Extracting Knowledge from Incompletely Known Models.
Intelligent Data Engineering and Automated Learning – IDEAL 2023: 24th International Conference, Évora, Portugal, November 22–24, 2023, Proceedings(2023)
摘要
In the age of Artificial Intelligence (AI), the use of trained models is a common practice to solve a huge amount of different problems. However, it is highly complicated to understand the decision-making process of these models and how the used training data affect them during the production phase. For this reason, multiple techniques related to model extraction have appeared. These techniques consist of analyzing the behavior of a (sometimes partially) unknown model and generating a clone that reacts similarly. This process is relatively simple with basic models, but it becomes arduous when complex models must be analyzed and replicated. This paper tackles this issue by presenting the Neural NetwOrk Models (VENNOM) system. It is a general framework architecture to extract knowledge and provide explainability to unknown models. The proposed approach uses low-capacity, high-explainability neural networks to produce flexible and interpretable models. The proposed framework offers several advantages, particularly in terms of obtaining explanations through visual and textual content for models that are otherwise opaque. The framework has been tested on tabular data sets, demonstrating its performance and potential.
更多查看译文
关键词
known models,knowledge
AI 理解论文
溯源树
样例
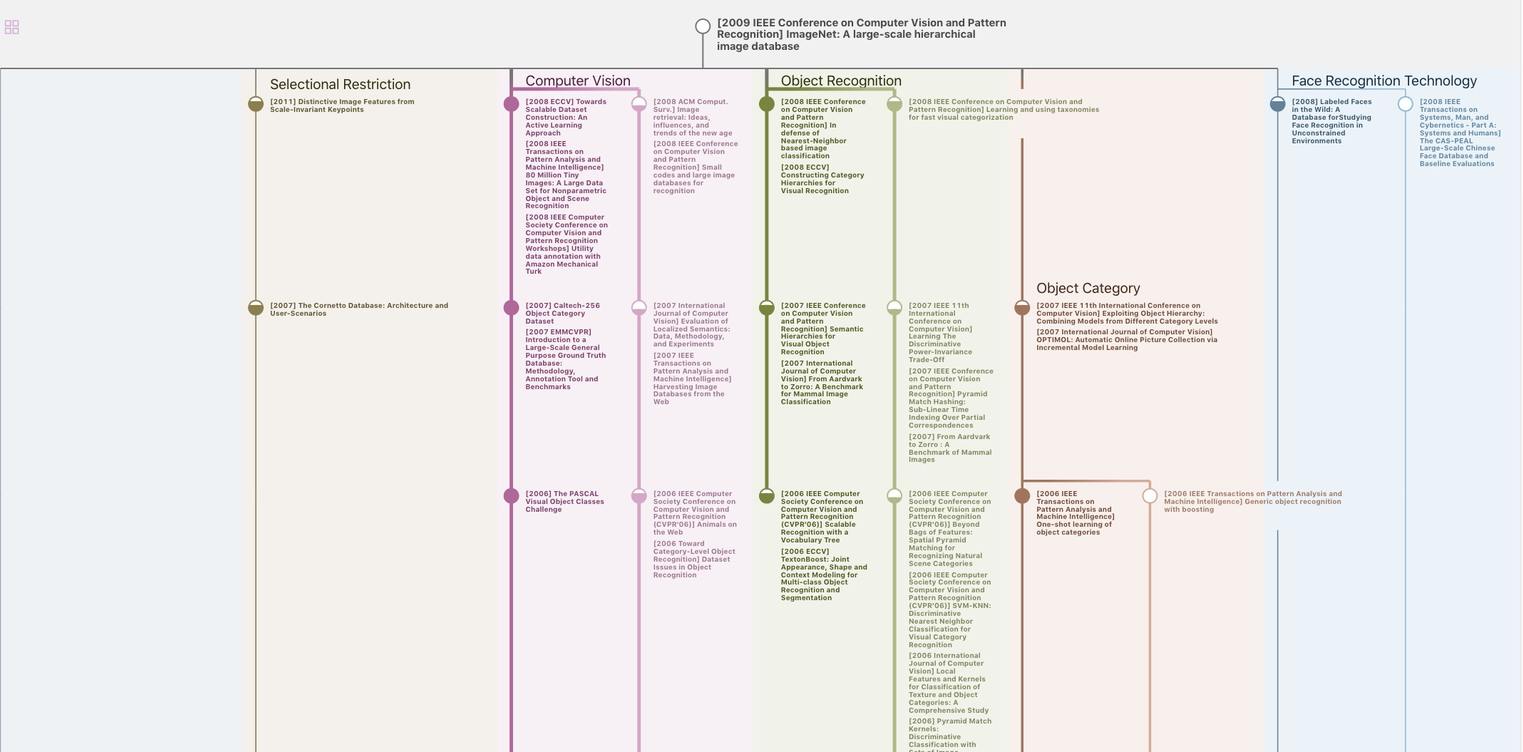
生成溯源树,研究论文发展脉络
Chat Paper
正在生成论文摘要