ADEQ: Adaptive Diversity Enhancement for Zero-Shot Quantization
NEURAL INFORMATION PROCESSING, ICONIP 2023, PT I(2024)
摘要
Zero-shot quantization (ZSQ) is an effective way to compress neural networks, especially when real training sets are inaccessible because of privacy and security issues. Most existing synthetic-data-driven zero-shot quantization methods introduce diversity enhancement to simulate the distribution of real samples. However, the adaptivity between the enhancement degree and network is neglected, i.e., whether the enhancement degree benefits different network layers and different classes, and whether it reaches the best match between the inter-class distance and intra-class diversity. Due to the absence of the metric for class-wise and layer-wise diversity, maladaptive enhancement degree run the vulnerability of mode collapse of the inter-class inseparability. To address this issue, we propose a novel zero-shot quantization method, ADEQ. For layer-wise and class-wise adaptivity, the enhancement degree of different layers is adaptively initialized with a diversity coefficient. For inter-class adaptivity, an incremental diversity enhancement strategy is proposed to achieve the trade-off between inter-class distance and intra-class diversity. Extensive experiments on the CIFAR-100 and ImageNet show that our ADEQ is observed to have advanced performance at low bit-width quantization. For example, when ResNet-18 is quantized to 3 bits, we improve top-1 accuracy by 17.78% on ImageNet compared to the advanced ARC. Code at https://github.com/dangsingrue/ADEQ.
更多查看译文
关键词
Zero-shot Quantization,Diversity Enhancement,Class-wise Adaptability,Layer-wise Adaptability,Inter-class Separability
AI 理解论文
溯源树
样例
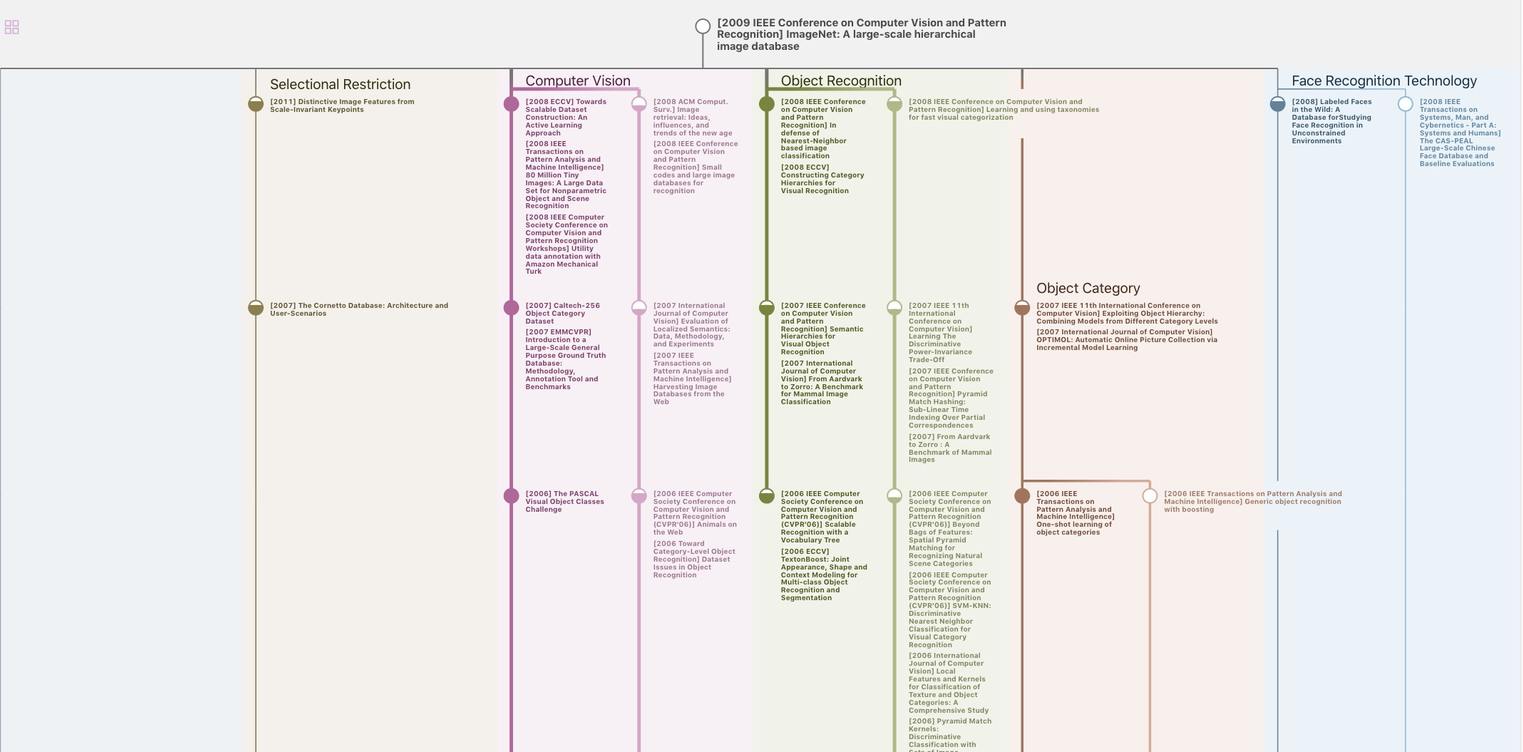
生成溯源树,研究论文发展脉络
Chat Paper
正在生成论文摘要