A Data Augmentation Algorithm for Trajectory Data.
EMODE '23: Proceedings of the 1st ACM SIGSPATIAL International Workshop on Methods for Enriched Mobility Data: Emerging issues and Ethical perspectives 2023(2023)
摘要
The growing prevalence of location-based devices has resulted in a significant abundance of location data from various tracking vendors. Nevertheless, a noticeable deficit exists regarding readily accessible, extensive, and publicly available datasets for research purposes, primarily due to privacy concerns and ownership constraints. There is a pressing need for expansive datasets to advance machine learning techniques in this domain. The absence of such resources currently represents a substantial hindrance to research progress in this field. Data augmentation is emerging as a popular technique to mitigate this issue in several domains. However, applying state-of-the-art techniques as-is proves challenging when dealing with trajectory data due to the intricate spatio-temporal dependencies inherent to such data. In this work, we propose a novel strategy for augmenting trajectory data that applies a geographical perturbation on trajectory points along a trajectory. Such a perturbation results in controlled changes in the raw trajectory and, consequently, causes changes in the trajectory feature space. We test our strategy in two trajectory datasets and show a performance improvement of approximately 20% when contrasted with the baseline. We believe this strategy will pave the way for a more comprehensive framework for trajectory data augmentation that can be used in fields where few labeled trajectory data are available for training machine learning models.
更多查看译文
AI 理解论文
溯源树
样例
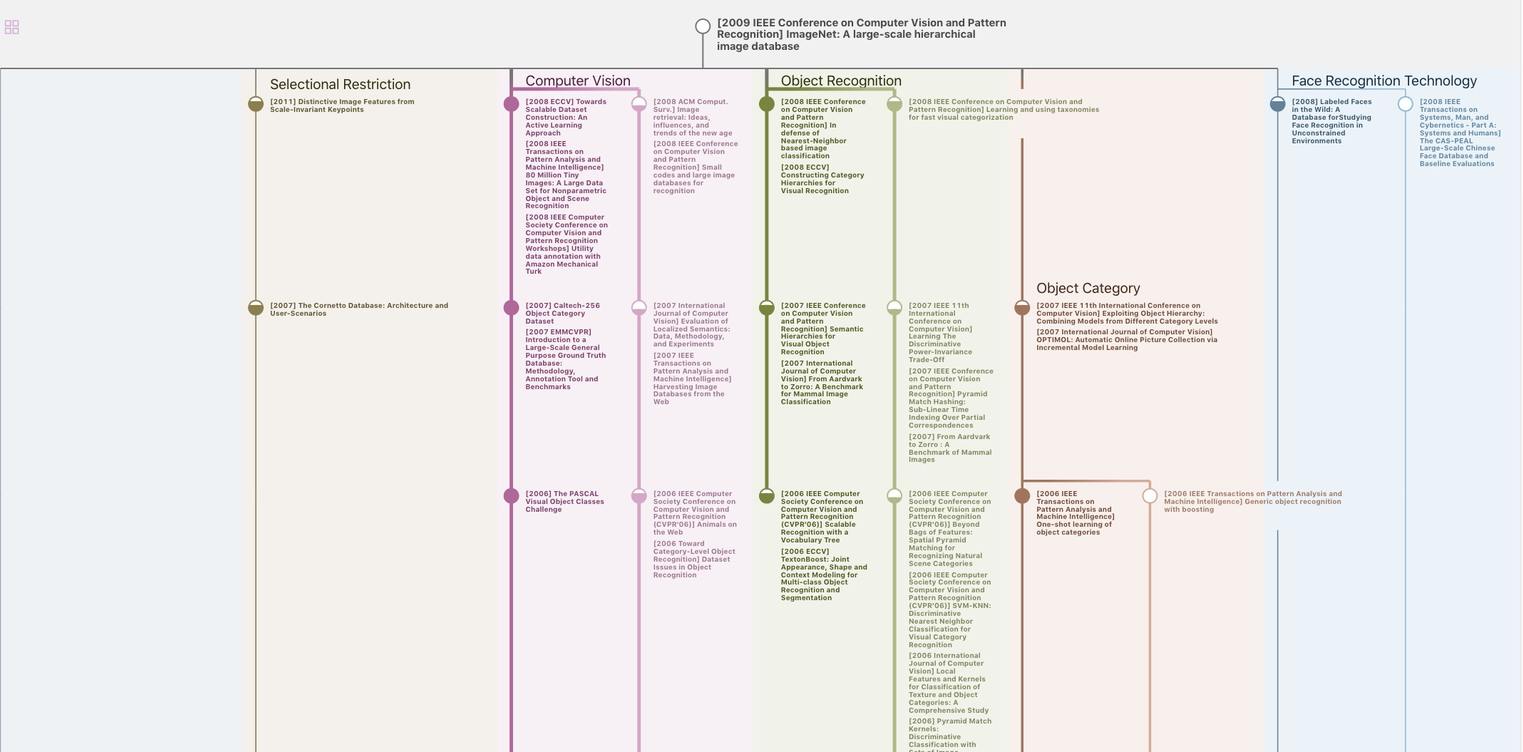
生成溯源树,研究论文发展脉络
Chat Paper
正在生成论文摘要