Critical Pattern Selection Method Based on CNN Embeddings for Full-Chip Optimization
PHOTONICS(2023)
摘要
Source mask optimization (SMO), a primary resolution enhancement technology, is one of the most pivotal technologies for enhancing lithography imaging quality. Due to the high computation complexity of SMO, patterns should be selected by a selection algorithm before optimization. However, the limitations of existing selection methods are twofold: they are computationally intensive and they produce biased selection results. The representative method having the former limitation is the diffraction signature method. And IBM's method utilizing the rigid transfer function tends to cause biased selection results. To address this problem, this study proposes a novel pattern cluster and selection algorithm architecture based on a convolutional neural network (CNN). The proposed method provides a paradigm for solving the critical pattern selection problem by CNN to transfer patterns from the source image domain to unified embeddings in a K-dimensional feature space, exhibiting higher efficiency and maintaining high accuracy.
更多查看译文
关键词
critical pattern selection method,source mask optimization,convolutional neural networks,computational lithography
AI 理解论文
溯源树
样例
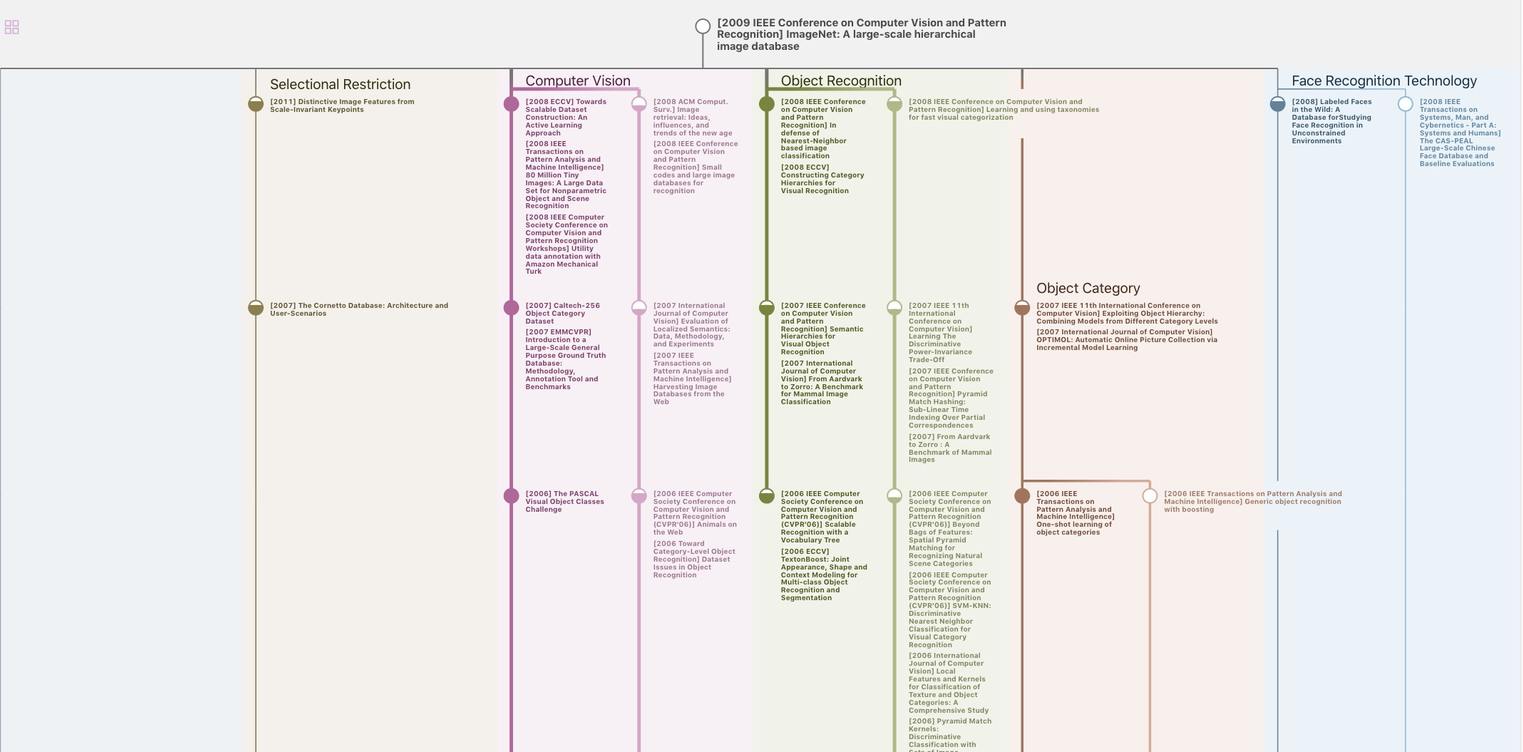
生成溯源树,研究论文发展脉络
Chat Paper
正在生成论文摘要