GAN-Based Semi-Supervised Training of LSTM Nets for Intention Recognition in Cooperative Tasks
IEEE ROBOTICS AND AUTOMATION LETTERS(2024)
摘要
The accumulation of a sufficient amount of data for training deep neural networks is a major hindrance in the application of deep learning in robotics. Acquiring real-world data requires considerable time and effort, yet it might still not capture the full range of potential environmental variations. The generation of new synthetic data based on existing training data has been enabled with the development of generative adversarial networks (GANs). In this paper, we introduce a training methodology based on GANs that utilizes a recurrent, LSTM-based architecture for intention recognition in robotics. The resulting networks predict the intention of the observed human or robot based on input RGB videos. They are trained in a semi-supervised manner, with the output classification networks predicting one of possible labels for the observed motion, while the recurrent generator networks produce fake RGB videos that are leveraged in the training process. We show that utilization of the generated data during the network training process increases the accuracy and generality of motion classification compared to using only real training data. The proposed method can be applied to a variety of dynamic tasks and different LSTM-based classification networks to supplement real data.
更多查看译文
关键词
Deep learning methods,real-time action recognition from video,human-robot collaboration
AI 理解论文
溯源树
样例
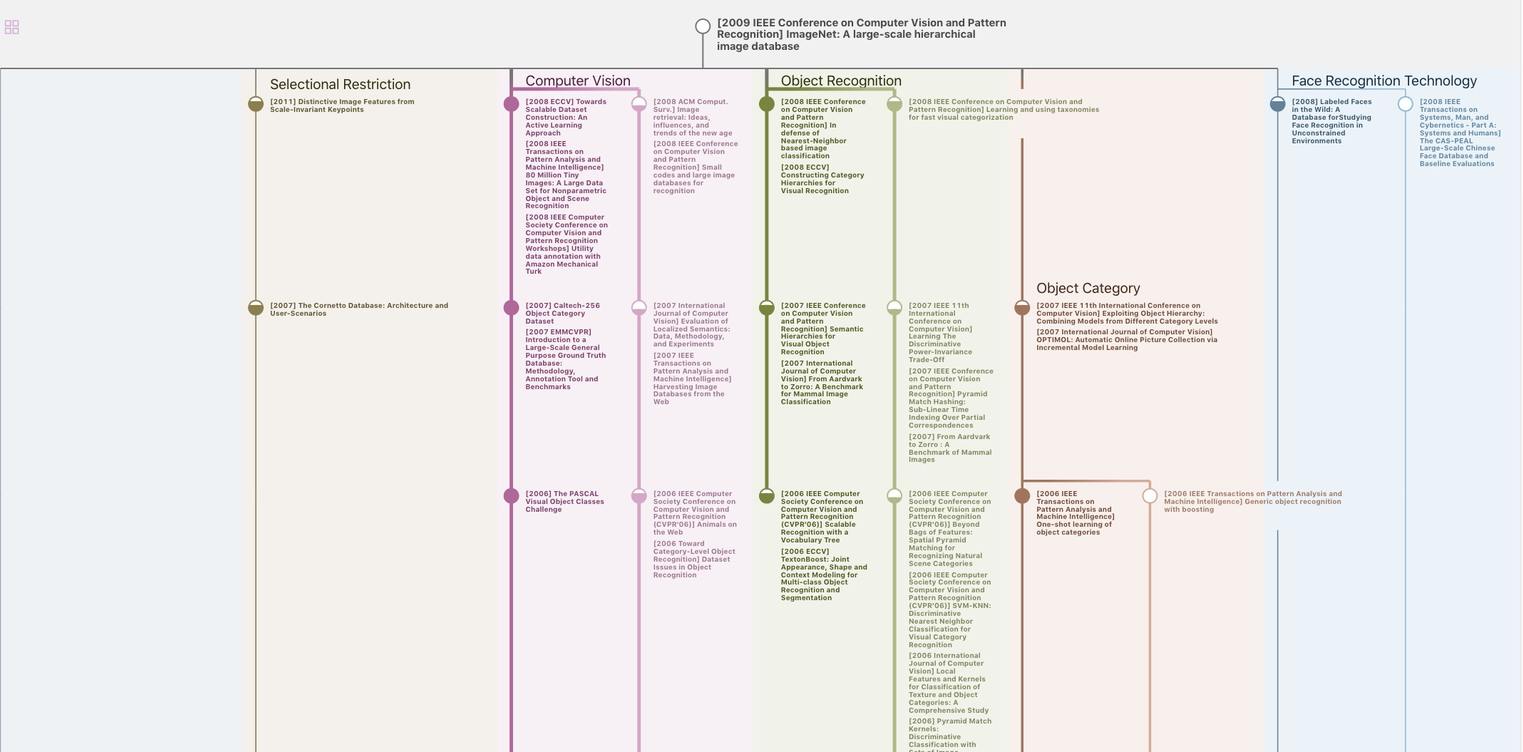
生成溯源树,研究论文发展脉络
Chat Paper
正在生成论文摘要