Densely connected convolutional networks for ultrasound image based lesion segmentation
COMPUTERS IN BIOLOGY AND MEDICINE(2024)
摘要
Delineating lesion boundaries play a central role in diagnosing thyroid and breast cancers, making related therapy plans and evaluating therapeutic effects. However, it is often time-consuming and error-prone with limited reproducibility to manually annotate low-quality ultrasound (US) images, given high speckle noises, heterogeneous appearances, ambiguous boundaries etc., especially for nodular lesions with huge intra-class variance. It is hence appreciative but challenging for accurate lesion segmentations from US images in clinical practices. In this study, we propose a new densely connected convolutional network (called MDenseNet) architecture to automatically segment nodular lesions from 2D US images, which is first pre-trained over ImageNet database (called PMDenseNet) and then retrained upon the given US image datasets. Moreover, we also designed a deep MDenseNet with pre-training strategy (PDMDenseNet) for segmentation of thyroid and breast nodules by adding a dense block to increase the depth of our MDenseNet. Extensive experiments demonstrate that the proposed MDenseNet-based method can accurately extract multiple nodular lesions, with even complex shapes, from input thyroid and breast US images. Moreover, additional experiments show that the introduced MDenseNet-based method also outperforms three state-of-the-art convolutional neural networks in terms of accuracy and reproducibility. Meanwhile, promising results in nodular lesion segmentation from thyroid and breast US images illustrate its great potential in many other clinical segmentation tasks.
更多查看译文
关键词
Nodular lesion,Ultrasound image,Densely connected convolutional network,Pre-training,Segmentation
AI 理解论文
溯源树
样例
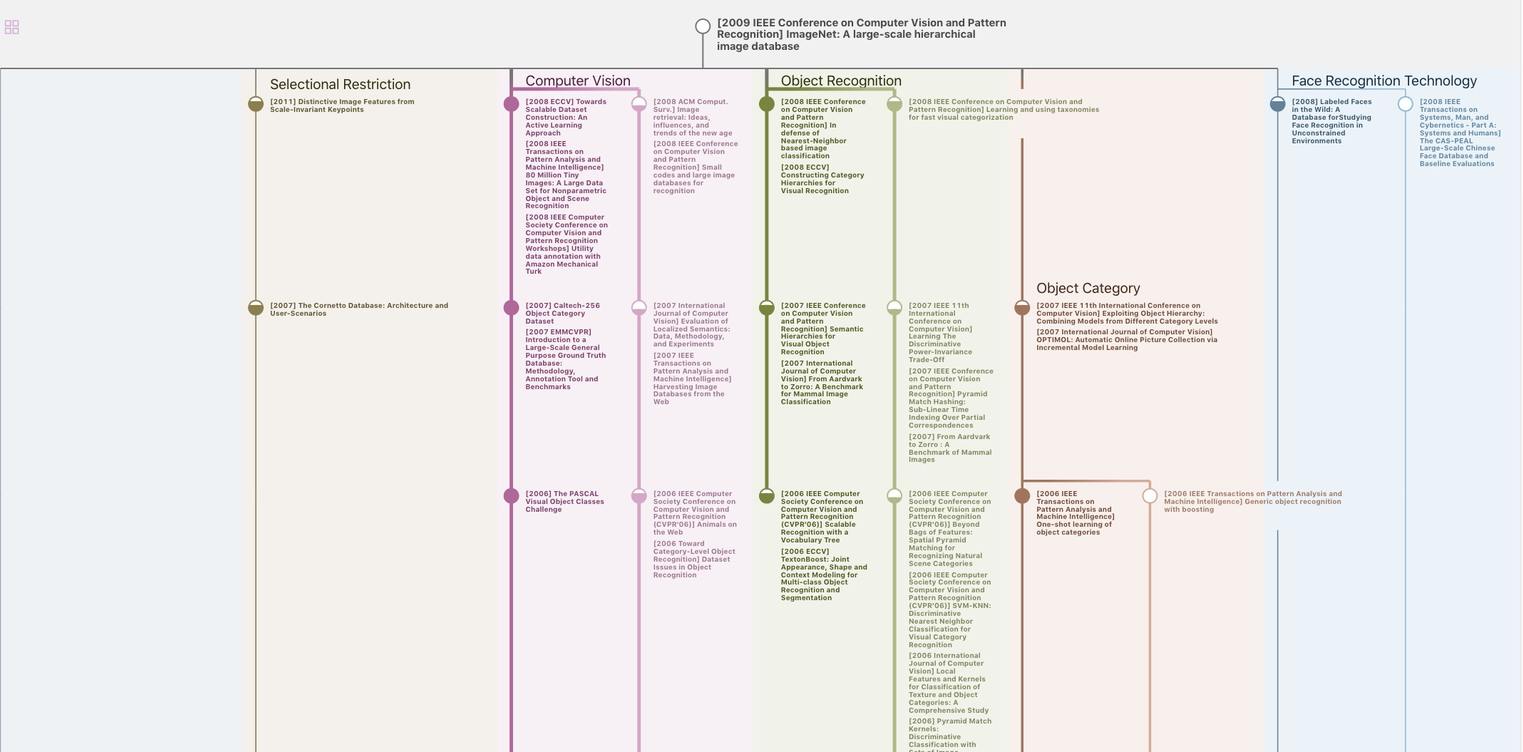
生成溯源树,研究论文发展脉络
Chat Paper
正在生成论文摘要