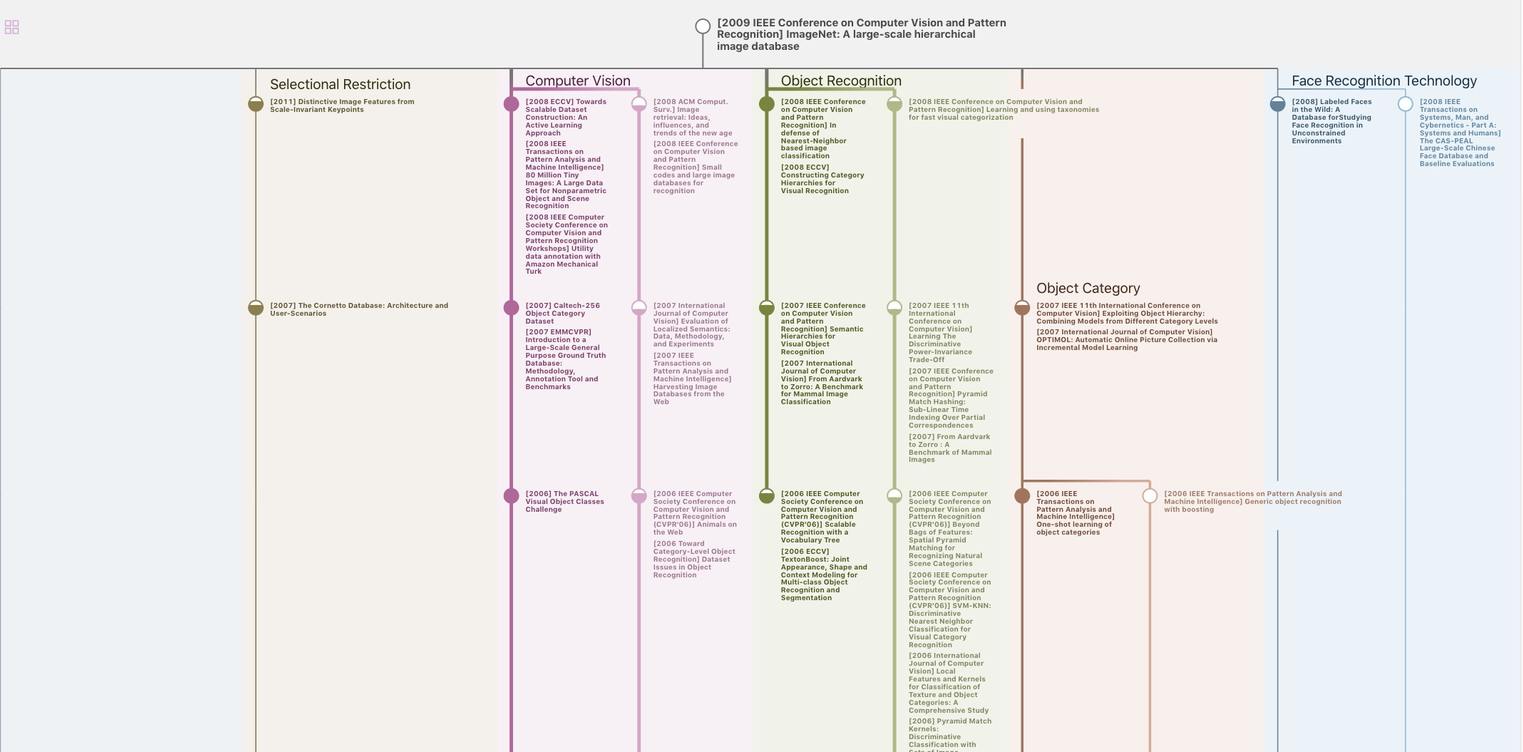
生成溯源树,研究论文发展脉络
Chat Paper
正在生成论文摘要