Contrastive Pretraining for Railway Detection: Unveiling Historical Maps with Transformers
PROCEEDINGS OF THE 6TH ACM SIGSPATIAL INTERNATIONAL WORKSHOP ON AI FOR GEOGRAPHIC KNOWLEDGE DISCOVERY, GEOAI 2023(2023)
摘要
Detecting railways from historical maps is challenging due to their infrequent representation in a map sheet and their visual similarity with roads. Basically, both railways and roads are symbolised as two parallel black lines, with slight differences only in line thickness. Recent advancements in transformer models for computer vision tasks have sparked interest in utilizing them for processing historical maps. However, the success of transformers heavily relies on large-scale labelled datasets, predominantly available for ground imagery rather than historical maps. To overcome these challenges, we exploit the unique spatial characteristics of historical map data, where the same location can be depicted over different time spans across different map series. For example, each location in Switzerland is depicted in both the Siegfried map and the Old National map, each exhibiting distinct symbols and drawing styles. In this work, we address the scarcity of labelled data by generating positive pairs of the same scene from different map series and employ self-supervised contrastive learning to pre-train a dedicated transformer encoder optimized for map data. Subsequently, we fine-tune the entire transformer network for the downstream railway detection task. Experimental results demonstrate that our method achieves comparable performance to fully supervised approaches, while significantly reducing the amount of required labelled dataset to a mere 2.5% after contrastive pretraining.
更多查看译文
关键词
railway detection,map processing,contrastive learning,Transformer,neural networks
AI 理解论文
溯源树
样例
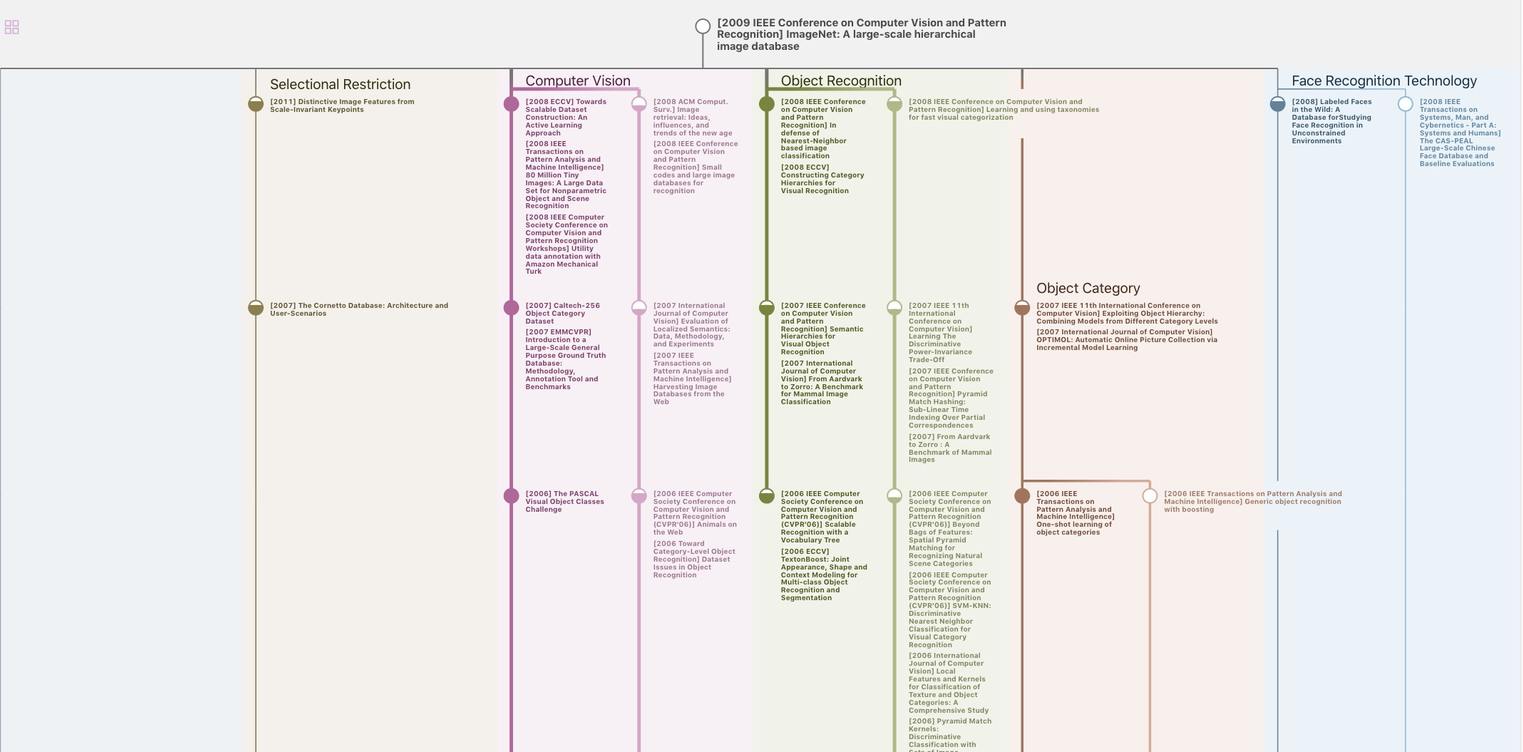
生成溯源树,研究论文发展脉络
Chat Paper
正在生成论文摘要