Sparse Identification of Nonlinear Dynamics via an Sequential Thresholded-Iteratively Reweighted Least Squares
2023 5th International Conference on Industrial Artificial Intelligence (IAI)(2023)
Abstract
This work proposes a sequential thresholded re-weighted ℓ
2
sparse regression method to learn the ordinary differential equations (ODEs) for nonlinear dynamical systems from time series data with noise. The method leverages the sparse identification of nonlinear dynamics (SINDy) framework developed in [1] to first construct a library of candidate terms for representing the nonlinear dynamic of ODEs and then use the sparse model selection to determine the active feature terms occurring in ODEs. This work aims at using more less measurements to robustly discover parsimonious ODEs with descriptive ability from noisy data. For this, an iterative re-weighted least square algorithm is used to promote the sparsity while reducing the measurements. We also place a thresholding step into the iterative re-weighted ℓ
2
least squares process, wherein a threshold parameter is set up to clean up possible disturbances occurring in the weight and re-estimate the weight using the remaining terms, iteratively until convergence. With several classical ODEs, we demonstrate the accuracy and robustness of the proposed method for inferring ODEs from noisy data and compare it with the original iterative re-weighted ℓ
2
least squares without the thresholding step.
MoreTranslated text
Key words
Ordinary differential equation,sequential threshold re-weighted least squares,sparse regression
AI Read Science
Must-Reading Tree
Example
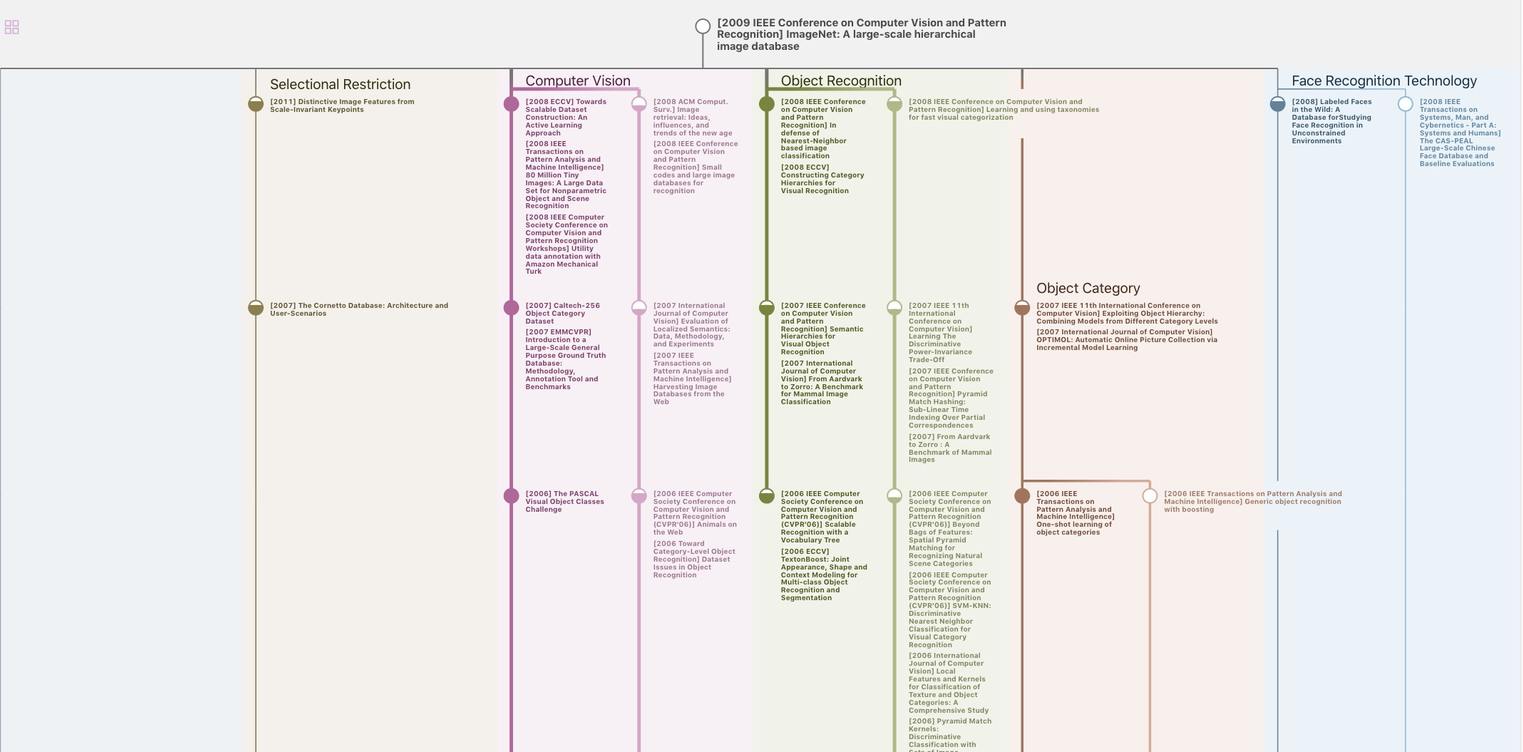
Generate MRT to find the research sequence of this paper
Chat Paper
Summary is being generated by the instructions you defined