TLFND: A Multimodal Fusion Model Based on Three-Level Feature Matching Distance for Fake News Detection
ENTROPY(2023)
摘要
In the rapidly evolving information era, the dissemination of information has become swifter and more extensive. Fake news, in particular, spreads more rapidly and is produced at a lower cost compared to genuine news. While researchers have developed various methods for the automated detection of fake news, challenges such as the presence of multimodal information in news articles or insufficient multimodal data have hindered their detection efficacy. To address these challenges, we introduce a novel multimodal fusion model (TLFND) based on a three-level feature matching distance approach for fake news detection. TLFND comprises four core components: a two-level text feature extraction module, an image extraction and fusion module, a three-level feature matching score module, and a multimodal integrated recognition module. This model seamlessly combines two levels of text information (headline and body) and image data (multi-image fusion) within news articles. Notably, we introduce the Chebyshev distance metric for the first time to calculate matching scores among these three modalities. Additionally, we design an adaptive evolutionary algorithm for computing the loss functions of the four model components. Our comprehensive experiments on three real-world publicly available datasets validate the effectiveness of our proposed model, with remarkable improvements demonstrated across all four evaluation metrics for the PolitiFact, GossipCop, and Twitter datasets, resulting in an F1 score increase of 6.6%, 2.9%, and 2.3%, respectively.
更多查看译文
关键词
fake news detection,deep learning,matching distance,multimodal integrated detection
AI 理解论文
溯源树
样例
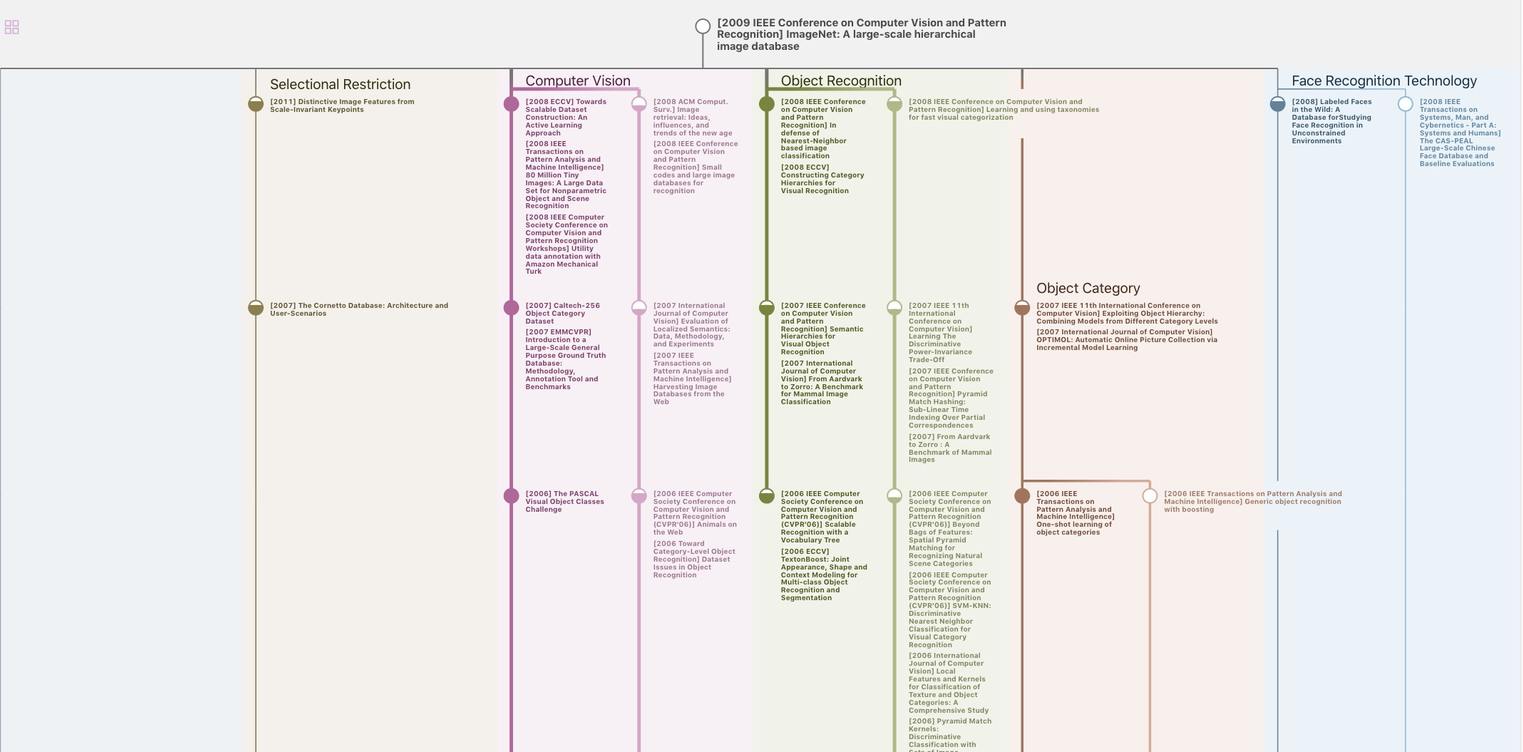
生成溯源树,研究论文发展脉络
Chat Paper
正在生成论文摘要