Enabling Feedback-Free MIMO Transmission for FD-RAN: A Data-driven Approach.
CoRR(2023)
Abstract
To enhance flexibility and facilitate resource cooperation, a novel fully-decoupled radio access network (FD-RAN) architecture is proposed for 6G. However, the decoupling of uplink (UL) and downlink (DL) in FD-RAN makes the existing feedback mechanism ineffective. To this end, we propose an end-to-end data-driven MIMO solution without the conventional channel feedback procedure. Data-driven MIMO can alleviate the drawbacks of feedback including overheads and delay, and can provide customized precoding design for different BSs based on their historical channel data. It essentially learns a mapping from geolocation to MIMO transmission parameters. We first present a codebook-based approach, which selects transmission parameters from the statistics of discrete channel state information (CSI) values and utilizes integer interpolation for spatial inference. We further present a non-codebook-based approach, which 1) derives the optimal precoder from the singular value decomposition (SVD) of the channel; 2) utilizes variational autoencoder (VAE) to select the representative precoder from the latent Gaussian representations; and 3) exploits Gaussian process regression (GPR) to predict unknown precoders in the space domain. Extensive simulations are performed on a link-level 5G simulator using realistic ray-tracing channel data. The results demonstrate the effectiveness of data-driven MIMO, showcasing its potential for application in FD-RAN and 6G.
MoreTranslated text
AI Read Science
Must-Reading Tree
Example
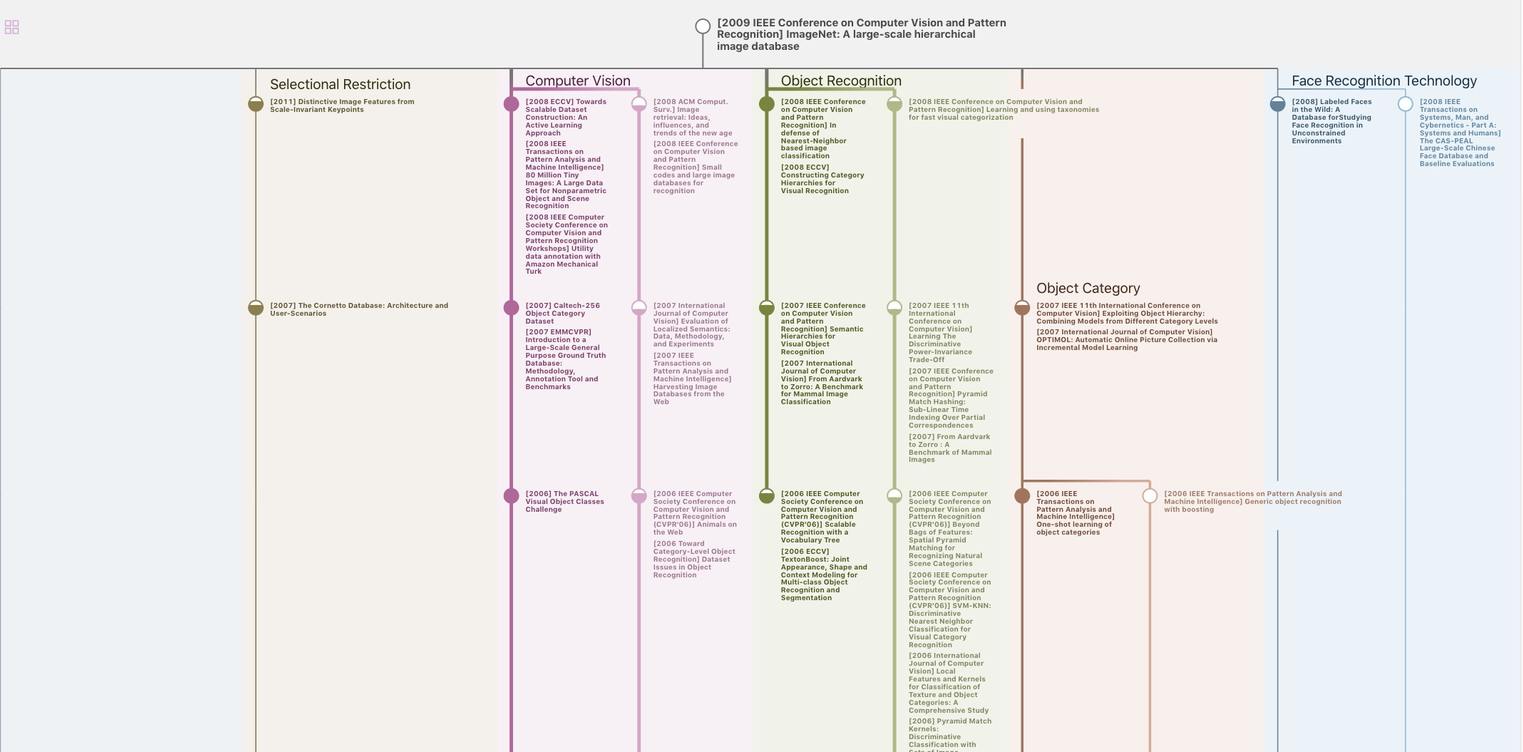
Generate MRT to find the research sequence of this paper
Chat Paper
Summary is being generated by the instructions you defined