MMpedia: A Large-Scale Multi-modal Knowledge Graph
SEMANTIC WEB, ISWC 2023, PT II(2023)
摘要
Knowledge graphs serve as crucial resources for various applications. However, most existing knowledge graphs present symbolic knowledge in the form of natural language, lacking other modal information, e.g., images. Previous multi-modal knowledge graphs have encountered challenges with scaling and image quality. Therefore, this paper proposes a highly-scalable and high-quality multi-modal knowledge graph using a novel pipeline method. Summarily, we first retrieve images from a search engine and build a new Recurrent Gate Multimodal model to filter out the non-visual entities. Then, we utilize entities' textual and type information to remove noisy images of the remaining entities. Through this method, we construct a large-scale multi-modal knowledge graph named MMpedia, containing 2,661,941 entity nodes and 19,489,074 images. As we know, MMpedia has the largest collection of images among existing multi-modal knowledge graphs. Furthermore, we employ human evaluation and downstream tasks to verify the usefulness of images in MMpedia. The experimental result shows that both the state-of-the-art method and multi-modal large language model (e.g., VisualChatGPT) achieve about a 4% improvement on Hit@1 in the entity prediction task by incorporating our collected images. We also find that the multi-modal large language model is hard to ground entities to images. The dataset (https://zenodo.org/record/7816711) and source code of this paper are available at https://github.com/Delicate2000/ MMpedia.
更多查看译文
关键词
Multi-modal,Knowledge graph,Entity grounding
AI 理解论文
溯源树
样例
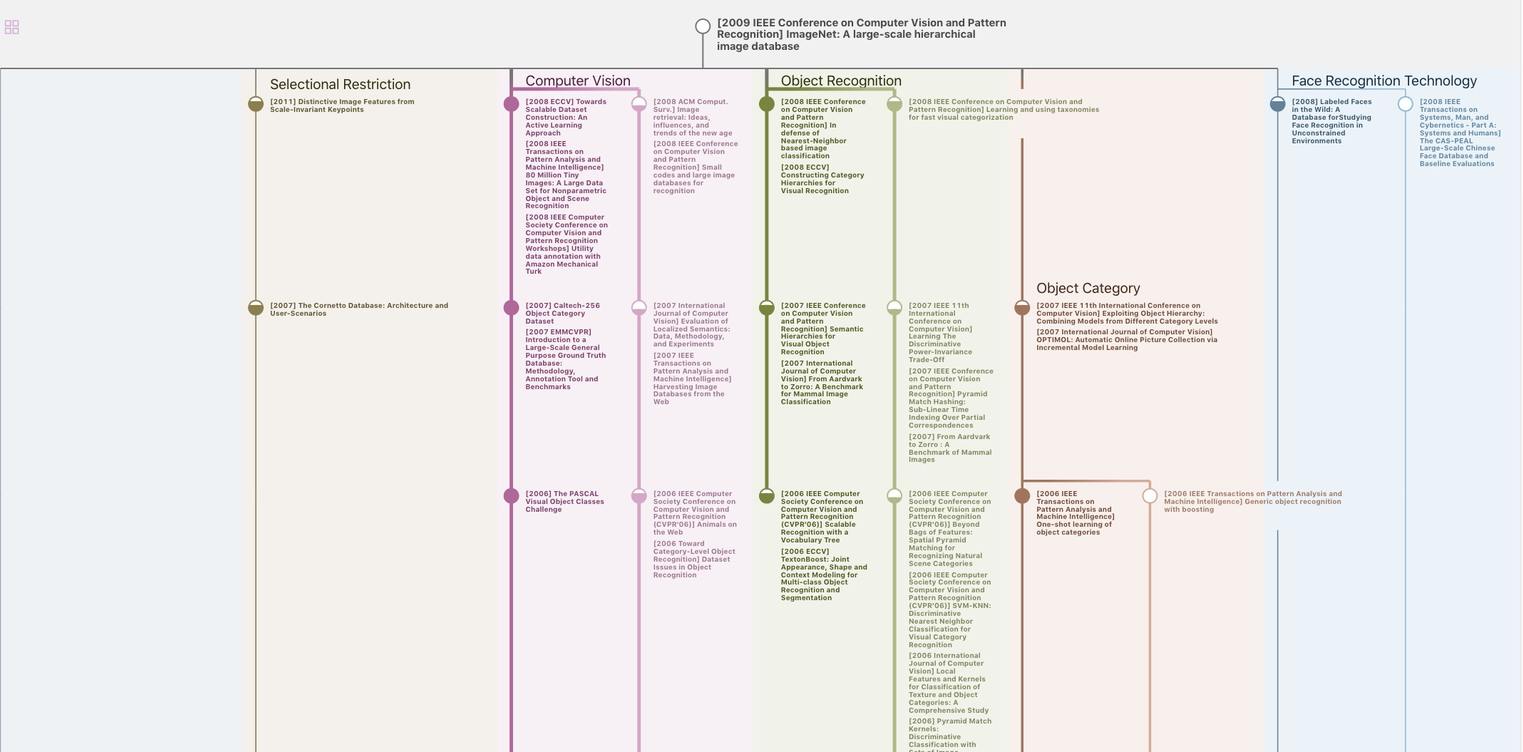
生成溯源树,研究论文发展脉络
Chat Paper
正在生成论文摘要