Prediction of Multiple Components of Secondary Ash in Blast Furnace Based on Firefly Algorithm Optimized LSTM
2023 5th International Conference on Industrial Artificial Intelligence (IAI)(2023)
摘要
Blast furnace ironmaking is a critical step in the development of heavy industry in urban areas. When multiple types of ash are generated and need to be reused, it is necessary to re-proportion them for proper utilization. However, due to practical limitations, material component content cannot achieve real-time detection, making ash re-proportioning a challenging task. To address this issue, we propose a Long Short-Term Memory Neural Network (LSTM) with sliding windows to predict the composition of blast furnace secondary ash that has not been tested. By utilizing the temporal nature of the data, a sliding window partitions the dataset, and Firefly Algorithm (FA) is employed to optimize the window size. The model is then trained, and multiple component content predictions are generated. The model data is derived from the actual two-year inspection and testing data of a steel and iron plant in China. The experimental results show that the predicted results of the model are close to the true values, confirming the feasibility of the FA-LSTM model with sliding windows to accurately predict the material composition. This study provides computer technology and means to support the utilization of ash, which has high application value. Furthermore, it offers data support for the input of material volume during workers’ work, which can help solve proportion issues in the ash cycle.
更多查看译文
关键词
multi-component prediction,sliding window,firefly algorithm,LSTM
AI 理解论文
溯源树
样例
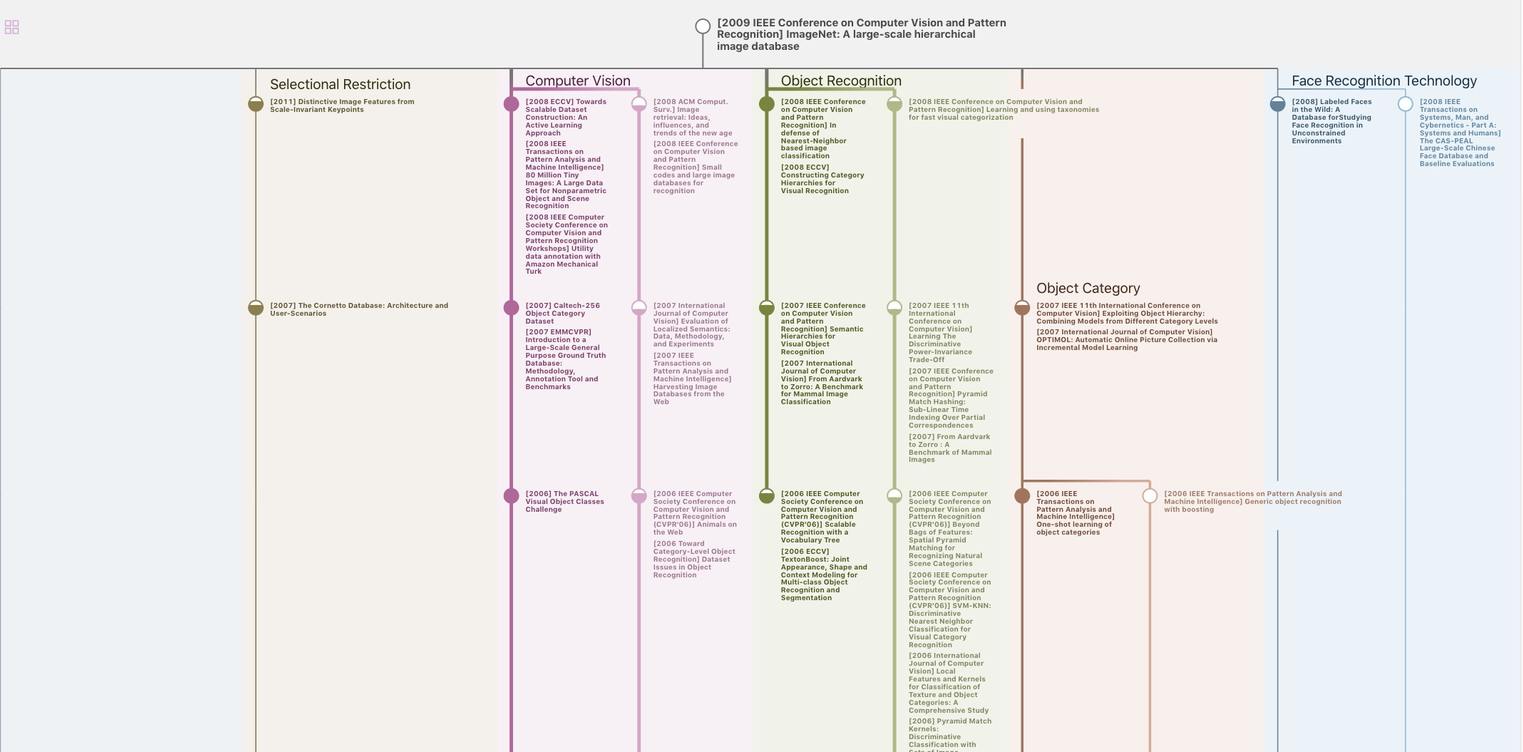
生成溯源树,研究论文发展脉络
Chat Paper
正在生成论文摘要