Overcoming Data Scarcity through Transfer Learning in CO2-Based Building Occupancy Detection
PROCEEDINGS OF THE 10TH ACM INTERNATIONAL CONFERENCE ON SYSTEMS FOR ENERGY-EFFICIENT BUILDINGS, CITIES, AND TRANSPORTATION, BUILDSYS 2023(2023)
摘要
Knowing indoor occupancy states is crucial for energy optimization in buildings. While neural networks can effectively be used to detect occupancy based on carbon dioxide measurements, their application is impeded by the need for sufficient labeled training data. In this study, we analyze the prediction performance of three different transfer learning (TL) methods leveraging target room data jointly with data from other rooms. The methods include (1) pretraining and fine-tuning, (2) layer freezing, and (3) domain-adversarial learning. Using data from five real-world rooms and one simulated room, including multiple room types, we provide the most extensive evaluation of TL in the field of occupancy prediction from environmental variables to date. This work's contribution further includes the architecture and hyperparameters of a deep CNN-LSTM model for CO2-based occupancy detection. Our results indicate that TL effectively reduces the required amount of target room data. Moreover, while previous literature was focused on pretraining with related real-world data, we show that similar performance can be achieved by the more practical approach of leveraging simulated data.
更多查看译文
关键词
Transfer Learning,Deep Learning,CNN-LSTM,Building Occupancy Detection,Indoor Carbon Dioxide
AI 理解论文
溯源树
样例
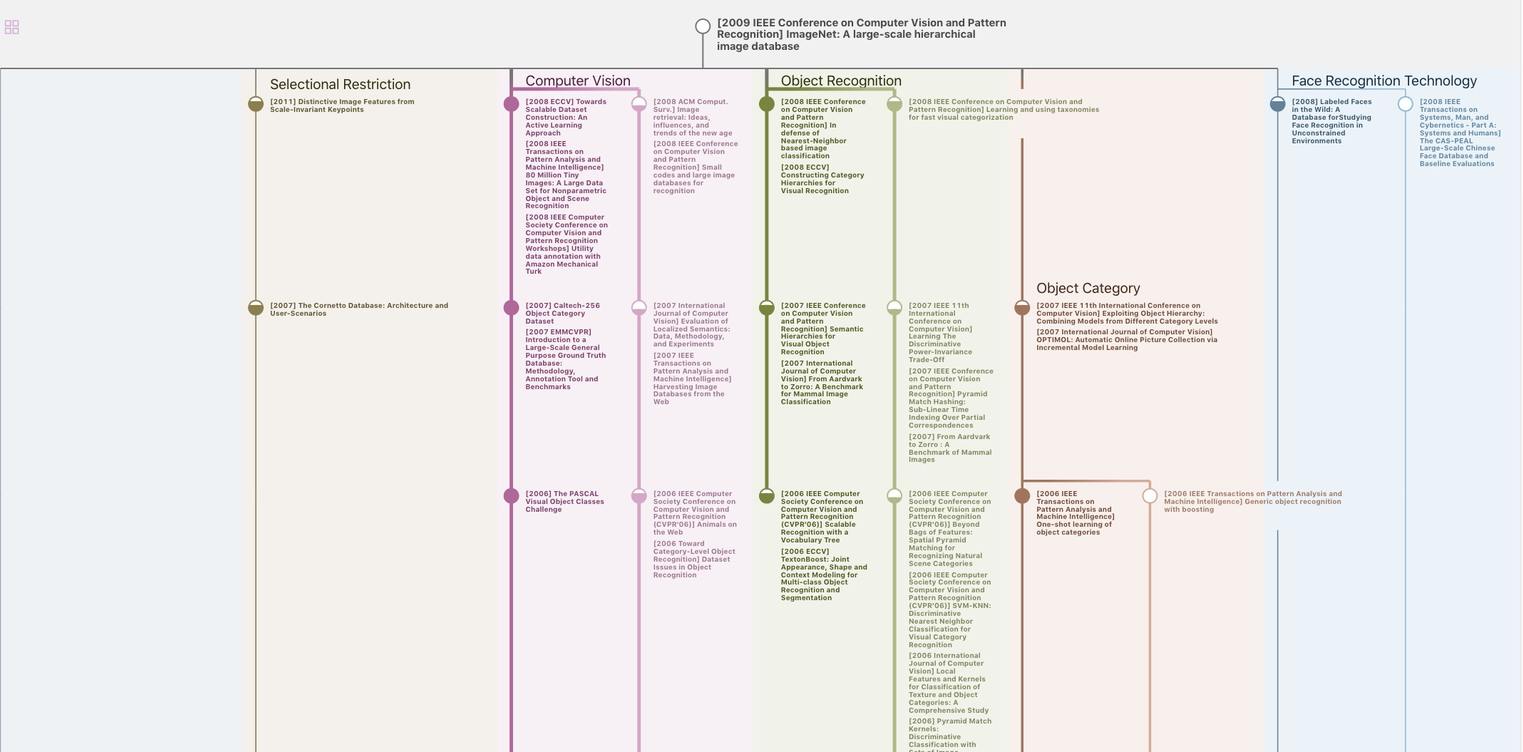
生成溯源树,研究论文发展脉络
Chat Paper
正在生成论文摘要