Non-intrusive, transferable model for coupled turbulent channel-porous media flow based upon neural networks
arxiv(2023)
摘要
Turbulent flow over permeable interface is omnipresent featuring complex flow topology. In this work, a data driven, end to end machine learning model has been developed to model the turbulent flow in porous media. For the same, we have derived a non linear reduced order model with a deep convolution autoencoder network. This model can reduce highly resolved spatial dimensions, which is a prerequisite for direct numerical simulation. A downstream recurrent neural network has been trained to capture the temporal trend of reduced modes, thus it is able to provide future evolution of modes. We further evaluate the trained model s capability on a newer dataset with a different porosity. In such cases, fine tuning could reduce the efforts (up to two order of magnitude) to train a model with limited dataset and knowledge and still show a good agreement on the mean velocity profile. Leveraging the current model, we find that even quick fine tuning achieving an impressive order of magnitude reduction in training time by approximately still results in effective flow predictions. This promising discovery encourages the fast development of a substantial amount of data-driven models tailored for various types of porous media. The diminished training time substantially lowers the computational cost when dealing with changing porous topologies, making it feasible to systematically explore interface engineering with different types of porous media. Overall, the data driven model shows a good agreement, especially for the porous media which can aid the DNS and reduce the burden to resolve this complex domain during the simulations. The fine tuning is able to reduce the training cost significantly and maintain an acceptable accuracy when a new flow condition comes into play.
更多查看译文
AI 理解论文
溯源树
样例
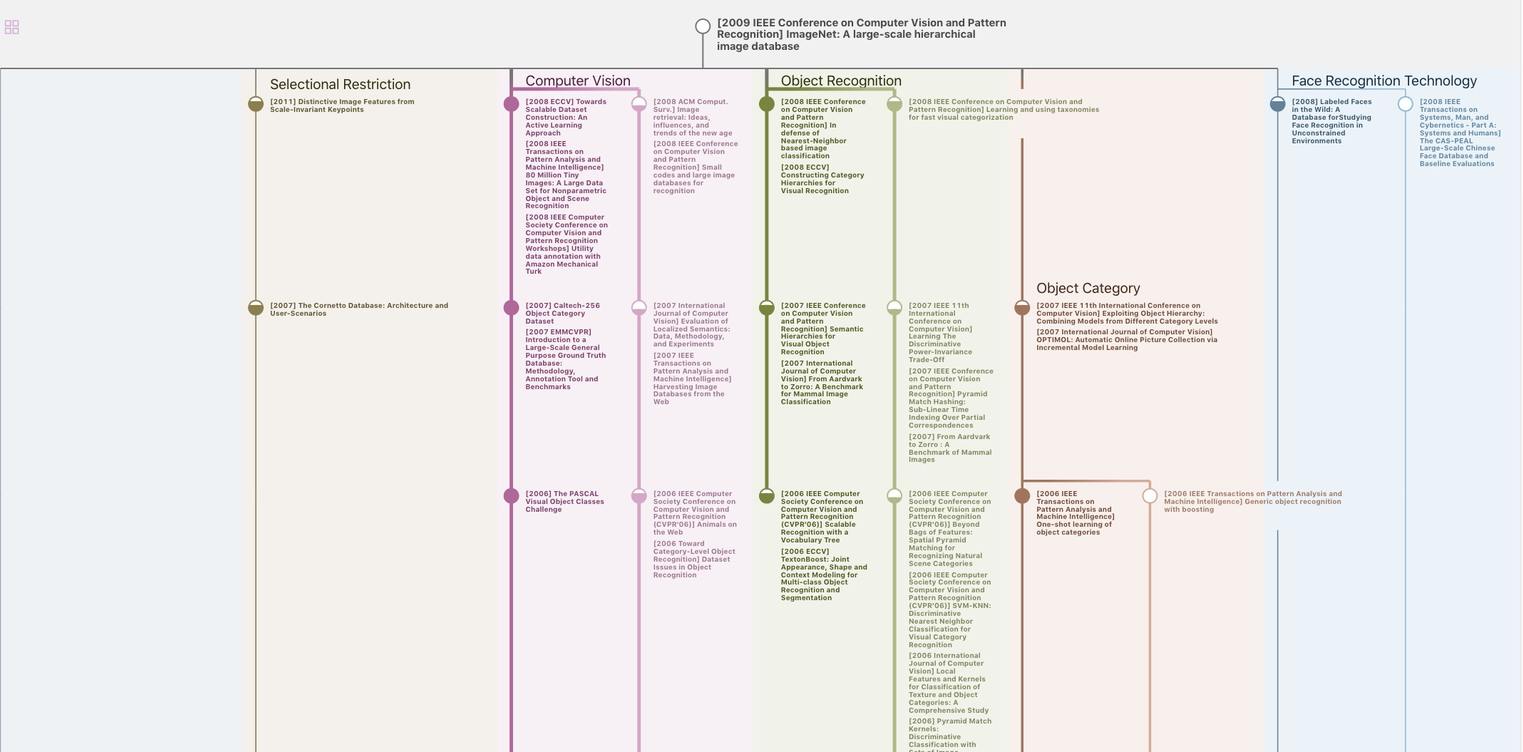
生成溯源树,研究论文发展脉络
Chat Paper
正在生成论文摘要