Boosting Ensemble Learning for Freeway Crash Classification under Varying Traffic Conditions: A Hyperparameter Optimization Approach
SUSTAINABILITY(2023)
摘要
Freeway crashes represent a significant and persistent threat to road safety, resulting in both loss of life and extensive property damage. Effectively addressing this critical issue requires a comprehensive understanding of the factors contributing to these incidents and the ability to accurately predict crash severity under different traffic conditions. This study aims to improve the accuracy of crash classification by incorporating key traffic-related variables such as braking, weather conditions, and speed. To validate the effectiveness of proposed model, we utilize real-world crash data from Flint, Michigan. To achieve the objective, we employ an innovative Boosting Ensemble Learning approach, leveraging five advanced ensemble learning models: Gradient Boosting, Cat Boost, XGBoost, LightGBM, and SGD. Through the application of hyperparameter optimization techniques, we further enhance the performance of these models, improving their predictive capabilities. Our evaluation results demonstrated the effectiveness of our approach, with Gradient Boosting algorithms achieving an accuracy rate of up to 96% in crash classification. This research provides valuable insights into the potential of using Boosting Ensemble Learning as a tool for accurately and efficiently classifying freeway crashes across a spectrum of traffic conditions. Additionally, it sheds light on the nuanced variations in crash mechanisms observed when employing diverse ensemble learning models. The findings of this study underscore the significance of hyperparameter optimization as a critical factor in elevating the predictive precision of freeway crashes.
更多查看译文
关键词
boosting ensemble learning,machine learning,Shapley Additive Explanations (SHAP),freeway crash,traffic conditions
AI 理解论文
溯源树
样例
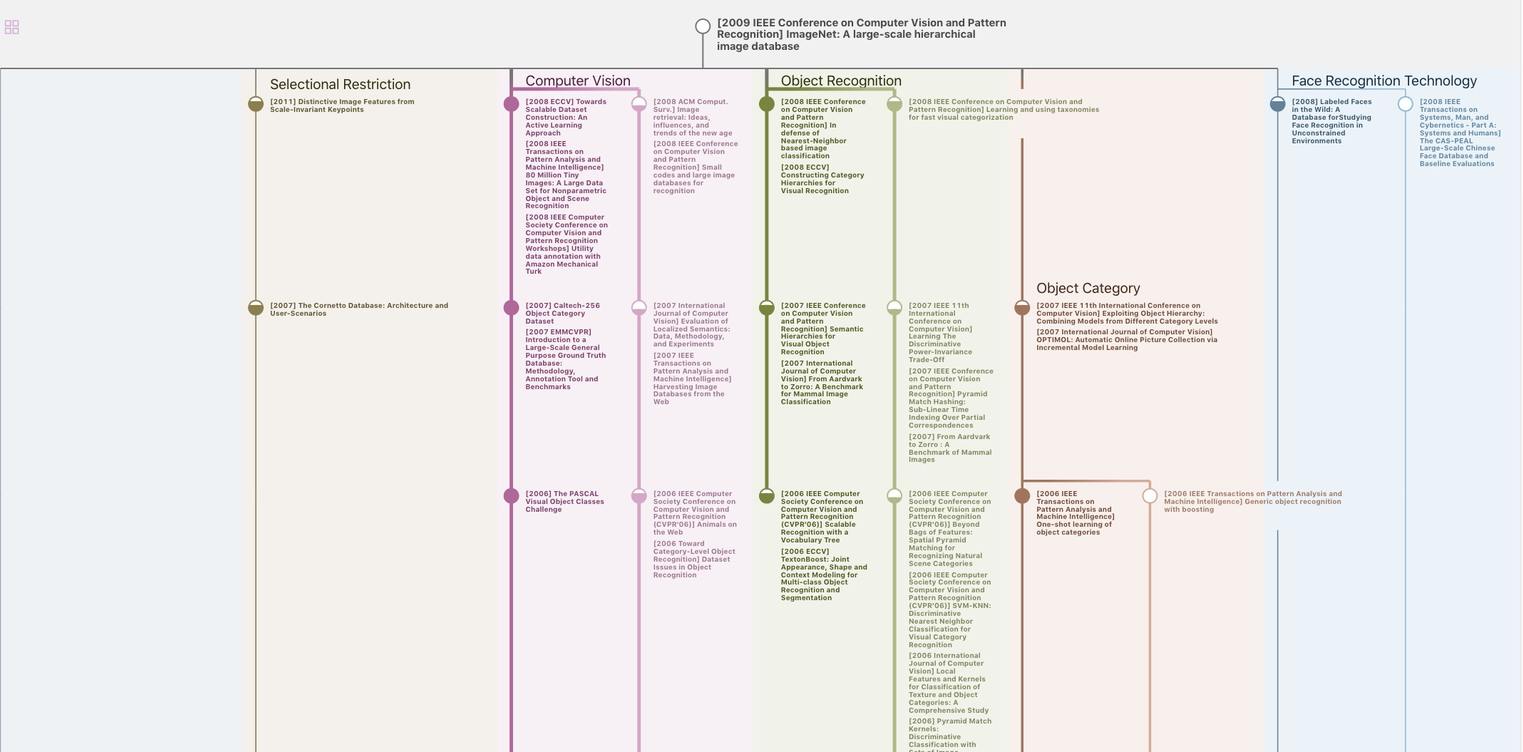
生成溯源树,研究论文发展脉络
Chat Paper
正在生成论文摘要