A Lithology Identification Approach Using Well Logs Data and Convolutional Long Short-Term Memory Networks
IEEE GEOSCIENCE AND REMOTE SENSING LETTERS(2023)
摘要
Lithology identification plays a crucial role in formation characterization and reservoir exploration. When available core samples are limited, well logs data becomes important in lithology identification. Various machine-learning algorithms have been adopted to identify lithology. However, because of the spatial coupling of logging data and the vertical spatial relationship of different depths, the lithology identification of subsurface reservoirs is a challenging task. To solve this challenge, we propose a lithology identification method based on a deep learning (DL) model, which combines a convolutional neural network (CNN) and a long short-term memory (LSTM) network to exert their complementary advantages. In the network mentioned above, the CNN is used to extract the complex features of the logging data, whereas the LSTM is designed to extract the vertical spatial relationship from the output features of the CNN, and finally, the mapping relationships between well logs and lithology types are established. The application results of two cases on field datasets demonstrate the effectiveness of the proposed method compared to the benchmark models. The proposed model is expected to be useful for identifying the lithology of complex strata.
更多查看译文
关键词
Data models,Reservoirs,Predictive models,Feature extraction,Data mining,Convolutional neural networks,Sediments,Convolutional neural network (CNN),deep learning (DL),lithology identification,long short-term memory (LSTM) network
AI 理解论文
溯源树
样例
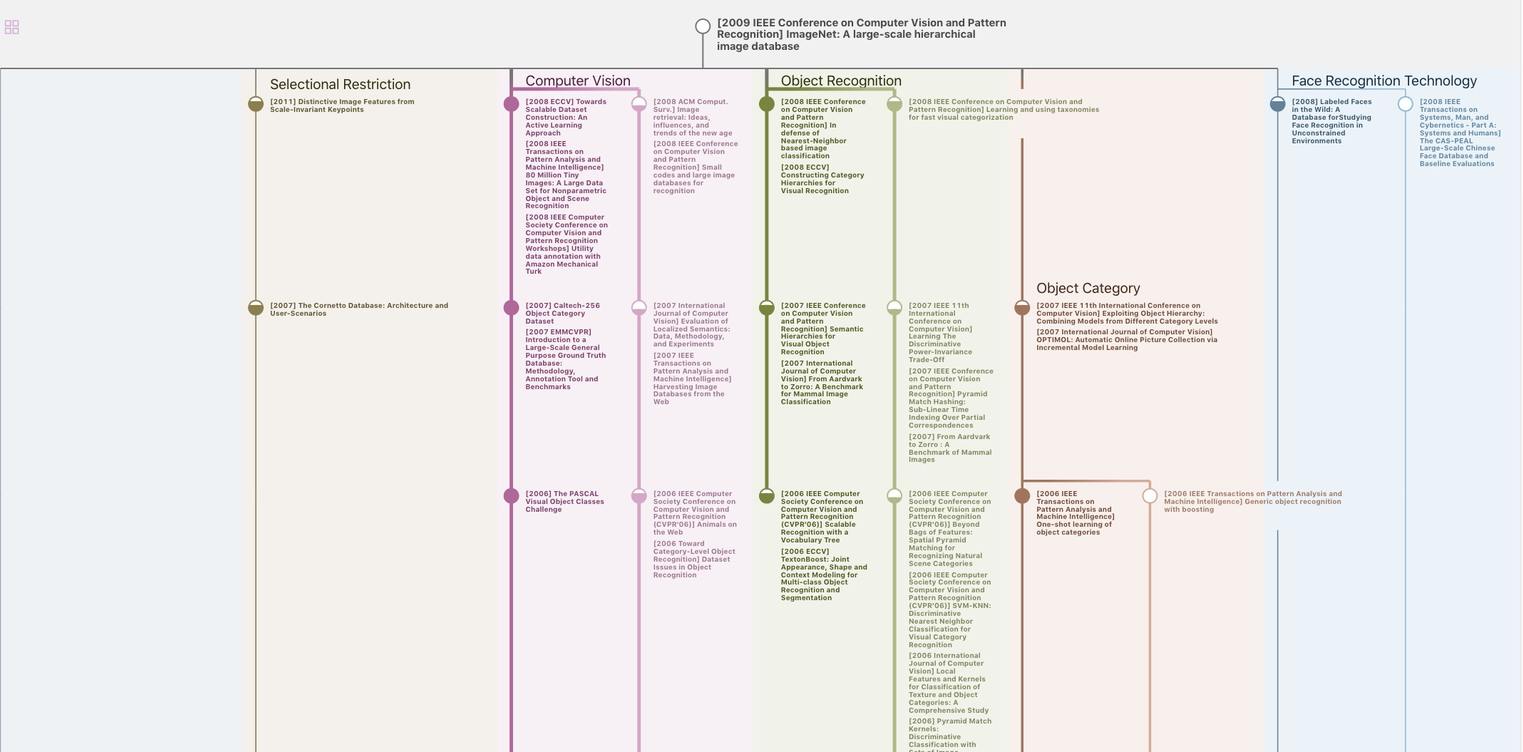
生成溯源树,研究论文发展脉络
Chat Paper
正在生成论文摘要