Improving the Reliability of ML-Corrected Climate Models With Novelty Detection
JOURNAL OF ADVANCES IN MODELING EARTH SYSTEMS(2023)
摘要
Using machine learning (ML) for the online correction of coarse-resolution atmospheric models has proven effective in reducing biases in near-surface temperature and precipitation rate. However, ML corrections often introduce new biases in the upper atmosphere and causes inconsistent model performance across different random seeds. Furthermore, they produce profiles that are outside the distribution of samples used in training, which can interfere with the baseline physics of the atmospheric model and reduce model reliability. This study introduces the use of a novelty detector to mask ML corrections when the atmospheric state is deemed out-of-sample. The novelty detector is trained on profiles of temperature and specific humidity in a semi-supervised fashion using samples from the coarsened reference fine-resolution simulation. The novelty detector responds to particularly biased simulations relative to the reference simulation by categorizing more columns as out-of-sample. Without novelty detection, corrective ML occasionally causes undesirably large climate biases. When coupled to a running year-long coarse-grid simulation, novelty detection deems about 21% of columns to be novelties. This identification reduces the spread in the root-mean-square error (RMSE) of time-mean spatial patterns of surface temperature and precipitation rate across a random seed ensemble. In particular, the random seed with the worst RMSE is improved by up to 60% (depending on the variable) while the best seed maintains its low RMSE. By reducing the variance in quality of ML-corrected climate models, novelty detection offers reliability without compromising prediction quality in atmospheric models.
更多查看译文
关键词
climate modeling,machine learning,global storm-resolving model
AI 理解论文
溯源树
样例
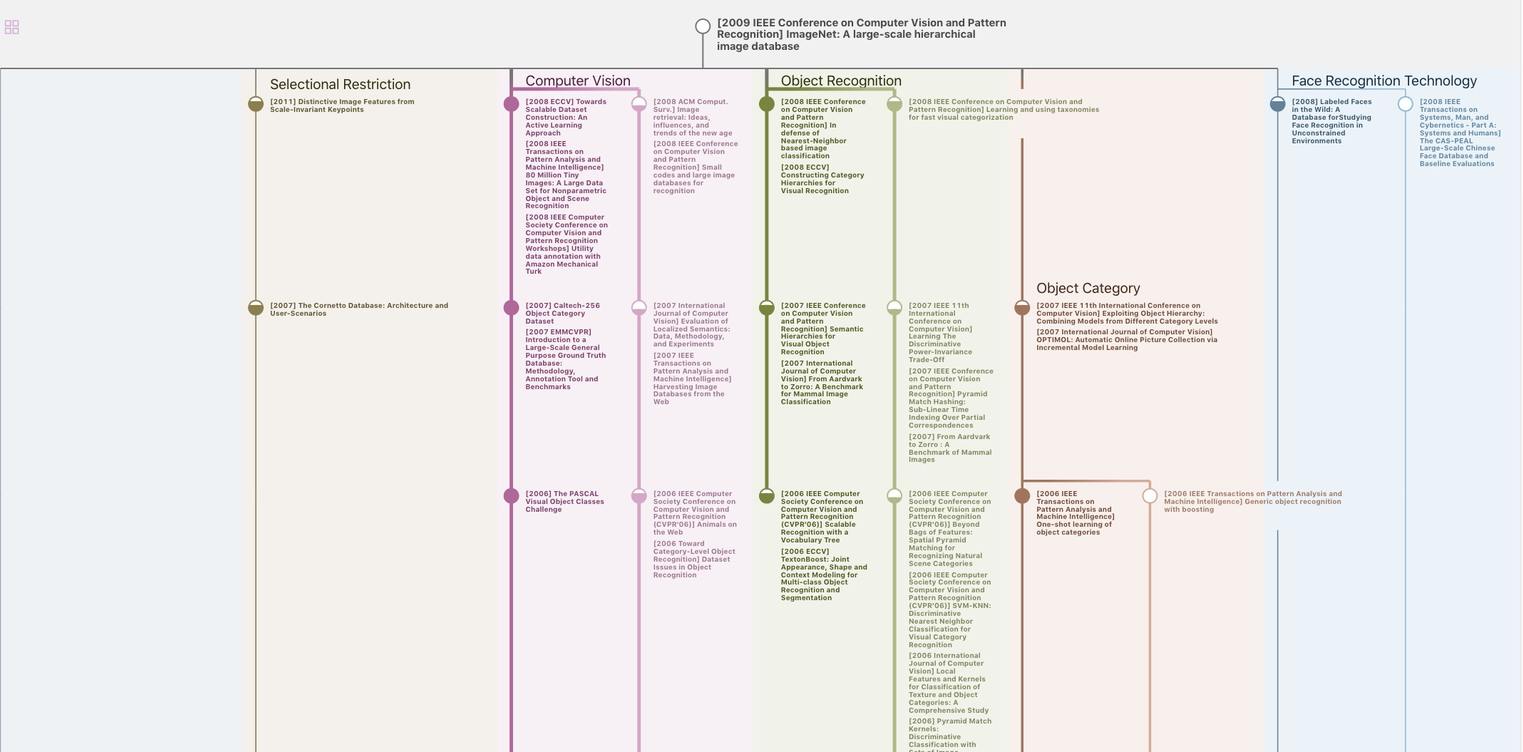
生成溯源树,研究论文发展脉络
Chat Paper
正在生成论文摘要