Causal Inference-Based Debiasing Framework for Knowledge Graph Completion
SEMANTIC WEB, ISWC 2023, PART I(2023)
摘要
The task of Knowledge Graph Completion (KGC) entails inferring missing relations and facts in a partially specified graph to discover new knowledge. However, the discrepancy in the targets between the training and inference phases might lead to in-depth bias and in-breadth bias during inference, potentially resulting in incorrect outcomes. In this work, we conduct a comprehensive analysis of these biases to determine their extent of impact. To mitigate these biases, we propose a novel debiasing framework called Causal Inference-based Debiasing Framework for KGC (CIDF) by formulating a causal graph and utilizing it for causal analysis of KGC tasks. The framework incorporates In-Depth Bias Mitigation to diminish the bias on feature representations by measuring the bias during inference, and In-Breadth Bias Mitigation to increase the distinguishability between feature representations by introducing a novel loss function. We evaluate the effectiveness of our proposed method on four benchmark datasets - WN18RR, FB15k-237, Wikidata5M-Trans, and Wikidata5M-Ind, achieving improvements of 2.5%, 0.9%, 3.2%, and 1.5% on Hit@1 respectively. Our results demonstrate that CIDF leads to significant improvements on these datasets, with more substantial gains observed in the biased settings on WN18RR achieving a 3.4% improvement in Hit@1.
更多查看译文
关键词
Knowledge Graph Completion,Causal Inference,Link Prediction
AI 理解论文
溯源树
样例
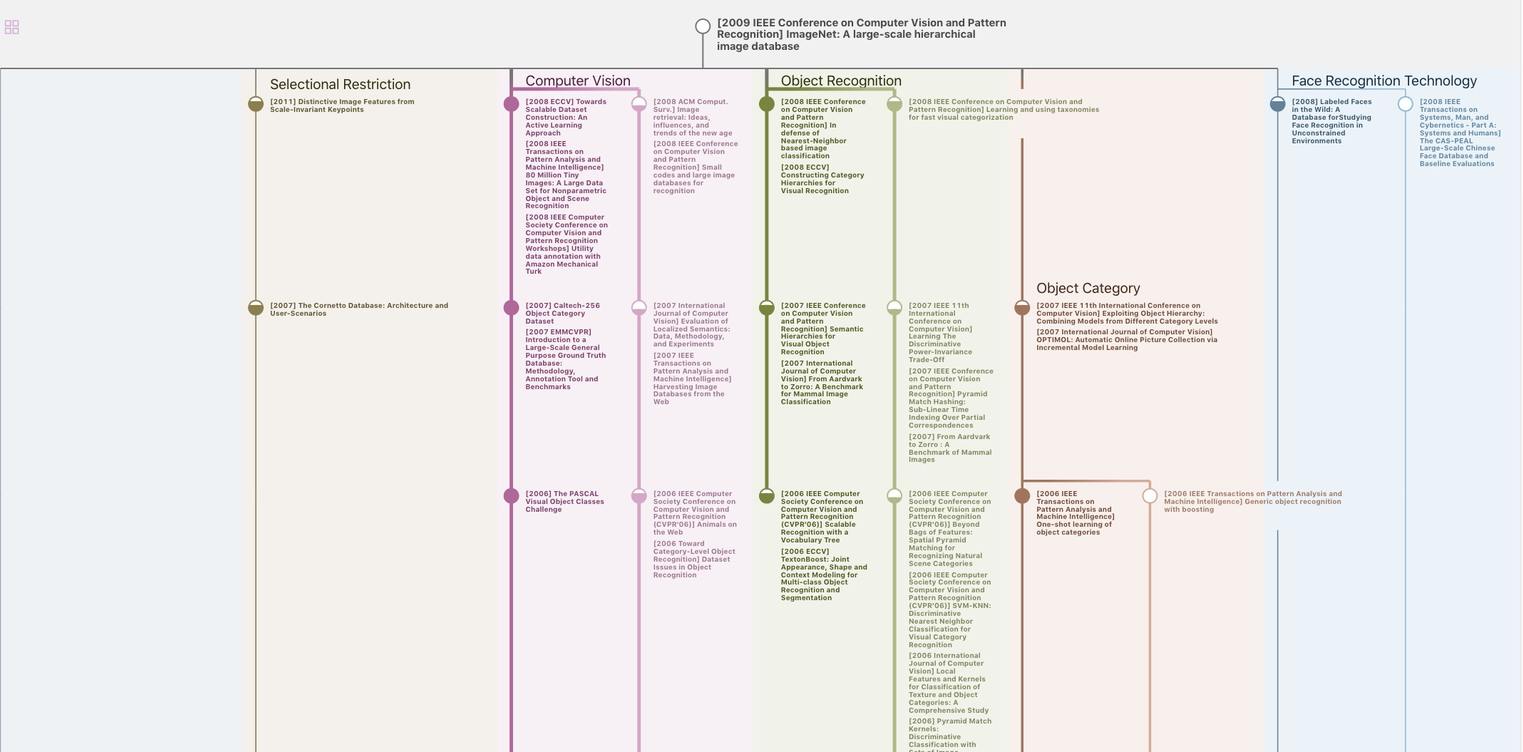
生成溯源树,研究论文发展脉络
Chat Paper
正在生成论文摘要