Bayesian denoising algorithm dealing with colored, non-stationary noise in continuous glucose monitoring timeseries
FRONTIERS IN BIOENGINEERING AND BIOTECHNOLOGY(2023)
摘要
Introduction: The retrospective analysis of continuous glucose monitoring (CGM) timeseries can be hampered by colored and non-stationary measurement noise. Here, we introduce a Bayesian denoising (BD) algorithm to address both autocorrelation of measurement noise and temporal variability of its variance.Methods: BD utilizes adaptive, a-priori models of signal and noise, whose unknown variances are derived on partially-overlapped CGM windows, via smoothing approach based on linear mean square estimation. The CGM signal and noise variability profiles are then reconstructed using a kernel smoother. BD is first assessed on two simulated datasets, DS1 and DS2. On DS1, the effectiveness of accounting for colored noise is evaluated by comparison against a literature algorithm; on DS2, the effectiveness of accounting for the noise variance temporal variability is evaluated by comparison against a Butterworth filter. BD is then evaluated on 15 CGM timeseries measured by the Dexcom G6 (DR).Results: On DS1, BD allows reducing the root-mean-square-error (RMSE) from 8.10 [6.79-9.24] mg/dL to 6.28 [5.47-7.27] mg/dL (median [IQR]); on DS2, RMSE decreases from 6.85 [5.50-8.72] mg/dL to 5.35 [4.48-6.49] mg/dL. On DR, BD performs a reasonable tracking of noise variance variability and a satisfactory denoising.Discussion: The new algorithm effectively addresses the nature of CGM measurement error, outperforming existing denoising algorithms.
更多查看译文
关键词
Bayesian denoising,continuous glucose monitoring,correlation,stationarity,Butterworth filter
AI 理解论文
溯源树
样例
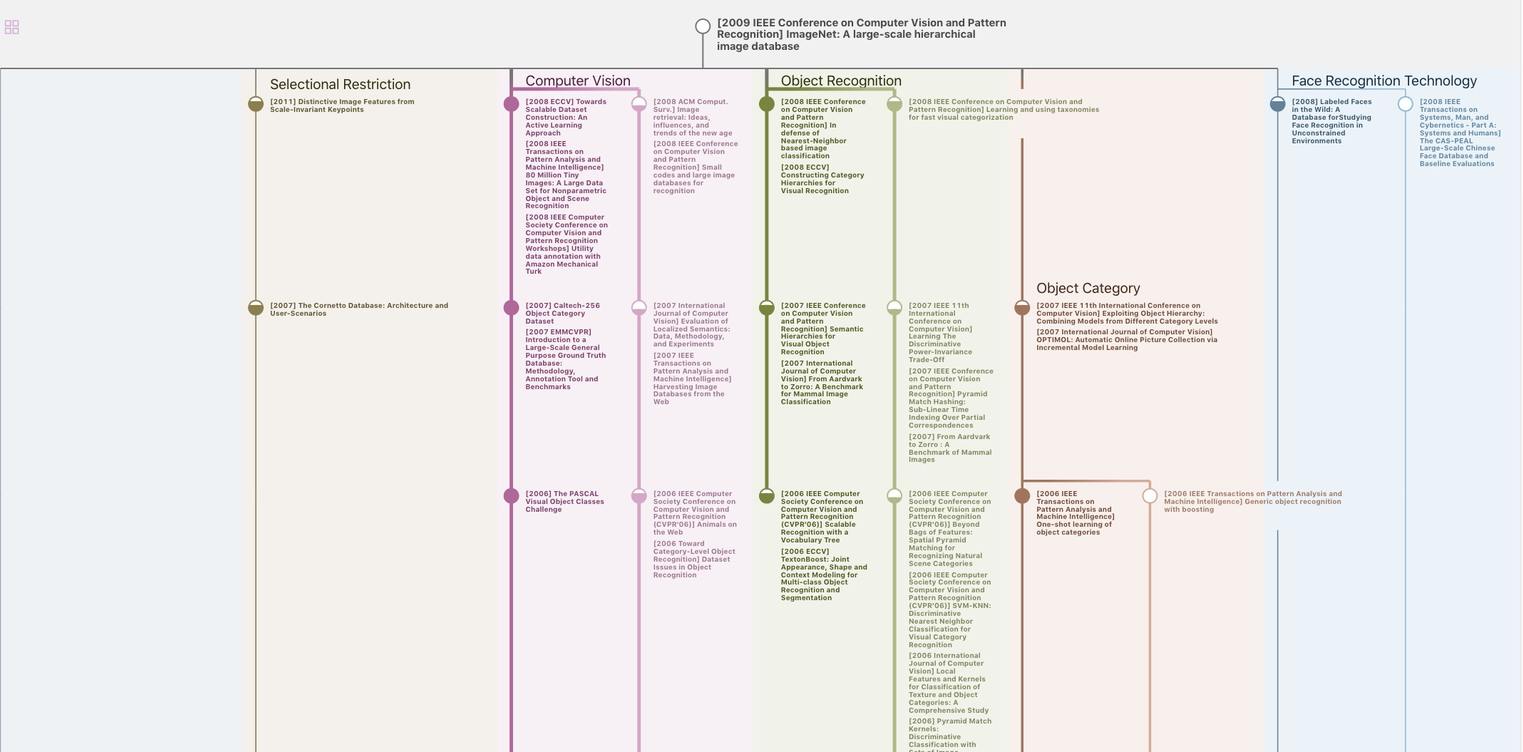
生成溯源树,研究论文发展脉络
Chat Paper
正在生成论文摘要