Bayesian Approach to Linear Bayesian Networks.
CoRR(2023)
摘要
This study proposes the first Bayesian approach for learning high-dimensional linear Bayesian networks. The proposed approach iteratively estimates each element of the topological ordering from backward and its parent using the inverse of a partial covariance matrix. The proposed method successfully recovers the underlying structure when Bayesian regularization for the inverse covariance matrix with unequal shrinkage is applied. Specifically, it shows that the number of samples $n = \Omega( d_M^2 \log p)$ and $n = \Omega(d_M^2 p^{2/m})$ are sufficient for the proposed algorithm to learn linear Bayesian networks with sub-Gaussian and 4m-th bounded-moment error distributions, respectively, where $p$ is the number of nodes and $d_M$ is the maximum degree of the moralized graph. The theoretical findings are supported by extensive simulation studies including real data analysis. Furthermore the proposed method is demonstrated to outperform state-of-the-art frequentist approaches, such as the BHLSM, LISTEN, and TD algorithms in synthetic data.
更多查看译文
AI 理解论文
溯源树
样例
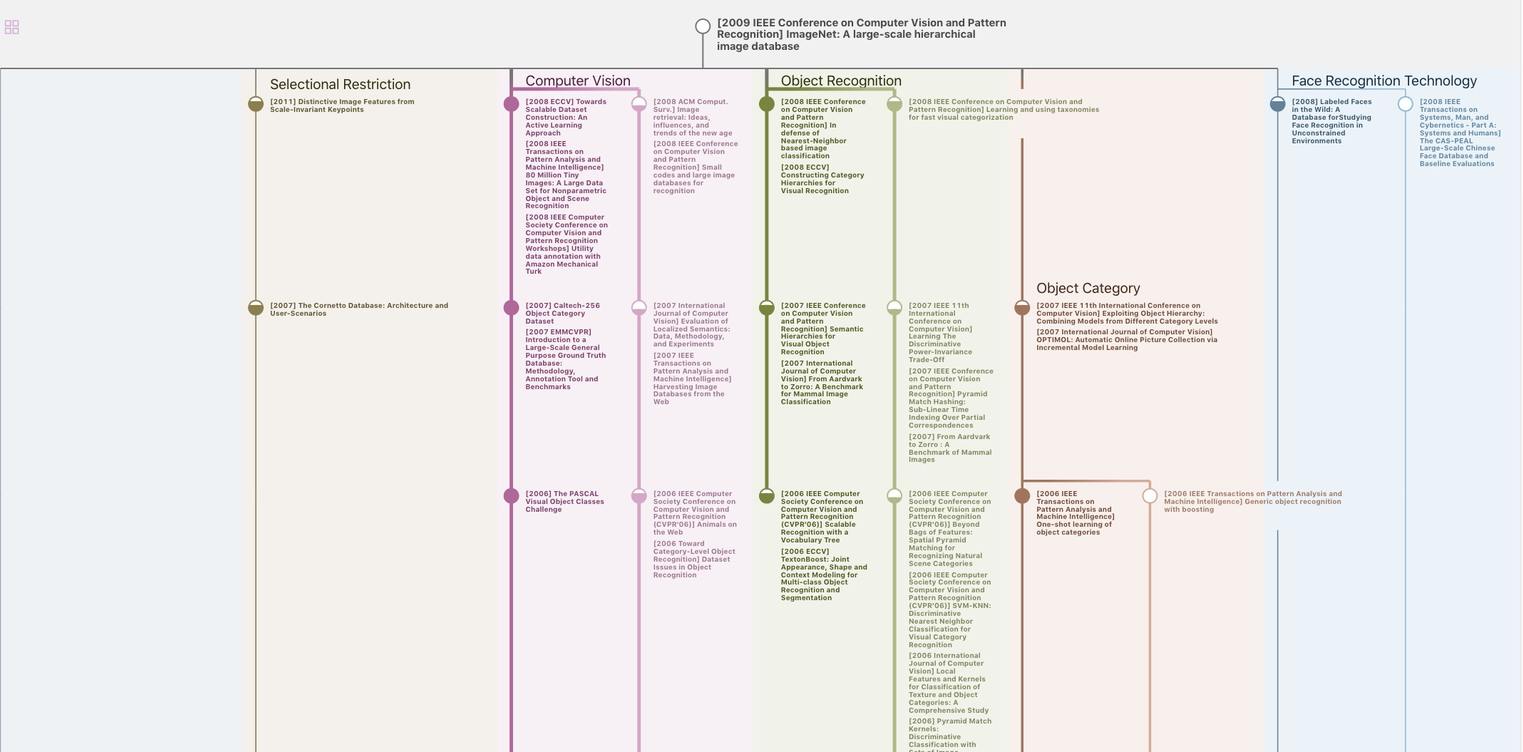
生成溯源树,研究论文发展脉络
Chat Paper
正在生成论文摘要