Automatic Nested Spatial Entity and Spatial Relation Extraction from Text for Knowledge Graph Creation: A Baseline Approach and a Benchmark Dataset
Proceedings of the 7th ACM SIGSPATIAL International Workshop on Geospatial Humanities(2023)
摘要
Automatically extracting geographic information from text is the key to harnessing the vast amount of spatial knowledge that only exists in this unstructured form. The fundamental elements of spatial knowledge include spatial entities, their types and the spatial relations between them. Structuring the spatial knowledge contained within text as a geospatial knowledge graph, and disambiguating the spatial entities, significantly facilitates its reuse. The automatic extraction of geographic information from text also allows the creation or enrichment of gazetteers. We propose a baseline approach for nested spatial entity and binary spatial relation extraction from text, a new annotated French-language benchmark dataset on the maritime domain that can be used to train algorithms for both extraction tasks, and benchmark results for the two tasks carried out individually and end-to-end. Our approach involves applying the Princeton University Relation Extraction system (PURE), made for flat, generic entity extraction and generic binary relation extraction, to the extraction of nested, spatial entities and spatial binary relations. By extracting nested spatial entities and the spatial relations between them, we have more information to aid entity disambiguation. In our experiments we compare the performance of a pretrained monolingual French BERT language model with that of a pretrained multilingual BERT language model, and study the effect of including cross-sentence context. Our results reveal very similar results for both models, although the multilingual model performs slightly better in entity extraction, and the monolingual model has slightly better relation extraction and end-to-end performances. We observe that increasing the amount of cross-sentence context improves the results for entity extraction whereas it has the opposite effect on relation extraction.
更多查看译文
关键词
geographic information,spatial knowledge,maritime data,nested spatial entity,binary spatial relation,deep learning,neural network,language model
AI 理解论文
溯源树
样例
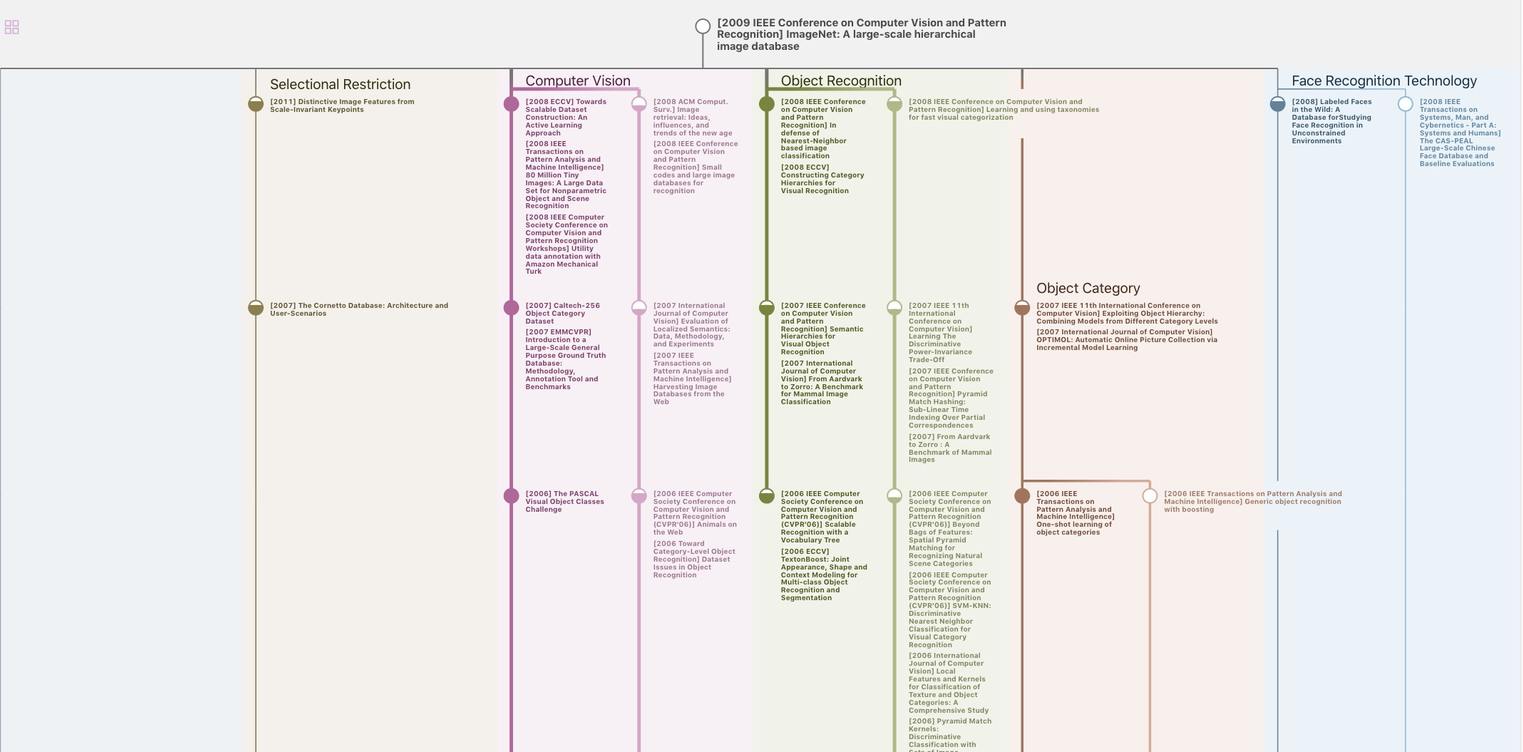
生成溯源树,研究论文发展脉络
Chat Paper
正在生成论文摘要