An Accurate Metaheuristic Mountain Gazelle Optimizer for Parameter Estimation of Single- and Double-Diode Photovoltaic Cell Models
MATHEMATICS(2023)
摘要
Accurate parameter estimation is crucial and challenging for the design and modeling of PV cells/modules. However, the high degree of non-linearity of the typical I-V characteristic further complicates this task. Consequently, significant research interest has been generated in recent years. Currently, this trend has been marked by a noteworthy acceleration, mainly due to the rise of swarm intelligence and the rapid progress of computer technology. This paper proposes a developed Mountain Gazelle Optimizer (MGO) to generate the best values of the unknown parameters of PV generation units. The MGO mimics the social life and hierarchy of mountain gazelles in the wild. The MGO was compared with well-recognized recent algorithms, which were the Grey Wolf Optimizer (GWO), the Squirrel Search Algorithm (SSA), the Differential Evolution (DE) algorithm, the Bat-Artificial Bee Colony Optimizer (BABCO), the Bat Algorithm (BA), Multiswarm Spiral Leader Particle Swarm Optimization (M-SLPSO), the Guaranteed Convergence Particle Swarm Optimization algorithm (GCPSO), Triple-Phase Teaching-Learning-Based Optimization (TPTLBO), the Criss-Cross-based Nelder-Mead simplex Gradient-Based Optimizer (CCNMGBO), the quasi-Opposition-Based Learning Whale Optimization Algorithm (OBLWOA), and the Fractional Chaotic Ensemble Particle Swarm Optimizer (FC-EPSO). The experimental findings and statistical studies proved that the MGO outperformed the competing techniques in identifying the parameters of the Single-Diode Model (SDM) and the Double-Diode Model (DDM) PV models of Photowatt-PWP201 (polycrystalline) and STM6-40/36 (monocrystalline). The RMSEs of the MGO on the SDM and the DDM of Photowatt-PWP201 and STM6-40/36 were 2.042717 x10-3, 1.387641 x10-3, 1.719946 x10-3, and 1.686104 x10-3, respectively. Overall, the identified results highlighted that the MGO-based approach featured a fast processing time and steady convergence while retaining a high level of accuracy in the achieved solution.
更多查看译文
关键词
metaheuristic algorithm,parameter identification,photovoltaic cells,single-diode,double-diode,mathematical models,mountain gazelle optimization
AI 理解论文
溯源树
样例
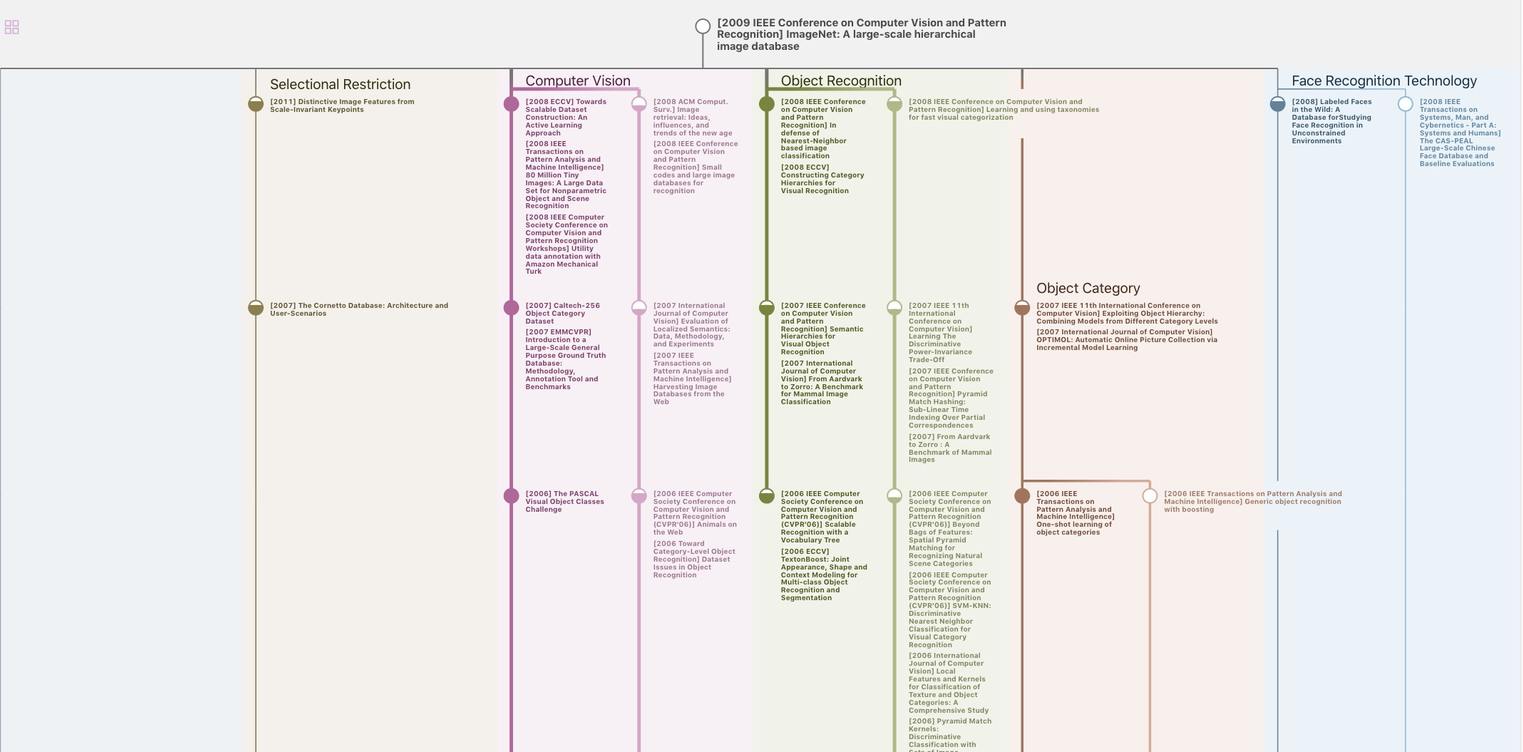
生成溯源树,研究论文发展脉络
Chat Paper
正在生成论文摘要