Signal Separation in Global, Temporal Gravity Data
acm(2024)
摘要
Satellite gravity data such as provided by the Gravity Recovery and Climate Experiment (GRACE) and its follow-up mission contain valuable information on all geophysical processes that involve a mass redistribution in the Earth system. However, as gravity is an integral quantity, only the sum of all signal components can be measured. In order to exploit gravity data for improving the geophysical understanding of the underlying mass redistribution processes, an algorithm to separate the superimposed signals is needed. We present two methods that solve the signal separation problem in global, spatial-temporal gravity data based on prior knowledge on the characteristic behavior of the individual signal components: A principal component analysis-based method is compared to a conditional generative adversarial network that has been originally developed for image-to-image translation tasks. Both methods are tested on synthetic model data in a closed-loop setup, and are shown to successfully accomplish the task of separating gravity signals related to atmospheric and oceanic processes from signals caused by processes in the continental hydrosphere. The two methods show a comparable level of prediction errors. For both methods, the preprocessing of the gravity signals before the signal separation step, more precisely, the choice of the gravity field functional, has a considerable impact on the predictive performance. All in all, we emphasize the potential of neural network algorithms for signal separation in spatial-temporal geodetic data, and suggest a more specific tuning of the algorithm to the task of interest.
更多查看译文
关键词
satellite gravimetry,signal separation,spatial-temporal data,neural networks
AI 理解论文
溯源树
样例
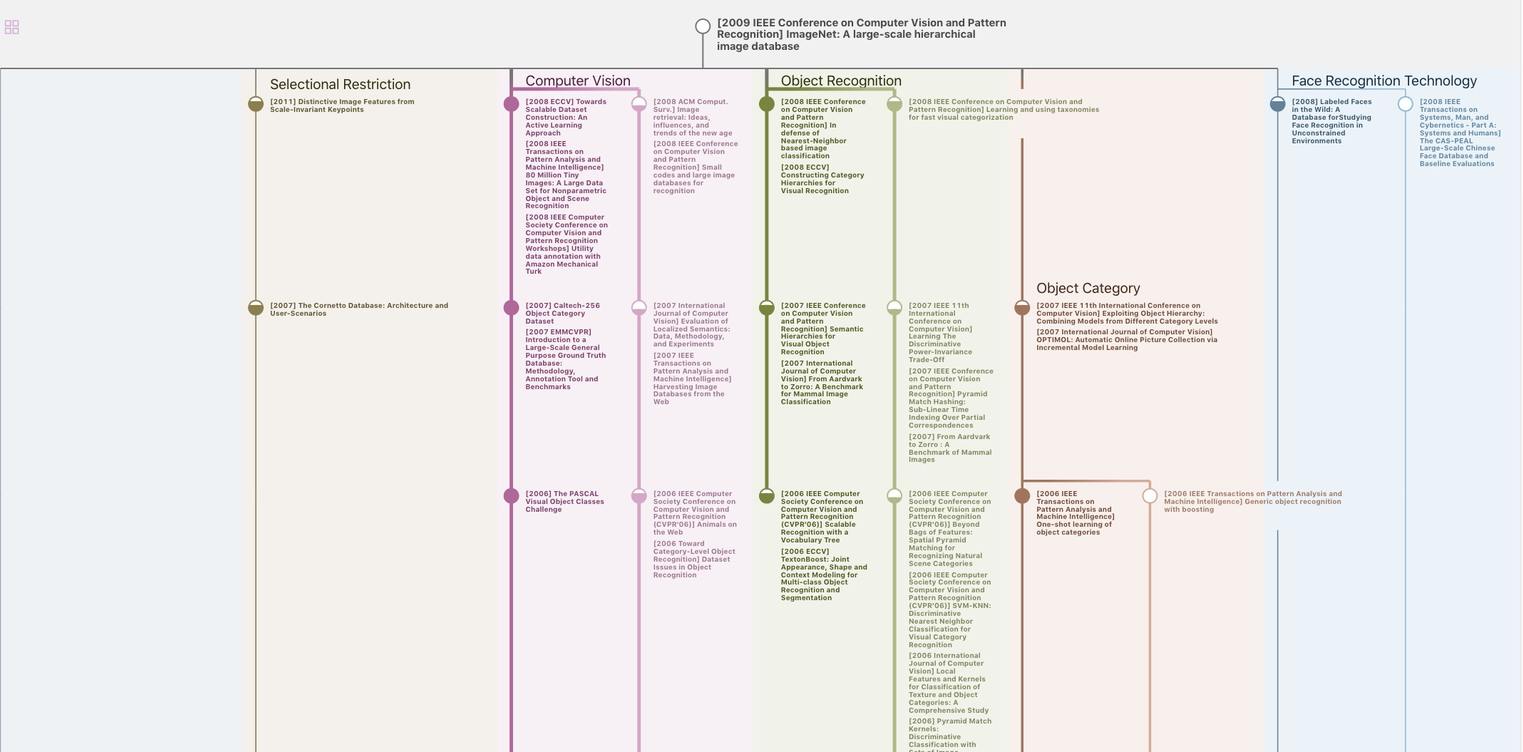
生成溯源树,研究论文发展脉络
Chat Paper
正在生成论文摘要