Flood Forecasting Using Hybrid LSTM and GRU Models with Lag Time Preprocessing
WATER(2023)
摘要
Climate change and urbanization have increased the frequency of floods worldwide, resulting in substantial casualties and property loss. Accurate flood forecasting can offer governments early warnings about impending flood disasters, giving them a chance to evacuate and save lives. Deep learning is used in flood forecasting to improve the timeliness and accuracy of flood water level predictions. While various deep learning models similar to Long Short-Term Memory (LSTM) have achieved notable results, they have complex structures with low computational efficiency, and often lack generalizability and stability. This study applies a spatiotemporal Attention Gated Recurrent Unit (STA-GRU) model for flood prediction to increase the models' computing efficiency. Another salient feature of our methodology is the incorporation of lag time during data preprocessing before the training of the model. Notably, for 12-h forecasting, the STA-GRU model's R-squared (R2) value increased from 0.8125 to 0.9215. Concurrently, the model manifested reduced root mean squared error (RMSE) and mean absolute error (MAE) metrics. For a more extended 24-h forecasting, the R2 value of the STA-GRU model improved from 0.6181 to 0.7283, accompanied by diminishing RMSE and MAE values. Seven typical deep learning models-the LSTM, the Convolutional Neural Networks LSTM (CNNLSTM), the Convolutional LSTM (ConvLSTM), the spatiotemporal Attention Long Short-Term Memory (STA-LSTM), the GRU, the Convolutional Neural Networks GRU (CNNGRU), and the STA-GRU-are compared for water level prediction. Comparative analysis delineated that the use of the STA-GRU model and the application of the lag time pre-processing method significantly improved the reliability and accuracy of flood forecasting.
更多查看译文
关键词
flood forecasting,water level prediction,STA-LSTM,STA-GRU
AI 理解论文
溯源树
样例
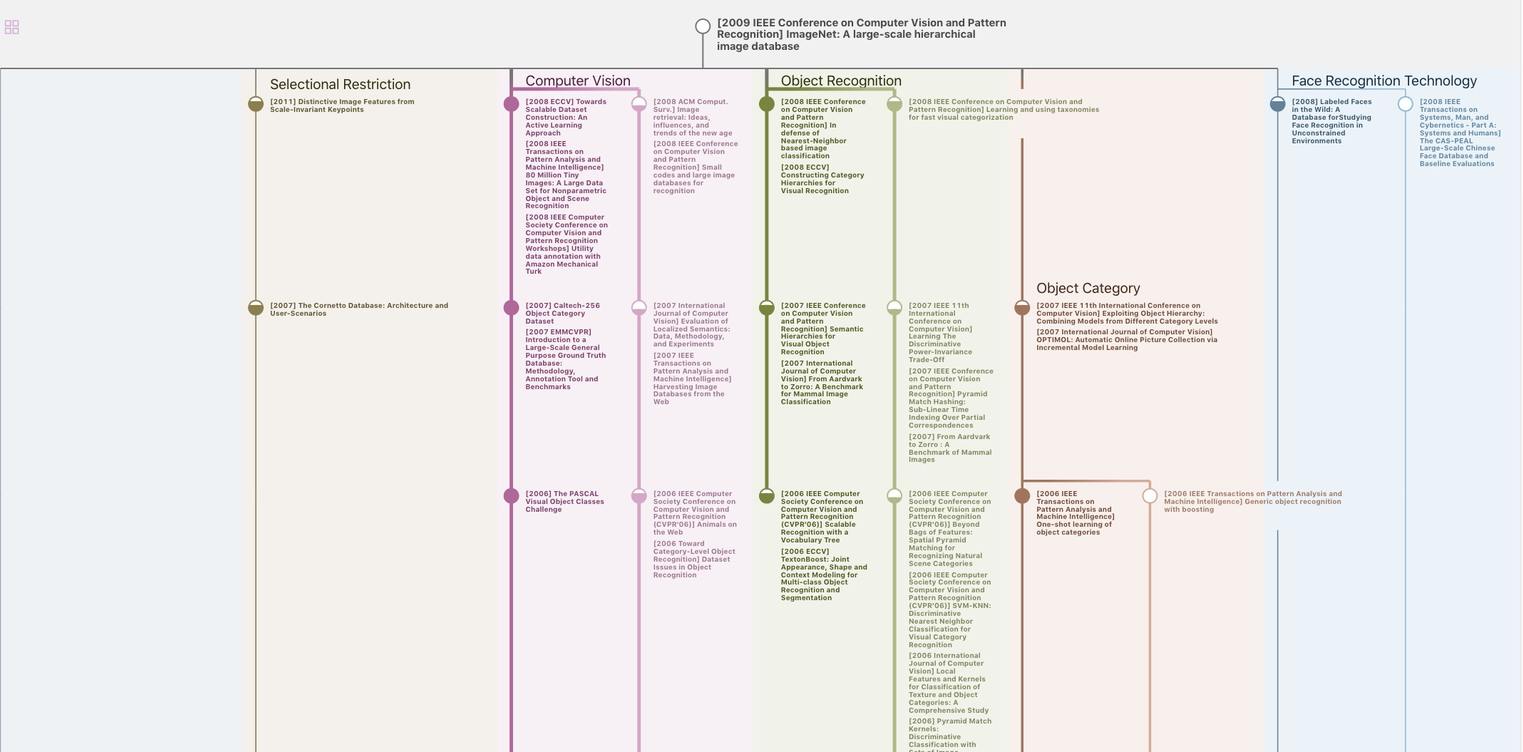
生成溯源树,研究论文发展脉络
Chat Paper
正在生成论文摘要