Vessel Behavior Anomaly Detection Using Graph Attention Network
NEURAL INFORMATION PROCESSING, ICONIP 2023, PT V(2024)
摘要
Vessel behavior anomaly detection is of great significance for ensuring navigation safety, combating maritime crimes, and maritime management. Unfortunately, most current researches ignore the temporal dependencies and correlations between ship features. We propose a novel vessel behavior anomaly detection using graph attention network (i.e., VBAD-GAT) framework, which characterizes these complicated relationships and dependencies through a graph attention module that consists of a time graph attention module and a feature graph attention module. We also adopt a process of graph structure learning to obtain the correct feature graph structure. Moreover, we propose a joint detection strategy combining reconstruction and prediction modules to capture the local ship features and long-term relationships between ship features. We demonstrate the effectiveness of the graph attention module and the joint detection strategy through the ablation study. In addition, the comparative experiments with three baselines, including the quantitative analysis and visualization, show that VBAD-GAT outperforms all other baselines.
更多查看译文
关键词
Anomaly detection,Graph attention network,AIS data mining
AI 理解论文
溯源树
样例
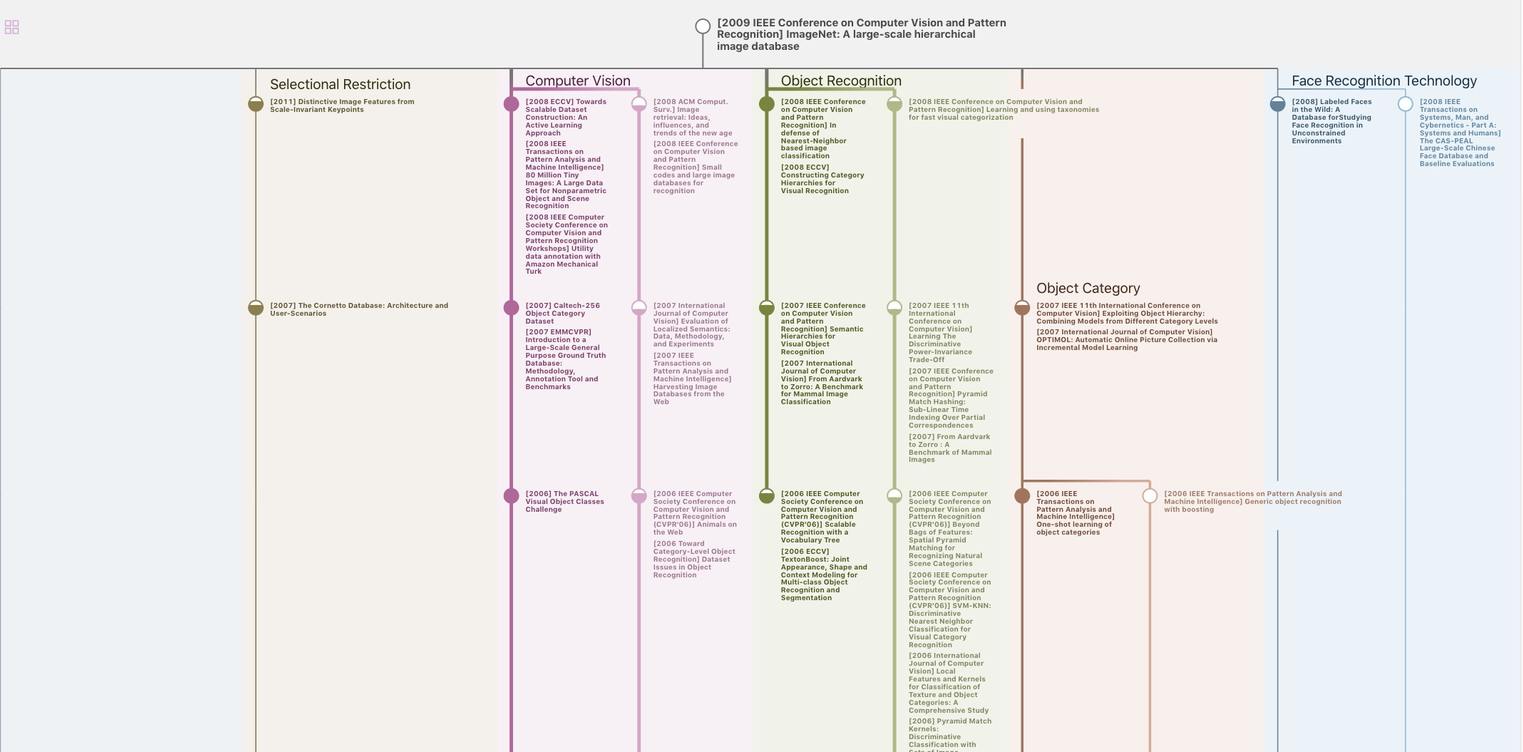
生成溯源树,研究论文发展脉络
Chat Paper
正在生成论文摘要