Analysis of Training Data Augmentation for Diabetic Foot Ulcer Semantic Segmentation
Electronics(2023)
摘要
Deep learning model training and achieved performance relies on available data. Diabetic foot ulcers and other image processing applications in the medical domain add another layer of complexity to training data collection. Data collection is troublesome and data annotation requires medical expertise. This problem is usually solved by employing training data augmentation. Although in previous research augmentation was facilitated in various ways, it is rarely evaluated or reported how much it contributes to achieved performance. The current research seeks to answer this question by applying individual photometric and geometric augmentation techniques and comparing the model performance achieved for semantic segmentation of diabetic foot ulcers. It was found that geometric augmentation techniques help achieve a better model performance when compared with photometric techniques. The model trained using an augmented dataset and applying a shear technique was found to improve segmentation results the most; the benchmark dice score was increased by 6%. An additional improvement over the benchmark was observed (a total of 6.9%) when the model was trained using data combining image sets generated by the three best-performing augmentation techniques. The highest test dice score achieved was 91%.
更多查看译文
关键词
augmentation,diabetic foot ulcer,semantic segmentation,deep learning
AI 理解论文
溯源树
样例
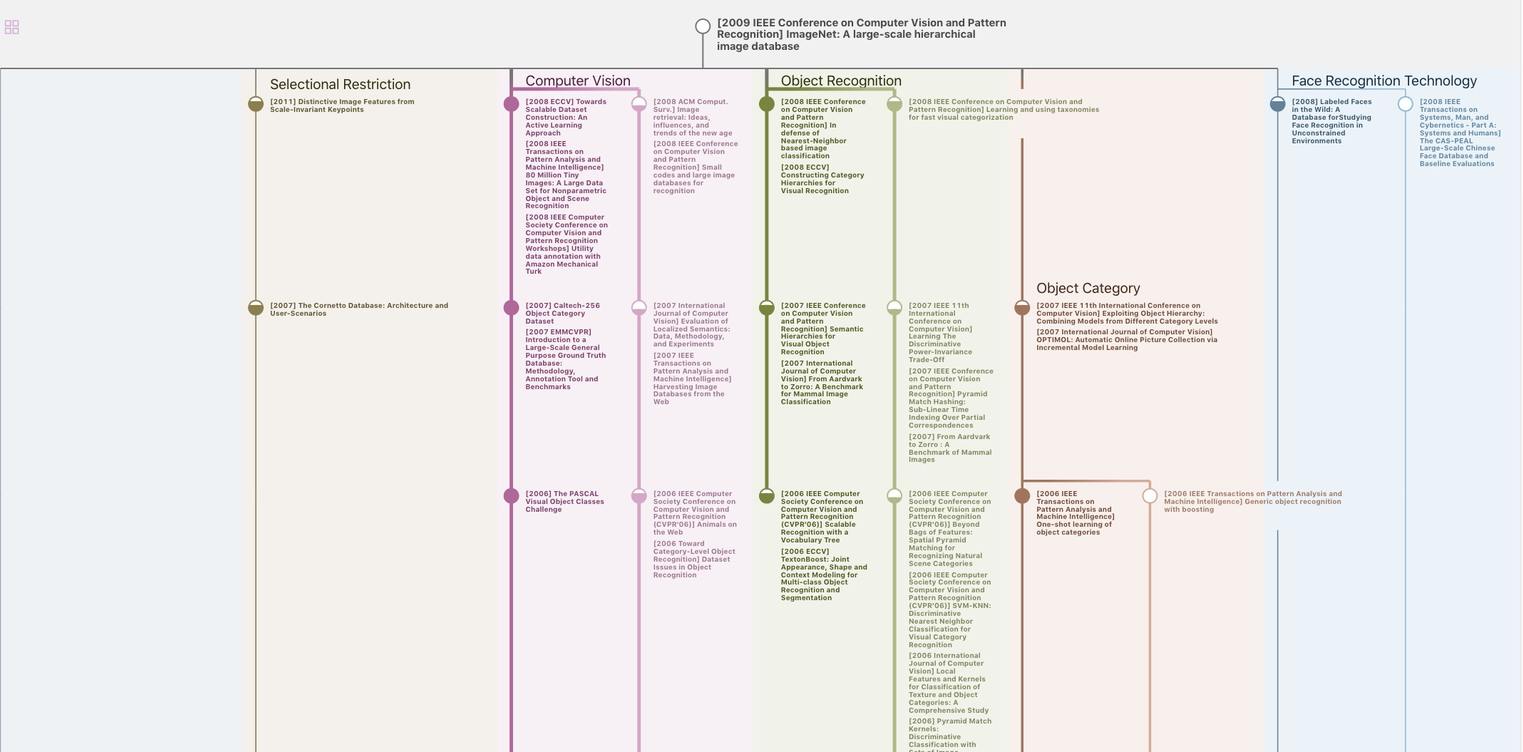
生成溯源树,研究论文发展脉络
Chat Paper
正在生成论文摘要