An Underwater Single Target Tracking Method Using SiamRPN++ Based on Inverted Residual Bottleneck Block
IEEE Access(2021)
摘要
Traditional tracking algorithms reply on manually extracted features while deep learning algorithms can automatically extract features, which is of great importance for single target tracking in the complex underwater environment that is characterized by poor visibility, low contrasts, and occlusion. The convolutional neural network SiamRPN++ is an advanced deep learning algorithm whose backbone network structure is. However, this algorithm is difficult to be implemented in an underwater platform due to its large amount of computation and high requirement for the computing power of the hardware. To solve this problem, a new backbone network structure NewNet-62 characterized by inverted residual bottleneck block and the SiamRPN++ algorithm are proposed. The depth-wise separable convolution in NewNet-62 simplifies the computation so that the SiamRPN++ algorithm can realize fast and accurate tracking of underwater single targets. The video set in VOT2016 was used to test the algorithm. The results show that the SiamRPN++ algorithm using NewNet-62 as the main network has the great performance. Compared with the algorithms using ResNet-50, the accuracy of the SiamRPN++ algorithm increased to 0.524 and its EAO increased to 0.303. And its tracking speed increased to 73.74. The network complexity reduced to 3.013 billion and the network parameters reduced to 12.538 million, which significantly reduces network complexity and number of network parameters.
更多查看译文
关键词
Underwater single target tracking,SiamRPN++,inverted residual
AI 理解论文
溯源树
样例
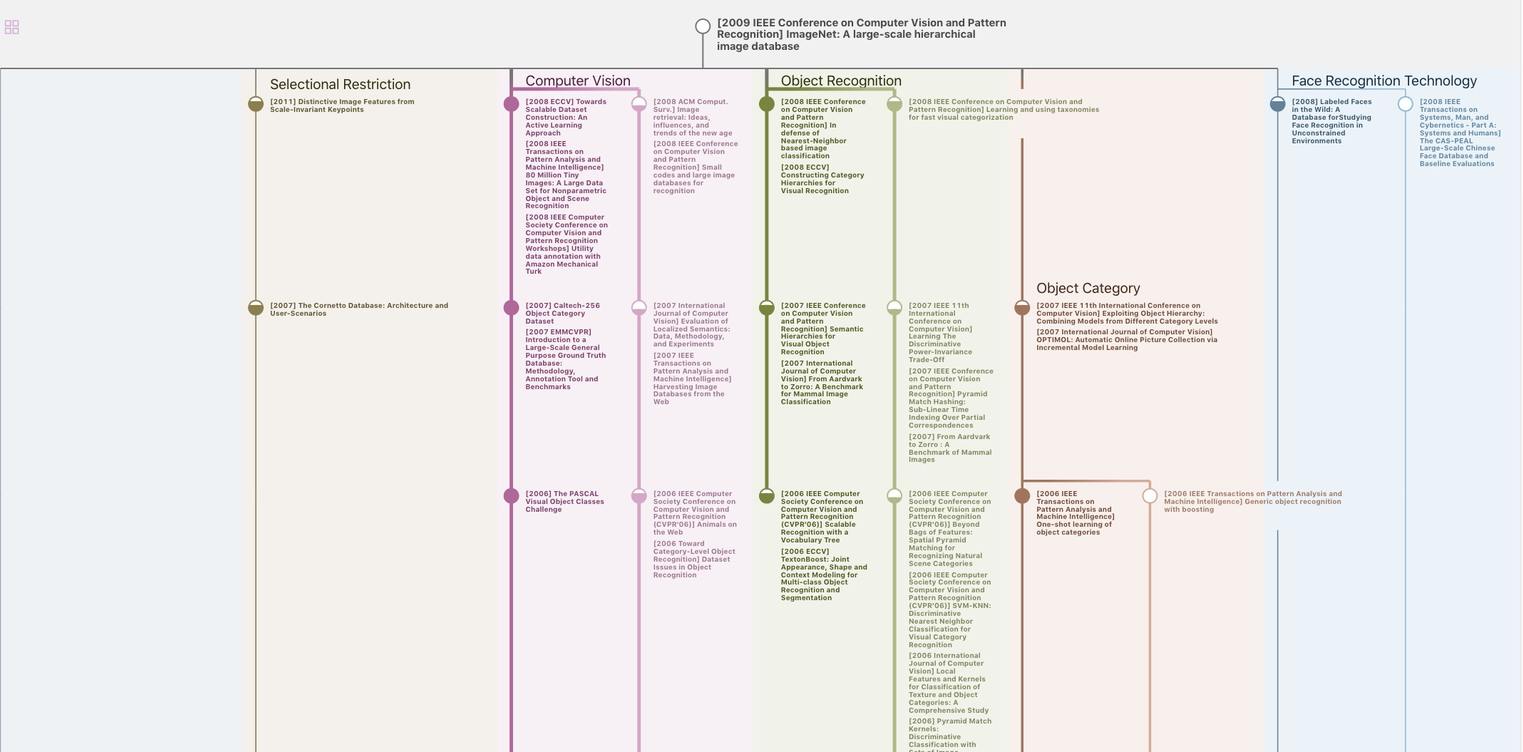
生成溯源树,研究论文发展脉络
Chat Paper
正在生成论文摘要